LIMITED OFFER
Save 50% on book bundles
Immediately download your ebook while waiting for your print delivery. No promo code needed.
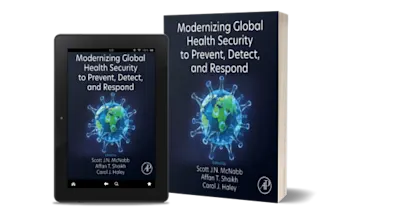
Topology optimization and AI-based design of power electronic and electrical devices provides an essential foundation in the emergent design methodology as it moves toward co… Read more
LIMITED OFFER
Immediately download your ebook while waiting for your print delivery. No promo code needed.
Topology optimization and AI-based design of power electronic and electrical devices provides an essential foundation in the emergent design methodology as it moves toward commercial development, including electrical devices as traction motors for electric motors, transformers, inductors, reactors, and power electronics circuits.
Opening with an introduction to electromagnetism and computational electromagnetics for optimal design, this book outlines principles and foundations in finite element methods and illustrates numerical techniques useful for finite element analysis. It summarizes the foundations of deterministic and stochastic optimization methods, including genetic algorithm, CMA-ES, and simulated annealing for quantum and quantum-inspired optimization, alongside representative algorithms. The book goes on to discuss parameter optimization and topology optimization of electrical devices alongside current implementations including magnetic shields, 2D and 3D models of electric motors, and wireless power transfer devices. Finally, it concludes with a lengthy exposition of AI-based design methods, including surrogate models for optimization, Bayesian optimization, direct inverse modeling, deep neural networks, explainable AI, variational autoencoder, and integrated design methods using Monte Carlo tree searches for electrical devices and circuits.
Assists researchers and design engineers in applying emergent topology design optimization to power electronics and electrical device design, supported by step-by-step methods, heuristic derivation, and pseudocodes
Proposes unique formulations of AI-based design for electrical devices using Monte Carlo tree search and other machine learning methods
Is richly accompanied by detailed numerical examples and repletes with computational support materials in algorithms and explanatory formulae
Includes access to pedagogical videos on topics including the evolutionary process of topology optimization, the distribution of genetic algorithms, and CMA-ES
Early career researchers and students studying computer-aided engineering for power electronics and electrical device applications, electromagnetic field analysis, optimal design of electric devices, and design of electronic and electrical applications. Engineers who design electrical machines such as motors, transformers, inductors, wireless power transfer systems as well as electric circuits for power electronics and other systems in automotive and electric industries. Design engineers in automotive industries seeking effective motor design methods
1. Electromagnetism for electric device design
2. Foundations of electromagnetic field analysis for electric device design
3. Optimization methods for electric device design
4. Parameter and Topology Optimization for electric device design
5. AI-based Design Methods for electric device design
HI