Limited Offer
Save 50% on book bundles
Immediately download your ebook while waiting for your print delivery. No promo code needed.
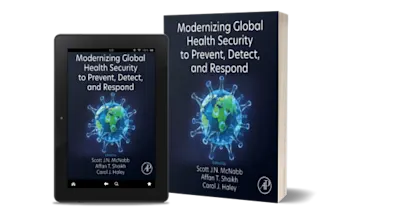
Trustworthy AI in Medical Imaging brings together scientific researchers, medical experts, and industry partners working in the field of trustworthiness, bridging the gap between A… Read more
Limited Offer
Trustworthy AI in Medical Imaging brings together scientific researchers, medical experts, and industry partners working in the field of trustworthiness, bridging the gap between AI research and concrete medical applications and making it a learning resource for undergraduates, masters students, and researchers in AI for medical imaging applications.
The book will help readers acquire the basic notions of AI trustworthiness and understand its concrete application in medical imaging, identify pain points and solutions to enhance trustworthiness in medical imaging applications, understand current limitations and perspectives of trustworthy AI in medical imaging, and identify novel research directions.
Although the problem of trustworthiness in AI is actively researched in different disciplines, the adoption and implementation of trustworthy AI principles in real-world scenarios is still at its infancy. This is particularly true in medical imaging where guidelines and standards for trustworthiness are critical for the successful deployment in clinical practice. After setting out the technical and clinical challenges of AI trustworthiness, the book gives a concise overview of the basic concepts before presenting state-of-the-art methods for solving these challenges.
ML
Marco Lorenzi is a tenured research scientist at the Inria Center of University Côte d’Azur (France), and junior chair holder at the Interdisciplinary Institute for Artificial Intelligence 3IA Côte d’Azur. He is also a visiting Senior Lecturer at the School of Biomedical Engineering & Imaging Sciences at King’s College London. His research focuses on developing statistical learning methods to model heterogeneous and secured data in biomedical applications. He is the founder and scientific responsible for the open-source federated learning platform Fed-BioMed.
MA