LIMITED OFFER
Save 50% on book bundles
Immediately download your ebook while waiting for your print delivery. No promo code needed.
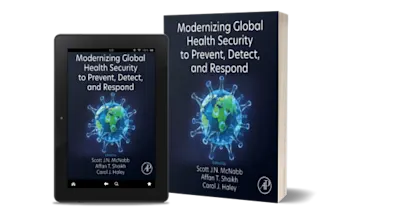
In an age where the amount of data collected from brain imaging is increasing constantly, it is of critical importance to analyse those data within an accepted framework to ensure… Read more
LIMITED OFFER
Immediately download your ebook while waiting for your print delivery. No promo code needed.
Acknowledgements
Part 1: Introduction
Chapter 1: A short history of SPM
Chapter 2: Statistical parametric mapping
Chapter 3: Modelling brain responses
Part 2: Computational anatomy
Chapter 4: Rigid Body Registration
Chapter 5: Non-linear Registration
Chapter 6: Segmentation
Chapter 7: Voxel-Based Morphometry
Part 3: General linear models
Chapter 8: The General Linear Model
Chapter 9: Contrasts and Classical Inference
Chapter 10: Covariance Components
Chapter 11: Hierarchical Models
Chapter 12: Random Effects Analysis
Chapter 13: Analysis of Variance
Chapter 14: Convolution Models for fMRI
Chapter 15: Efficient Experimental Design for fMRI
Chapter 16: Hierarchical models for EEG and MEG
Part 4: Classical inference
Chapter 17: Parametric procedures
Chapter 18: Random Field Theory
Chapter 19: Topological Inference
Chapter 20: False Discovery Rate procedures
Chapter 21: Non-parametric procedures
Part 5: Bayesian inference
Chapter 22: Empirical Bayes and hierarchical models
Chapter 23: Posterior probability maps
Chapter 24: Variational Bayes
Chapter 25: Spatio-temporal models for fMRI
Chapter 26: Spatio-temporal models for EEG
Part 6: Biophysical models
Chapter 27: Forward models for fMRI
Chapter 28: Forward models for EEG
Chapter 29: Bayesian inversion of EEG models
Chapter 30: Bayesian inversion for induced responses
Chapter 31: Neuronal models of ensemble dynamics
Chapter 32: Neuronal models of energetics
Chapter 33: Neuronal models of EEG and MEG
Chapter 34: Bayesian inversion of dynamic models
Chapter 35: Bayesian model selection and averaging
Part 7: Connectivity
Chapter 36: Functional integration
Chapter 37: Functional connectivity: eigenimages and multivariate analyses
Chapter 38: Effective Connectivity
Chapter 39: Non-linear coupling and kernels
Chapter 40: Multivariate autoregressive models
Chapter 41: Dynamic Causal Models for fMRI
Chapter 42: Dynamic causal models for EEG
Chapter 43: Dynamic Causal Models and Bayesian selection
Appendices
Linear models and inference
Dynamical systems
Expectation maximization
Variational Bayes under the Laplace approximation
Kalman filtering
Random field theory
Index
Color Plates
WP
KF
JA