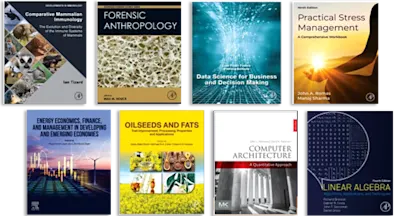
Unlocking the Secrets of Soil
Applying AI and Sensor Technologies for Sustainable Land Use
- 1st Edition - June 6, 2025
- Imprint: Elsevier
- Editors: David C. Weindorf, Somsubhra Chakraborty, Bin Li
- Language: English
- Paperback ISBN:9 7 8 - 0 - 4 4 3 - 2 9 8 7 9 - 0
- eBook ISBN:9 7 8 - 0 - 4 4 3 - 2 9 8 8 0 - 6
Unlocking the Secrets of the Soil: Applying AI and Sensor Technologies for Sustainable Land Use is a comprehensive guide to the latest advances in soil characterization. This b… Read more
Purchase options
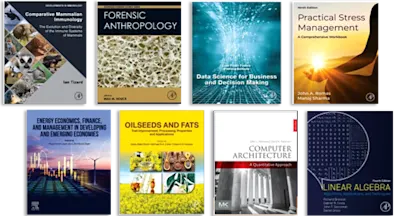
- Provides an integration of AI and Sensor technologies
- Highlights the importance of sustainable land use and the role that modern technologies can play in achieving this goal
- Presents an interdisciplinary approach, drawing on expertise from various fields such as agriculture, environmental science, and computer science
• Definition of soil characterization
• Overview of the importance of sensor-based soil characterization
• Brief history of soil characterization techniques
• Objectives of the book
2: Fundamentals of Soil Characterization
• Soil properties and their importance
• Types of soil characterization techniques
• Advantages and limitations of different techniques
• Soil sampling and preparation
3: Sensor-based Soil Characterization Techniques
• Introduction to sensor-based techniques
• Types of sensors for soil characterization
• Applications of different sensors in soil characterization
• Smart soil sensing: hardware and software components
• Advantages and limitations of sensor-based techniques
4: Machine Learning and AI Techniques
• Introduction to machine learning and AI
• Types of machine learning and AI techniques
• Data preparation for machine learning and AI
• Data processing techniques • Model selection and validation
• Data visualization and interpretation
5: Applications of Machine Learning and AI in Soil Science
• Soil classification and mapping
• Soil quality assessment
• Soil fertility prediction
• Soil erosion prediction
• Crop yield prediction
• Big data analytics for soil
6: Image Acquisition and Processing Techniques
• Introduction to image acquisition and processing
• Types of image acquisition and processing techniques
• Image enhancement and segmentation
• Feature extraction and selection
7: Applications of Machine Learning and AI in Image-Based Soil characterization
• Soil texture classification
• Soil structure analysis
• Soil organic matter content estimation
• Soil moisture prediction
• Soil compaction detection
• Digital soil morphometrics
8: Applications of Sensor + AI based Soil Characterization
• Precision agriculture
• Environmental monitoring
• Soil quality assessment
• Wireless sensor networks (WSNs) for soil sensing
• IoT platforms for data management and analysis
• Decision-making in agriculture and land management
• Challenges and future directions
9: Case Studies
• Case studies on the application of sensor-based soil characterization in agriculture, forestry, and environmental monitoring using machine learning and AI
10: Conclusion and Future Directions
• Summary of the key points
• Future directions for sensor+AI-based soil characterization
• Challenges and opportunities for further research and development.
- Edition: 1
- Published: June 6, 2025
- Imprint: Elsevier
- Language: English
DW
David C. Weindorf
Dr. Weindorf’s research interests are at the nexus of soil science and environmental quality assessment. He is an internationally recognized authority in proximal sensor characterization of soils with extensive work in hydrocarbon and heavy metal polluted soils. Over a 20+ year career, he has worked in 30 countries worldwide and serves on two Elsevier editorial boards (Pedosphere and Geoderma).
He has published >200 peer reviewed research papers with >6000 citations of his work. He is Fulbright Scholar, a Fulbright Specialist, and Fellow & Presidential Award winner in the Soil Science Society of America. He has offered invited testimony before the US Congress and regularly provides expert testimony in legal matters germane to environmental quality assessment.
SC
Somsubhra Chakraborty
Dr. Chakraborty's research interests lie in proximal soil sensors in combination with data mining and machine learning, with a focus on developing scalable algorithms for rapidly and non-invasively predicting soil properties. In particular, he has worked on developing methods for sensors like portable XRF, diffuse reflectance spectroscopy, smartphone-based soil sensing, Nix color sensor, digital soil mapping, as well as techniques for dealing with real-time soil characterization.
He has published over 100 research articles in various international journals and conferences, and his work has been cited over 3,700 times. He has received several awards and honors for his contributions to the field of soil science, including the Australia awards fellowship and SPESS garden scholarship (USA). In addition to his research, Chakraborty is also actively involved in teaching and mentoring students at IIT Kharagpur. He has supervised several Ph.D. and M. Tech. students and has also been involved in the development of several online courses on soil science.
BL