Limited Offer
Save 50% on book bundles
Immediately download your ebook while waiting for your print delivery. No promo code needed.
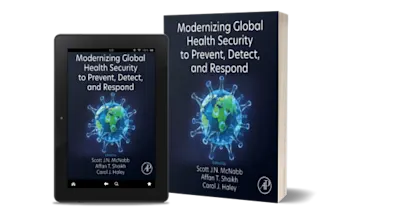
Transportation Big Data: Theory and Methods is centered around big data theory and methods. As big data is now a key topic in transport because the volume of data has increa… Read more
Limited Offer
ZL
ZG