Trolley Crash
Approaching Key Metrics for Ethical AI Practitioners, Researchers, and Policy Makers
- 1st Edition - January 26, 2024
- Editors: Peggy Wu, Michael Salpukas, Hsin-Fu Wu, Shannon Ellsworth
- Language: English
- Paperback ISBN:9 7 8 - 0 - 4 4 3 - 1 5 9 9 1 - 6
- eBook ISBN:9 7 8 - 0 - 4 4 3 - 1 5 9 9 2 - 3
The prolific deployment of Artificial Intelligence (AI) across different fields has introduced novel challenges for AI developers and researchers. AI is permeating decision ma… Read more
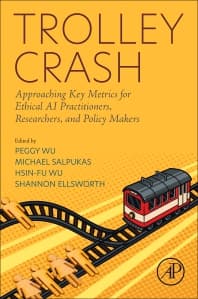
Purchase options
Institutional subscription on ScienceDirect
Request a sales quoteThe prolific deployment of Artificial Intelligence (AI) across different fields has introduced novel challenges for AI developers and researchers. AI is permeating decision making for the masses, and its applications range from self-driving automobiles to financial loan approvals. With AI making decisions that have ethical implications, responsibilities are now being pushed to AI designers who may be far-removed from how, where, and when these ethical decisions occur.
Trolley Crash: Approaching Key Metrics for Ethical AI Practitioners, Researchers, and Policy Makers provides audiences with a catalogue of perspectives and methodologies from the latest research in ethical computing. This work integrates philosophical and computational approaches into a unified framework for ethical reasoning in the current AI landscape, specifically focusing on approaches for developing metrics. Written for AI researchers, ethicists, computer scientists, software engineers, operations researchers, and autonomous systems designers and developers, Trolley Crash will be a welcome reference for those who wish to better understand metrics for ethical reasoning in autonomous systems and related computational applications.
- Presents a comparison between human oversight and ethical simulation in robots
- Introduces approaches for measuring, evaluating, and auditing ethical AI
- Investigates how AI and technology are changing human behavior
1. Introduction
Michael R. Salpukas, Peggy Wu, Shannon Ellsworth and Hsin-Fu Wu
2. Terms and References
Michael R. Salpukas, Peggy Wu, Shannon Ellsworth and Hsin-Fu Wu
How is AI Changing Human Behavior?
3. Boiling the Frog: Ethical Leniency due to Prior Exposure to Technology
Noah Ari, Nusrath Jahan, Johnathan Mell and Pamela Wisniewski
Human Oversight vs. Ethical Simulation in Robots
4. Automated Ethical Reasoners Must Be Interpretation-Capable
John Licato
5. Towards Unifying the Descriptive and Prescriptive for Machine Ethics
Taylor Olson
6. Competent Moral Reasoning in Robot Applications: Inner Dialog as a Step Towards Artificial Phronesis
John Paul Sullins III, Antonio Chella and Arianna Pipitone
Measuring, Evaluating, and Auditing Ethical AI
7. Autonomy Compliance with Doctrine and Ethics Ontological Frameworks
Donald P. Brutzman, Hsin-Fu Wu, Curtis Blais and Carl Andersen
8. Meaningful Human Control and Ethical Neglect Tolerance; Initial Thoughts on How to Define Model and Measure Them
Christopher A. Miller and Marcel Baltzer
9. Continuous automation approach for autonomous Ethics Based Audit of AI Systems
Guy Lupo, Quoc Bao Vo and Natania Locke
10. A Tiered Approach for Ethical AI Evaluation Metrics
Peggy Wu, Hsin-Fu Wu, Brett Israelsen and Robert Grabowski
11. Designing Meaningful Metrics for Demonstrating Ethical Supervision of Autonomous Systems
Donald P. Brutzman and Curtis Blais
Research Topics and Methods: Ethical AI and Big Questions
12. Obtaining Hints to Understand Language Model-based Moral Decision Making by Generating Consequences of Acts
Rafal Rzepka and Kenji Araki
13. Emerging Issues and Challenges
Michael R. Salpukas, Peggy Wu, Shannon Ellsworth and Hsin-Fu Wu
Acronyms Appendix
Hsin-Fu Wu
- No. of pages: 264
- Language: English
- Edition: 1
- Published: January 26, 2024
- Imprint: Academic Press
- Paperback ISBN: 9780443159916
- eBook ISBN: 9780443159923
PW
Peggy Wu
Peggy Wu is an award-winning scientist with over 20 years of experience combining cognitive psychology with AI in Human-Machine systems. She conducts research advancing Knowledge Representation and Reasoning, Human-Computer Etiquette, Socially Intelligent Agents, Virtual/Mixed Reality, and AI explainability, transparency, and trust applied to military, commercial, and space domains. She is a speaker, author, judge for XPrize, and an associate producer of a documentary on the psychology of Space Exploration entitled, "The Longest Goodbye." Her work has been covered by media outlets including National Geographic and the BBC.
MS
Michael Salpukas
Michael Salpukas is a research and development leader with 25 years of experience transitioning advanced algorithms and robust AI into products. He leads a portfolio of government and internally funded research and development projects designed to provide human assistance to the warfighter and rapid updates to mitigate novel inputs. Michael has authored intellectual property and publications on a variety of topics and is a regular conference panelist/moderator in AI Ethics, AI Assurance, and AI for Manufacturing. He is on the advisory board for the Northeastern University Institute for the Wireless Internet of Things, and is a Judge/Mentor for startups via Activate and MassChallenge.
HW
Hsin-Fu Wu
Hsin-Fu “Sinker” Wu performs operations research for business development and customer engagement. Sinker served a 20-year career in the U.S. Navy, in which he developed and implemented strategies for coalition, joint, naval, and undersea warfare. He co-authored a report on Ethical Control of autonomous systems for naval missions and is an AI Assurance researcher.
SE
Shannon Ellsworth
Shannon Ellsworth currently leads several fundamental research efforts to apply Artificial Intelligence and Machine Learning to U.S. Department of Defense systems. Her research is based on over 20 years of work in the field and is focused on calculated design of algorithms to support smart command and control applications. She also serves as co-chair to the AI/ML Working Group for the Military Operations Research Society.