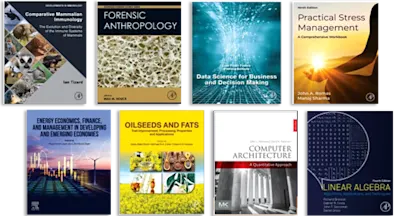
Wise Use of Null Hypothesis Tests
A Practitioner's Handbook
- 1st Edition - October 14, 2022
- Imprint: Academic Press
- Author: Frank S Corotto
- Language: English
- Paperback ISBN:9 7 8 - 0 - 3 2 3 - 9 5 2 8 4 - 2
- eBook ISBN:9 7 8 - 0 - 3 2 3 - 9 5 2 8 5 - 9
Few students sitting in their introductory statistics class learn that they are being taught the product of a misguided effort to combine two methods into one. Few students le… Read more
Purchase options
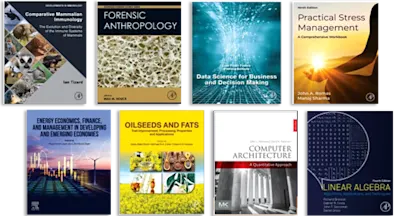
Few students sitting in their introductory statistics class learn that they are being taught the product of a misguided effort to combine two methods into one. Few students learn that some think the method they are being taught should be banned. Wise Use of Null Hypothesis Tests: A Practitioner’s Handbook follows one of the two methods that were combined: the approach championed by Ronald Fisher. Fisher’s method is simple, intuitive, and immune to criticism.
Wise Use of Null Hypothesis Tests
is also a user-friendly handbook meant for practitioners. Rather than overwhelming the reader with endless mathematical operations that are rarely performed by hand, the author of Wise Use of Null Hypothesis Tests emphasizes concepts and reasoning. In Wise Use of Null Hypothesis Tests, the author explains what is accomplished by testing null hypotheses—and what is not. The author explains the misconceptions that concern null hypothesis testing. He explains why confidence intervals show the results of null hypothesis tests, performed backwards. Most importantly, the author explains the Big Secret. Many—some say all—null hypotheses must be false. But authorities tell us we should test false null hypotheses anyway to determine the direction of a difference that we know must be there (a topic unrelated to so-called one-tailed tests). In Wise Use of Null Hypothesis Tests, the author explains how to control how often we get the direction wrong (it is not half of alpha) and commit a Type III (or Type S) error.- Offers a user-friendly book, meant for the practitioner, not a comprehensive statistics book
- Based on the primary literature, not other books
- Emphasizes the importance of testing null hypotheses to decide upon direction, a topic unrelated to so-called one-tailed tests
- Covers all the concepts behind null hypothesis testing as it is conventionally understood, while emphasizing a superior method
- Covers everything the author spent 32 years explaining to others: the debate over correcting for multiple comparisons, the need for factorial analysis, the advantages and dangers of repeated measures, and more
- Explains that, if we test for direction, we are practicing an unappreciated and unnamed method of inference
Chapter 1. The conventional method is a flawed fusion
1.1 Three statisticians, two methods, and the mess that should be banned
1.2 Wise use and testing nulls that must be false
1.3 Null hypothesis testing in perspective
Chapter 2. The point is to generalize beyond our results
2.1 Samples and populations
2.2 Real and hypothetical populations
2.3 Randomization
2.4 Know your population, and do not generalize beyond it
Chapter 3. Null hypothesis testing explained
3.1 The effect of sampling error
3.2 The logic of testing a null hypothesis
3.3 We should know from the start that many null hypotheses cannot be correct
3.4 The traditional explanation of how to use p
3.5 What use of α accomplishes
3.6 The flawed hybrid in action
3.7 Criticisms of the flawed hybrid
3.8 We should test nulls in a way that answers the criticisms
3.9 How to use p and α
3.10 Mouse preference, done right this time
3.11 More p-values in action
3.12 What were the nulls and predictions?
3.13 What if p50.05000?
3.14 A radical but wise way to use p
3.15 0.05 or .05? p or P?
Chapter 4. How often do we get it wrong?
4.1 Distributions around means4.2 Distributions of test statistics
4.3 Null hypothesis testing explained with distributions
4.4 Type I errors explained
4.5 Probabilities before and after collecting data
4.6 The null’s precision explained
4.7 The awkward definition of p explained
4.8 Errors in direction
4.9 Power and errors in direction
4.10 Manipulating power to lower p-values
4.11 Increasing power with one-tailed tests
4.12 Power and why we should we set α to 0.10 or higher
4.13 Power, estimated effect size, and type M errors
4.14 How can we know a population’s distribution?
Chapter 5. Important things to know about null hypothesis testing
5.1 Examples of null hypotheses in proper statistics books and what they really mean
5.2 Categories of null hypotheses?
5.3 What if is important to accept the null?
5.4 Never do this
5.5 Null hypothesis testing as never explained before
5.6 Effect size: what is it and when is it important?
5.7 We should provide all results, even those not statistically “significant”
Chapter 6. Common misconceptions
6.1 Null hypothesis testing is misunderstood by many
6.2 Statistical “significance” means a difference is large enough to be important—wrong!
6.3 p is the probability of a type I error—wrong!
6.4 If results are statistically “significant,” we should accept the alternative hypothesis that something other than the null is correct—wrong!
6.5 If results are not statistically “significant,” we should accept the null hypothesis—wrong!
6.6 Based on p we should either reject or fail to reject the null hypothesis—often wrong!
6.7 Null hypothesis testing is so flawed that we should use confidence intervals instead—wrong!
6.8 Power can be used to justify accepting the null hypothesis—wrong!
6.9 The null hypothesis is a statement of no difference—not always
6.10 The null hypothesis is that there will be no significant difference between the expected and observed values—very, very wrong!
6.11 A null hypothesis should not be a negative statement—wrong!
Chapter 7. The debate over null hypothesis testing and wise use as the solution
7.1 The debate over null hypothesis testing
7.2 Communicate to educate
7.3 Plan ahead
7.4 Test nulls when appropriate, not promiscuously
7.5 Strike the right balance between what is conventional and what is best
7.6 Think outside of the null hypothesis test
7.7 Encourage our audience to draw their own conclusions
7.8 Allow ourselves to draw our own conclusions
7.9 Strike the right balance when providing our results
7.10 Know the misconceptions and do not fall for them
7.11 Do not say that two groups “differ” or “do not differ”
7.12 Provide all results somehow
7.13 Other reformed methods of null hypothesis testing
Chapter 8. Simple principles behind the mathematics and some essential concepts
8.1 Why different types of data require different types of tests
8.1.1 Simple principles behind the mathematics
8.1.2 Numerical data exhibit variation
8.1.3 Nominal data do not exhibit variation
8.1.4 How to tell the difference between nominal and numerical data
8.2 Simple principles behind the analysis of groups of measurements and discrete numerical data
8.2.1 Variance: a statistic of huge importance
8.2.2 Incorporating sample size and the difference between our prediction and our outcome
8.3 Drawing conclusions when we knew all along that the null must be false
8.4 Degrees of freedom explained
8.5 Other types of t tests
8.6 Analysis of variance and t tests have certain requirements
8.7 Do not test for equal variances unless . . .
8.8 Simple principles behind the analysis of counts of observations within categories
8.8.1 Counts of observations within categories
8.8.2 When the null hypothesis specifies the prediction
8.8.3 When there is only one degree of freedom
8.8.4 When the null hypothesis does not specify the prediction
8.9 Interpreting p when the null hypothesis cannot be correct
8.10 232 Designs and other variations
8.11 The problem with chi-squared tests
8.12 The reasoning behind the mathematics
8.13 Rules for chi-squared tests
Chapter 9. The two-sample t test and the importance of pooled variance
Chapter 10. Comparing more than two groups to each other
10.1 If we have three or more samples, most say we cannot use two-sample t tests to compare them two samples at a time
10.2 Analysis of variance
10.3 The price we pay is power
10.4 Comparing every group to every other group
10.5 Comparing multiple groups to a single reference, like a control
10.6 Is all of this a load of rubbish?
Chapter 11. Assessing the combined effects of multiple independent variables
11.1 Independent variables alone and in combination
11.2 No, we may not use multiple t tests
11.3 We have a statistical main effect: now what?
11.4 We have a statistical interaction: things to consider
11.5 We have a statistical interaction and we want to keep testing nulls
11.6 Which is more important, the main effect or the interaction?
11.7 Designs with more than two independent variables
11.8 Use of analysis of variance to reduce variation and increase power
Chapter 12. Comparing slopes: analysis of covariance
12.1 Analysis of covariance
12.2 Use of analysis of covariance to reduce variation and increase power
12.3 More on the use of analysis of covariance to reduce variation and increase power
12.4 Use of analysis of covariance to limit the effects of a confound
Chapter 13. When data do not meet the requirements of t tests and analysis of variance
13.1 When do we need to take action?
13.2 Floor effects and the square root transformation
13.3 Floor and ceiling effects and the arcsine transformation
13.4 Not as simple as a floor or ceiling effect—the rank transformation
13.5 Making analysis of variance sensitive to differences in proportion—the logarithmic transformation
13.6 Nonparametric tests
13.7 Transforming data changes the question being asked
Chapter 14. Reducing variation and increasing power by comparing subjects to themselves
14.1 The simple principle behind the mathematics
14.2 Repeated measures analysis of variances
14.3 Multiple comparisons tests on repeated measures
14.4 When subjects are not organisms
14.5 When repeated does not mean repeated over time
14.6 Pretest-posttest designs illustrate the danger of measures repeated over time
14.7 Repeated measures analysis of variance versus t tests
14.8 The problem with repeated measures
14.8.1 The requirement for sphericity
14.8.2 Correcting for a lack of sphericity
14.8.3 Multiple comparisons tests when there is a lack of sphericity
14.8.4 The multivariate alternative to correction
Chapter 15. What do those error bars mean?
15.1 Confidence intervals
15.2 Testing null hypotheses in our heads
15.3 Plotting confidence intervals
15.4 Error bars and repeated measures
15.5 Plot comparative confidence intervals to make the overlap myth a reality
Bonus chapters:
Appendix A: Philosophical objections
A.1 Decades of bitter debate
A.2 We want to know when we are wrong, not how often
A.3 Setting α to 0.05 does not mean that 5% of all null-based decisions are wrong 158
A.4 There are better ways to analyze and interpret data
A.5 The fallacy of affirming the consequent
A.6 Some say our method cannot be used to determine direction
A.6.1. The return of one-tailed tests
A.6.2. Kaiser’s absurd directional two-tailed tests
A.6.3. Invoking power to justify Kaiser’s directional two-tailed tests
A.6.4. Fisher did not follow Kaiser’s rules
A.6.5. Still not convinced?
Appendix B: How Fisher used null hypothesis tests
B.1 Why follow my advice?
B.2 Fisher tested for direction
B.3 Others did too
B.4 Fisher believed α should vary according to the circumstances
B.5 Fisher came close to saying there should be no α at all
B.6 In practice, Fisher did not categorize outcomes
B.7 Fisher’s language answers many criticisms of null hypothesis testing
B.8 Except for Fisher’s use of “significant”
B.9 Fisher’s inconsistency explained
B.10 Fisher’s thinking expressed in one word
B.11 We have come a long way since Fisher, but the wrong way?
Appendix C: The method attributed to Neyman and Pearson
C.1 Neyman and Pearson with Pearson
C.2 Neyman and Pearson without Pearson
C.3 An important limitation
C.4 Alternatives are always infinitely numerically precise
C.5 The method step-by-step
C.6 The method’s influence on the flawed hybrid
C.7 The method’s fate in the world of the flawed hybrid
C.8 Power spreads its wings
C.9 Neyman et al.’s method has no place in science
- Edition: 1
- Published: October 14, 2022
- Imprint: Academic Press
- Language: English
FC