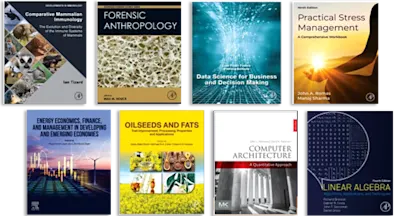
Tactile Sensing, Skill Learning, and Robotic Dexterous Manipulation
- 1st Edition - April 7, 2022
- Imprint: Academic Press
- Editors: Qiang Li, Shan Luo, Zhaopeng Chen, Chenguang Yang, Jianwei Zhang
- Language: English
- Paperback ISBN:9 7 8 - 0 - 3 2 3 - 9 0 4 4 5 - 2
- eBook ISBN:9 7 8 - 0 - 3 2 3 - 9 0 4 1 7 - 9
Tactile Sensing, Skill Learning and Robotic Dexterous Manipulation focuses on cross-disciplinary lines of research and groundbreaking research ideas in three research lines: ta… Read more
Purchase options
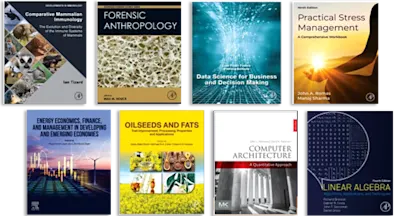
Tactile Sensing, Skill Learning and Robotic Dexterous Manipulation focuses on cross-disciplinary lines of research and groundbreaking research ideas in three research lines: tactile sensing, skill learning and dexterous control. The book introduces recent work about human dexterous skill representation and learning, along with discussions of tactile sensing and its applications on unknown objects’ property recognition and reconstruction. Sections also introduce the adaptive control schema and its learning by imitation and exploration. Other chapters describe the fundamental part of relevant research, paying attention to the connection among different fields and showing the state-of-the-art in related branches.
The book summarizes the different approaches and discusses the pros and cons of each. Chapters not only describe the research but also include basic knowledge that can help readers understand the proposed work, making it an excellent resource for researchers and professionals who work in the robotics industry, haptics and in machine learning.
- Provides a review of tactile perception and the latest advances in the use of robotic dexterous manipulation
- Presents the most detailed work on synthesizing intelligent tactile perception, skill learning and adaptive control
- Introduces recent work on human’s dexterous skill representation and learning and the adaptive control schema and its learning by imitation and exploration
- Reveals and illustrates how robots can improve dexterity by modern tactile sensing, interactive perception, learning and adaptive control approaches
Part I: Tactile sensing and perception
1. Tactile sensors for dexterous manipulation
2. Robotic perception of object properties using tactile sensing
3. Multimodal perception for dexterous manipulation
4. Using Machine Learning for Material Detection with Capacitive Proximity Sensors
Part II: Skill representation and learning
5. Admittance control: learning from human and collaboration with human
6. Sensorimotor Control for Dexterous Grasping--Inspiration from human hand
7. Efficient Haptic Learning and Interaction
8. From human to robot grasping: kinematics and forces synergies
9. Learning a form-closure grasping with attractive region in environment
10. Learning hierarchical control for robust in-hand manipulation
11. Learning Industrial Assembly by Guided-DDPG
Part III: Robotic hand adaptive control
12. The novel poly-articulated prosthetic hand Hannes: A survey study, and clinical evaluation
13. Enhancing vision control by tactile sensing for robotic manipulation
14. Neural Network enhanced Optimal Control of Manipulator
15. Towards Dexterous In-Hand Manipulation of Unknown Objects: A Feedback Based Control Approach
16. Learning Industrial Assembly by Guided-DDPG
- Edition: 1
- Published: April 7, 2022
- Imprint: Academic Press
- Language: English
QL
Qiang Li
SL
Shan Luo
ZC
Zhaopeng Chen
CY
Chenguang Yang
JZ