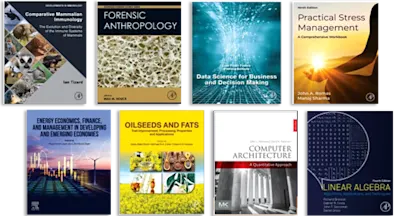
Mining Biomedical Text, Images and Visual Features for Information Retrieval
- 1st Edition - November 15, 2024
- Imprint: Academic Press
- Editors: Sujata Dash, Subhendu Kumar Pani, Wellington Pinheiro Dos Santos, Jake Y Chen
- Language: English
- Paperback ISBN:9 7 8 - 0 - 4 4 3 - 1 5 4 5 2 - 2
- eBook ISBN:9 7 8 - 0 - 4 4 3 - 1 5 4 5 1 - 5
Mining Biomedical Text, Images and Visual Features for Information Retrieval provides broad coverage of the concepts, themes, and instrumentalities of the important, evolvi… Read more
Purchase options
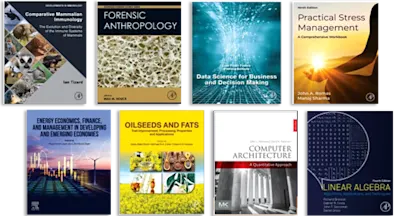
- Describes many biomedical imaging techniques to detect diseases at the cellular level i.e., image segmentation, classification, or image indexing using a variety of computational intelligence and image processing approaches
- Discusses how data mining techniques can be used for noise diminution and filtering MRI, EEG, MEG, fMRI, fNIRS, and PET Images
- Presents text mining techniques used for clinical documents in the areas of medicine and Biomedical NLP Systems
1. Introduction to IoT and Health Informatics
2. IoT system architectures in healthcare
3. Computational Intelligence in IoT Healthcare
4. Data Privacy in IoT E-health
5. IoT big data analytics in the healthcare industry.
6. Methodical IoT Based Information System in Healthcare Industry.
7. IoT for Smart Healthcare monitoring System
Part II: Computational Intelligence for Medical Image Processing
8. Computational Intelligence approaches in Biomedical image Processing
9. Distributed 3-D Medical Image Registration Using Intelligent Agents
10. Image Segmentation and Parameterization for Automatic Diagnostics
11. Computational Intelligence on Medical Imaging with Artificial Neural Networks
12. Evolutionary Computing and Its Use in Medical Imaging
13. Image Informatics for Clinical and Preclinical Biomedical Analysis
14. Topic Extractions (in Psychology)
15. Deep Learning in Medical Image Analysis
16. Automatic Segmentation of Multiple Organs on CT Images by Using Deep Learning Approaches
17. Medical Image Synthesis using Deep Learning
18. Medical Image Mining Using Data Mining Techniques
19. Biomedical Image Characterization and Radio genomics Using Machine Learning Techniques
Part III: Biomedical Natural Language Processing
20. Medical Ontology for text Categorization System
21. Biomedical terminologies resources for Information Retrieval
22. Image retrieval and Linguistic Indexing
23. Translation of Biomedical terms Using inferring rewriting rules
24. Lexical Analysis of Biomedical Ontologies
25. Word Sense Disambiguation in biomedical applications
26. Domain Specific Semantic Categories in Biomedical applications
- Edition: 1
- Published: November 15, 2024
- Imprint: Academic Press
- Language: English
SD
Sujata Dash
Sujata Dash holds the position of Professor at the Information Technology School of Engineering and Technology, Nagaland University, Dimapur Campus, Nagaland, India, bringing more than three decades of dedicated service in teaching and mentoring students. She has been honoured with the prestigious Titular Fellowship from the Association of Commonwealth Universities, United Kingdom. As a testament to her global contributions, she served as a visiting professor in the Computer Science Department at the University of Manitoba, Canada. With a prolific academic record, she has authored over 200 technical papers published in esteemed international journals, and conference proceedings, and edited book chapters by reputed publishers Serving as a reviewer and Associate Editor for approximately 15 international journals.
SK
Subhendu Kumar Pani
WD
Wellington Pinheiro Dos Santos
JY