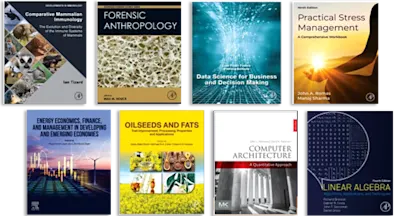
Computational Methods and Deep Learning for Ophthalmology
- 1st Edition - February 24, 2023
- Imprint: Academic Press
- Editor: D. Jude Hemanth
- Language: English
- Paperback ISBN:9 7 8 - 0 - 3 2 3 - 9 5 4 1 5 - 0
- eBook ISBN:9 7 8 - 0 - 3 2 3 - 9 5 4 1 4 - 3
Computational Methods and Deep Learning for Ophthalmology presents readers with the concepts and methods needed to design and use advanced computer-aided diagnosis systems f… Read more
Purchase options
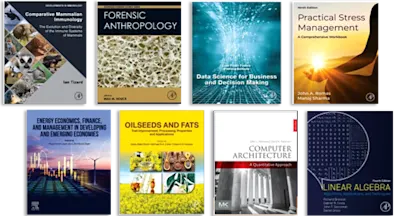
Computational Methods and Deep Learning for Ophthalmology presents readers with the concepts and methods needed to design and use advanced computer-aided diagnosis systems for ophthalmologic abnormalities in the human eye. Chapters cover computational approaches for diagnosis and assessment of a variety of ophthalmologic abnormalities. Computational approaches include topics such as Deep Convolutional Neural Networks, Generative Adversarial Networks, Auto Encoders, Recurrent Neural Networks, and modified/hybrid Artificial Neural Networks. Ophthalmological abnormalities covered include Glaucoma, Diabetic Retinopathy, Macular Degeneration, Retinal Vein Occlusions, eye lesions, cataracts, and optical nerve disorders.
This handbook provides biomedical engineers, computer scientists, and multidisciplinary researchers with a significant resource for addressing the increase in the prevalence of diseases such as Diabetic Retinopathy, Glaucoma, and Macular Degeneration.
- Presents the latest computational methods for designing and using Decision-Support Systems for ophthalmologic disorders in the human eye
- Conveys the role of a variety of computational methods and algorithms for efficient and effective diagnosis of ophthalmologic disorders, including Diabetic Retinopathy, Glaucoma, Macular Degeneration, Retinal Vein Occlusions, eye lesions, cataracts, and optical nerve disorders
- Explains how to develop and apply a variety of computational diagnosis systems and technologies, including medical image processing algorithms, bioinspired optimization, Deep Learning, computational intelligence systems, fuzzy-based segmentation methods, transfer learning approaches, and hybrid Artificial Neural Networks
Researchers, developers, and industry professionals in Machine Learning, Deep Learning, Computational Intelligence, Medical Image Analysis, and Medical Decision Support Systems, as well as researchers and industry professionals in biomedical imaging, and human-machine interaction. In addition, clinicians and ophthalmologists who are involved in rese
1. Classification of ocular diseases using transfer learning approaches and glaucoma severity grading
D. Selvathi
2. Early diagnosis of diabetic retinopathy using deep learning techniques
Bam Bahadur Sinha, R. Dhanalakshmi and K. Balakrishnan
3. Comparison of deep CNNs in the identification of DME structural changes in retinal OCT scans
N. Padmasini, R. Umamaheswari, Mohamed Yacin Sikkandar and Manavi D. Sindal
4. Epidemiological surveillance of blindness using deep learning approaches
Kurubaran Ganasegeran and Mohd Kamarulariffin Kamarudin
5. Transfer learning-based detection of retina damage from optical coherence tomography images
Bam Bahadur Sinha, Alongbar Wary, R. Dhanalakshmi and K. Balakrishnan
6. An improved approach for classification of glaucoma stages from color fundus images using Efficientnet-b0 convolutional neural network and recurrent neural network
Poonguzhali Elangovan, D. Vijayalakshmi and Malaya Kumar Nath
7. Diagnosis of ophthalmic retinoblastoma tumors using 2.75D CNN segmentation technique
T. Jemima Jebaseeli and D. Jasmine David
8. Fast bilateral filter with unsharp masking for the preprocessing of optical coherence tomography images - an aid for segmentation and classification
Ranjitha Rajan and S.N. Kumar
9. Deep learning approaches for the retinal vasculature segmentation in fundus images
V. Sathananthavathi and G. Indumathi
10. Grading of diabetic retinopathy using deep learning techniques
Asha Gnana Priya H, Anitha J and Ebenezer Daniel
11. Segmentation of blood vessels and identification of lesion in fundus image by using fractional derivative in fuzzy domain
V.P. Ananthi and G. Santhiya
12. U-net autoencoder architectures for retinal blood vessels segmentation
S. Deivalakshmi, R. Adarsh, J. Sudaroli Sandana and Gadipudi Amarnageswarao
13. Detection and diagnosis of diseases by feature extraction and analysis on fundus images using deep learning techniques
Ajantha Devi Vairamani
- Edition: 1
- Published: February 24, 2023
- Imprint: Academic Press
- Language: English
DH