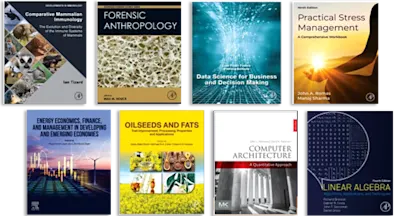
Uncertainty in Artificial Intelligence 4
- 1st Edition, Volume 9 - October 3, 2014
- Imprint: North Holland
- Editors: T.S. Levitt, L.N. Kanal, J.F. Lemmer, R.D. Shachter
- Language: English
- Paperback ISBN:9 7 8 - 0 - 4 4 4 - 8 8 7 3 7 - 5
- eBook ISBN:9 7 8 - 1 - 4 8 3 2 - 9 6 5 4 - 8
Clearly illustrated in this volume is the current relationship between Uncertainty and AI.It has been said that research in AI revolves around five basic questions asked relativ… Read more
Purchase options
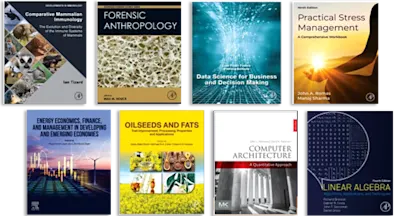
Clearly illustrated in this volume is the current relationship between Uncertainty and AI.
It has been said that research in AI revolves around five basic questions asked relative to some particular domain: What knowledge is required? How can this knowledge be acquired? How can it be represented in a system? How should this knowledge be manipulated in order to provide intelligent behavior? How can the behavior be explained? In this volume, all of these questions are addressed. From the perspective of the relationship of uncertainty to the basic questions of AI, the book divides naturally into four sections which highlight both the strengths and weaknesses of the current state of the relationship between Uncertainty and AI.
It has been said that research in AI revolves around five basic questions asked relative to some particular domain: What knowledge is required? How can this knowledge be acquired? How can it be represented in a system? How should this knowledge be manipulated in order to provide intelligent behavior? How can the behavior be explained? In this volume, all of these questions are addressed. From the perspective of the relationship of uncertainty to the basic questions of AI, the book divides naturally into four sections which highlight both the strengths and weaknesses of the current state of the relationship between Uncertainty and AI.
I. Causal Models. On the Logic of Causal Models (D. Geiger, J. Pearl). Process, Structure, and Modularity in Reasoning with Uncertainty (B. D'Ambrosio). Probabilistic Causal Reasoning (T. Dean, K. Kanazawa). Generating Decision Structures and Causal Explanations for Decision Making (S. Star). Control of Problem Solving: Principles and Architecture (J.S. Breese, M.R. Fehling). Causal Networks: Semantics and Expressiveness (T. Verma, J. Pearl). II. Uncertainty Calculi and Comparisons. 1. Uncertainty Calculi. Stochastic Sensitivity Analysis Using Fuzzy Influence Diagrams (P. Jain, A.M. Agogino). A Linear Approximation Method for Probabilistic Inference (R.D. Shachter). Minimum Cross Entropy Reasoning in Recursive Causal Networks (W.X. Wen). Probabilistic Semantics and Defaults (E. Neufeld, D. Poole, R. Aleliunas). Modal Logics of Higher-Order Probability (P. Haddawy, A.M. Frisch). A General Non-Probabilistic Theory of Inductive Reasoning (W. Spohn). Epistemological Relevance and Statistical Knowledge (H.E. Kyburg, Jr.). Axioms for Probability and Belief-Function Propagation (P.F. Shenoy, G. Shafer). A Summary of a New Normative Theory of Probabilistic Logic (R. Aleliunas). Hierarchical Evidence and Belief Functions (P.K. Black, K.B. Laskey). On Probability Distributions over Possible Worlds (F. Bacchus). A Framework of Fuzzy Evidential Reasoning (J. Yen). 2. Comparisons. Parallel Belief Revision (D. Hunter). Evidential Reasoning Compared in a Network Usage Prediction Testbed: Preliminary Report (R.P. Loui). A Comparison of Decision Analysis and Expert Rules for Sequential Diagnosis (J. Kalagnanam, M. Henrion). An Empirical Comparison of Three Inference Methods (D. Heckerman). Modeling Uncertain and Vague Knowledge in Possibility and Evidence Theories (D. Dubois, H. Prade). Probabilistic Inference and Non-Monotonic Inference (H.E. Kyburg, Jr.). Multiple Decision Trees (S.W. Kwok, C. Carter). III. Knowledge Acquisition and Explanation. KNET: Integrating Hypermedia and Normative Bayesian Modeling (R.M. Chavez, G.F. Cooper). Generating Explanations of Decision Models Based on an Augmented Representation of Uncertainty (H.B. Jimison). IV. Applications. Induction and Uncertainty Management Techniques Applied to Veterinary Medical Diagnosis (M. Cecile, M. McLeish, P. Pascoe, W. Taylor). Predicting the Likely Behaviors of Continuous Nonlinear Systems in Equilibrium (A. Yeh). The Structure of Bayes Networks for Visual Recognition (J.M. Agosta). Utility-Based Control for Computer Vision (T.S. Levitt, T.O. Binford, G.J. Ettinger).
- Edition: 1
- Volume: 9
- Published: October 3, 2014
- Imprint: North Holland
- Language: English
TL
T.S. Levitt
Affiliations and expertise
Advanced Decision Systems, Mountain View, CA, USALK
L.N. Kanal
Affiliations and expertise
University of Maryland, Department of Computer Science, College Park, MD, USAJL
J.F. Lemmer
Affiliations and expertise
CTA, Inc., Rome, NY USARS
R.D. Shachter
Affiliations and expertise
Stanford University, Stanford, CA, USARead Uncertainty in Artificial Intelligence 4 on ScienceDirect