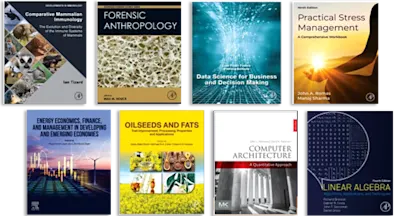
Uncertainty in Data Envelopment Analysis
Fuzzy and Belief Degree-Based Uncertainties
- 1st Edition - May 24, 2023
- Imprint: Academic Press
- Authors: Farhad Hosseinzadeh Lotfi, Masoud Sanei, Ali Asghar Hosseinzadeh, Sadegh Niroomand, Ali Mahmoodirad
- Language: English
- Paperback ISBN:9 7 8 - 0 - 3 2 3 - 9 9 4 4 4 - 6
- eBook ISBN:9 7 8 - 0 - 3 2 3 - 9 9 4 4 5 - 3
Classical data envelopment analysis (DEA) models use crisp data to measure the inputs and outputs of a given system. In cases such as manufacturing systems, production processes,… Read more
Purchase options
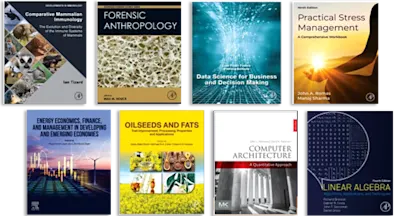
Classical data envelopment analysis (DEA) models use crisp data to measure the inputs and outputs of a given system. In cases such as manufacturing systems, production processes, service systems, etc., the inputs and outputs may be complex and difficult to measure with classical DEA models. Crisp input and output data are fundamentally indispensable in the conventional DEA models. If these models contain complex uncertain data, then they will become more important and practical for decision makers.
Uncertainty in Data Envelopment Analysis introduces methods to investigate uncertain data in DEA models, providing a deeper look into two types of uncertain DEA methods, fuzzy DEA and belief degree-based uncertainty DEA, which are based on uncertain measures. These models aim to solve problems encountered by classical data analysis in cases where the inputs and outputs of systems and processes are volatile and complex, making measurement difficult.
Uncertainty in Data Envelopment Analysis introduces methods to investigate uncertain data in DEA models, providing a deeper look into two types of uncertain DEA methods, fuzzy DEA and belief degree-based uncertainty DEA, which are based on uncertain measures. These models aim to solve problems encountered by classical data analysis in cases where the inputs and outputs of systems and processes are volatile and complex, making measurement difficult.
- Introduces methods to deal with uncertain data in DEA models, as a source of information and a reference book for researchers and engineers
- Presents DEA models that can be used for evaluating the outputs of many reallife systems in social and engineering subjects
- Provides fresh DEA models for efficiency evaluation from the perspective of imprecise data
- Applies the fuzzy set and uncertainty theories to DEA to produce a new method of dealing with the empirical data
Graduate students, researchers, and professional engineers who study or perform optimization and evaluation, in the fields of applied mathematics, industrial engineering, computer science, information science, management science, economics, and operations research
1: Uncertain Theories
1.1 Introduction
1.1.1 Importance of the subjects of the book.
1.1.2 Motivation
1.1.3 The structure of the book
1.2 Fuzzy set theory
1.2.1 Sone basic definitions of fuzzy sets
1.2.2 Types of fuzzy sets
1.2.3 Fuzzy numbers
1.2.4 Fuzzy measures
1.2.5 Extension principle
1.2.6 Ranking function
1.2.7 Possibility theory
1.3 Belief degree based uncertain theor
1.3.1 Uncertain measur
1.3.2 Uncertain Variable
1.3.3 Operation law
1.3.4 Expected value
2: Introduction to Data Envelopment Analysis
2.1 Basic DEA Models
2.1.1 The CCR Model
2.1.2 The BCC Model
2.1.3 A Slacks-Based Measure Model
2.1.4 RUSSEL Model
2.1.5 Additive Model
2.2 Ranking DMUs in DEA
3: Fuzzy Data Envelopment Analysis
3.1 Fuzzy DEA Models
3.1.1 Fuzzy CCR Model
3.1.2 Fuzzy BCC Model
3.1.3 Fuzzy A Slacks-Based Measure Model
3.1.4 Fuzzy RUSSEL Model
3.1.5 Fuzzy Additive Model
4: Ranking and Sensitivity and Stability in Fuzzy DEA
4.1 The Ranking Models DMUs in fuzzy DEA
4.1.1 The fuzzy Ranking Models by L-1 Norm
4.1.2 The fuzzy Ranking Models by infinity Norm
4.1.3 Other ranking models in fuzzy DEA
4.1.4 The fuzzy cross-efficiency Model
4.2 Sensitivity and Stability
5: Uncertain Data Envelopment Analysis
5.1 Belief degree based uncertain CCR model
5.2 Belief degree based uncertain BCC model
5.3 Belief degree based uncertain SBM model
5.4 Belief degree based uncertain RUSSEL model
5.5 Belief degree based uncertain Additive models
6: Ranking and Sensitivity and Stability in Uncertain DEA
6.1 Uncertain DEA Ranking Criteria
6.1.1 Expected Ranking Criteria
6.1.2 Optimistic Ranking Criteria
6.1.3 Maximal Chance Ranking Criteria
6.1.4 Hurwicz Ranking Criteria
6.2 Sensitivity and Stability
1.1 Introduction
1.1.1 Importance of the subjects of the book.
1.1.2 Motivation
1.1.3 The structure of the book
1.2 Fuzzy set theory
1.2.1 Sone basic definitions of fuzzy sets
1.2.2 Types of fuzzy sets
1.2.3 Fuzzy numbers
1.2.4 Fuzzy measures
1.2.5 Extension principle
1.2.6 Ranking function
1.2.7 Possibility theory
1.3 Belief degree based uncertain theor
1.3.1 Uncertain measur
1.3.2 Uncertain Variable
1.3.3 Operation law
1.3.4 Expected value
2: Introduction to Data Envelopment Analysis
2.1 Basic DEA Models
2.1.1 The CCR Model
2.1.2 The BCC Model
2.1.3 A Slacks-Based Measure Model
2.1.4 RUSSEL Model
2.1.5 Additive Model
2.2 Ranking DMUs in DEA
3: Fuzzy Data Envelopment Analysis
3.1 Fuzzy DEA Models
3.1.1 Fuzzy CCR Model
3.1.2 Fuzzy BCC Model
3.1.3 Fuzzy A Slacks-Based Measure Model
3.1.4 Fuzzy RUSSEL Model
3.1.5 Fuzzy Additive Model
4: Ranking and Sensitivity and Stability in Fuzzy DEA
4.1 The Ranking Models DMUs in fuzzy DEA
4.1.1 The fuzzy Ranking Models by L-1 Norm
4.1.2 The fuzzy Ranking Models by infinity Norm
4.1.3 Other ranking models in fuzzy DEA
4.1.4 The fuzzy cross-efficiency Model
4.2 Sensitivity and Stability
5: Uncertain Data Envelopment Analysis
5.1 Belief degree based uncertain CCR model
5.2 Belief degree based uncertain BCC model
5.3 Belief degree based uncertain SBM model
5.4 Belief degree based uncertain RUSSEL model
5.5 Belief degree based uncertain Additive models
6: Ranking and Sensitivity and Stability in Uncertain DEA
6.1 Uncertain DEA Ranking Criteria
6.1.1 Expected Ranking Criteria
6.1.2 Optimistic Ranking Criteria
6.1.3 Maximal Chance Ranking Criteria
6.1.4 Hurwicz Ranking Criteria
6.2 Sensitivity and Stability
- Edition: 1
- Published: May 24, 2023
- Imprint: Academic Press
- Language: English
FL
Farhad Hosseinzadeh Lotfi
Dr. Lotfi is a Full Professor of Mathematics at the Science and Research Branch, Islamic Azad University (IAU), Tehran, Iran. In 1992, he received his undergraduate degree in Mathematics at Yazd University, Yazd, Iran. He received his M.Sc in Operations Research at IAU, Lahijan, Iran in 1996 and PhD in Applied Mathematics (O.R.) at IAU, Science and Research Branch, Tehran, Iran in 2000. His major research interests are operations research and data envelopment analysis. He has published more than 300 scientific and technical papers in leading scientific journals, including European Journal of Operational Research, Computers and Industrial Engineering, Journal of the Operational Research Society, Applied Mathematics and Computation, Applied Mathematical Modelling, Mathematical and Computer Modelling, and Journal of the Operational Research Society of Japan, etc. He is Editor-in-Chief and member of editorial board of Journal of Data Envelopment Analysis and Decision Science. He is also Director-in-Charge and member of editorial board of International Journal of Industrial Mathematics.
Affiliations and expertise
Science and Research Branch, Islamic Azad University, Tehran, IranMS
Masoud Sanei
Masoud Sanei is an Associate Professor at the Department of Applied Mathematics, Islamic Azad University, Central Tehran Branch, in Iran. His research interests are in the areas of operation research such as Data Envelopment Analysis, Uncertainty Theory, and Supply Chain Management. He has several papers in journals and conference proceedings.
Affiliations and expertise
Associate Professor of Mathematics, Central Tehran Branch, Islamic Azad University, Tehran, IranAH
Ali Asghar Hosseinzadeh
Ali Asghar Hosseinzadeh is an Assistant Professor of Applied Mathematics in the Lahijan branch of Islamic Azad University, in Iran. His research interests include Fuzzy Mathematical Programming, Data Envelopment Analysis, and Uncertainty Theory. He has published research articles in international journals of Mathematics and Industrial Engineering.
Affiliations and expertise
Assistant Professor of Mathematics, Lahijan Branch, Islamic Azad University, Lahijan, IranSN
Sadegh Niroomand
Sadegh Niroomand is an Associate Professor of Industrial Engineering in Firouzabad Institute of Higher Education, in Iran. He received his PhD degree in Industrial Engineering from Eastern Mediterranean University. His research interests are Operations Research, Fuzzy Theory, Exact and Meta-heuristic Solution Approaches.
Affiliations and expertise
Associate Professor of Industrial Engineering, Firouzabad Institute of Higher Education, Firouzabad, Fars, IranAM
Ali Mahmoodirad
Ali Mahmoodirad is an Associate Professor of Applied Mathematics in Masjed-Sleiman branch of Islamic Azad University in Iran. His research interests include Fuzzy Mathematical Programming, Supply Chain Management, and Uncertainty Theory. He has published research articles in international journals of Mathematics and industrial engineering.
Affiliations and expertise
Associate Professor of Applied Mathematics, Masjed-Soleiman Branch Islamic Azad University, Masjed-Soleiman, IranRead Uncertainty in Data Envelopment Analysis on ScienceDirect