TinyML for Edge Intelligence in IoT and LPWAN Networks
- 1st Edition - May 29, 2024
- Editors: Bharat S Chaudhari, Sheetal N Ghorpade, Marco Zennaro, Rytis Paškauskas
- Language: English
- Paperback ISBN:9 7 8 - 0 - 4 4 3 - 2 2 2 0 2 - 3
- eBook ISBN:9 7 8 - 0 - 4 4 3 - 2 2 2 0 3 - 0
TinyML for Edge Intelligence in IoT and LPWAN Networks presents the evolution, developments, and advances in TinyML as applied to the Internet of Things (IoT) and low-power… Read more
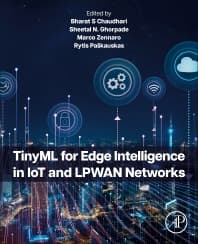
Purchase options
Institutional subscription on ScienceDirect
Request a sales quoteTinyML for Edge Intelligence in IoT and LPWAN Networks presents the evolution, developments, and advances in TinyML as applied to the Internet of Things (IoT) and low-power wide area networks (LPWANs). It starts by providing the foundations of IoT/LPWANs, low-power embedded systems and hardware, the role of AI and machine learning in communication networks in general, and cloud/edge intelligence. It then presents the concepts, methods, algorithms, and tools of TinyML. Practical applications of TinyML are given from the healthcare and industrial sectors, providing practical guidance on the design of applications and the selection of appropriate technologies.
- This book provides one-stop solutions for emerging TinyML for IoT and LPWAN applications.
- The principles and methods of TinyML are explained, with a focus on how it can be used for IoT, LPWANs, and 5G applications.
- Applications from the healthcare and industrial sectors are presented.
- Guidance on the design of applications and the selection of appropriate technologies is provided.
- Cover image
- Title page
- Table of Contents
- Copyright
- List of contributors
- Preface
- Acknowledgments
- Chapter 1: TinyML for low-power Internet of Things
- Abstract
- 1.1. Introduction
- 1.2. IoT/LPWANs
- 1.3. LPWAN applications/nodes constraints and requirements
- 1.4. Edge AI and TinyML
- 1.5. Challenges for edge AI and TinyML
- 1.6. Hardware and software considerations
- References
- Chapter 2: Embedded systems for low-power applications
- Abstract
- 2.1. Introduction
- 2.2. Key considerations and requirements for microcontroller units
- 2.3. Component selection
- 2.4. Embedded software tools for MCUs
- 2.5. Important hardware platforms
- References
- Chapter 3: Cloud and edge intelligence
- Abstract
- 3.1. Introduction
- 3.2. Edge intelligence paradigms
- 3.3. Edge intelligence: advantages and applications
- 3.4. Challenges and opportunities for edge intelligence
- 3.5. AI solutions for optimizing computation of edge intelligence
- 3.6. Architectural layers in the roadmap for edge intelligence
- 3.7. Conclusions
- References
- Chapter 4: TinyML: principles and algorithms
- Abstract
- 4.1. Introduction
- 4.2. Overview of TinyML
- 4.3. Workflow of TinyML
- 4.4. AI and edge computing
- 4.5. Edge computing optimization using AI solutions
- References
- Chapter 5: TinyML using neural networks for resource-constrained devices
- Abstract
- 5.1. Introduction
- 5.2. Background
- 5.3. Neural networks for TinyML
- 5.4. Concerns/issues in TinyML
- 5.5. Applications
- 5.6. Conclusion
- References
- Chapter 6: Reinforcement learning for LoRaWANs
- Abstract
- 6.1. Introduction
- 6.2. Reinforcement learning algorithms
- 6.3. Related work
- 6.4. Interference avoidance and energy efficiency for LoRa
- 6.5. Applications
- 6.6. Conclusion
- References
- Chapter 7: Software frameworks for TinyML
- Abstract
- 7.1. Introduction
- 7.2. Overview of TinyML
- 7.3. Challenges
- 7.4. Software and libraries
- 7.5. Advantages of TinyML
- References
- Chapter 8: Extensive energy modeling for LoRaWANs
- Abstract
- 8.1. Introduction
- 8.2. LoRa/LoRaWAN components and elements
- 8.3. LoRa energy modeling
- 8.4. Methodology
- 8.5. Case study and experimental setup
- 8.6. Conclusion
- References
- Chapter 9: TinyML for 5G networks
- Abstract
- 9.1. Introduction
- 9.2. 5G network architecture
- 9.3. Requirements for 5G use case
- 9.4. Security in 5G wide scope
- 9.5. 5G mobile network and virtualization systems
- 9.6. Multi-access edge computing for 5G
- 9.7. Open5GS, srsRAN/srsLTE, and OpenAirInterface
- 9.8. Low-power communication, massive machine type communication (mMTC), and IoT
- 9.9. 5G edge intelligence features
- 9.10. Machine learning modeling for 5G
- 9.11. TinyML for edge intelligence in 5G
- 9.12. TinyML for 5G security and data anomaly
- 9.13. Design and modeling for LPWAN-based 5G
- 9.14. 5G MEC latency standards
- 9.15. TinyML for 5G computation complexity
- References
- Chapter 10: Non-static TinyML for ad hoc networked devices
- Abstract
- 10.1. Introduction
- 10.2. Backbones and TinyML boards
- 10.3. Neural network model reduction
- 10.4. Transfer learning
- 10.5. Purely distributed federated learning
- 10.6. Challenges
- 10.7. Conclusions
- References
- Chapter 11: Bayesian-driven optimizations of TinyML for efficient edge intelligence in LPWANs
- Abstract
- 11.1. Introduction
- 11.2. Background and related work
- 11.3. Methodology
- 11.4. Proposed Bayesian optimization algorithms of TinyML in LPWANs
- 11.5. Experimental results
- 11.6. Conclusion and future directions
- References
- Chapter 12: 6TiSCH adaptive scheduling for Industrial Internet of Things
- Abstract
- 12.1. Introduction
- 12.2. Benefits of TinyML in IIoT
- 12.3. IEEE 802.15.4e TSCH
- 12.4. IPv6 over the TSCH mode of IEEE 802.15.4e (6TiSCH)
- 12.5. 6TiSCH architecture
- 12.6. 6TiSCH protocol stack
- 12.7. 6TiSCH scheduling strategies
- 12.8. Deterministic scheduling for IIoT networks
- 12.9. Traffic-aware and reliable 6TiSCH scheduling
- 12.10. Energy efficiency and reliability considerations
- 12.11. 6TiSCH simulation software
- 12.12. Challenges and limitations
- 12.13. Conclusion and future directions
- References
- Chapter 13: Securing TinyML in a connected world
- Abstract
- 13.1. Introduction
- 13.2. Related work
- 13.3. Issues and challenges in securing TinyML
- 13.4. Existing solutions and approaches for TinyML security
- 13.5. Threat models in TinyML
- 13.6. Applications of TinyML
- 13.7. Implications for future research in TinyML security
- References
- Chapter 14: TinyML applications and use cases for healthcare
- Abstract
- 14.1. Introduction
- 14.2. Wearable devices
- 14.3. Remote patient monitoring
- 14.4. Role in diagnosis and treatment by healthcare providers
- 14.5. Drug discovery
- 14.6. Ethics and TinyML
- 14.7. Conclusion
- References
- Chapter 15: Machine learning techniques for indoor localization on edge devices
- Abstract
- 15.1. Introduction
- 15.2. The challenges of indoor localization
- 15.3. State-of-the-art techniques for indoor localization
- 15.4. A tiny indoor localization implementation
- 15.5. Conclusions
- References
- Chapter 16: Embedded intelligence in Internet of Things scenarios: TinyML meets eBPF
- Abstract
- Acknowledgements
- 16.1. Introduction
- 16.2. State-of-the-art
- 16.3. Design and implementation
- 16.4. Case of study
- References
- Chapter 17: A real-time price recognition system using lightweight deep neural networks on mobile devices
- Abstract
- 17.1. Introduction
- 17.2. Related works
- 17.3. Machine learning-based techniques for image processing
- 17.4. Material and methods
- 17.5. Experimental results and discussion
- 17.6. Conclusion
- References
- Chapter 18: TinyML network applications for smart cities
- Abstract
- 18.1. Introduction
- 18.2. TinyML applications in smart cities
- 18.3. Analysis and discussion
- 18.4. Conclusion
- References
- Chapter 19: Emerging application use cases and future directions
- Abstract
- 19.1. Introduction
- 19.2. Overview of TinyML
- 19.3. Workflow steps, challenges, and proposed solutions
- 19.4. Applications of TinyML and future directions
- References
- Index
- No. of pages: 518
- Language: English
- Edition: 1
- Published: May 29, 2024
- Imprint: Academic Press
- Paperback ISBN: 9780443222023
- eBook ISBN: 9780443222030
BS
Bharat S Chaudhari
Dr. Bharat S. Chaudhari is working as a Professor (HAG) in Electrical/Electronics and Communication Engineering at MIT World Peace University, Pune, India. He graduated in Industrial Electronics Engineering from Amravati University in 1989 and received M. E. Telecom. E. and PhD (Engineering) from Jadavpur University, Kolkata, India in 1993 and 2000, respectively. He has previously held positions like Principal, Dean, and Professor at various Institutes in Pune. He is a recipient of the 2020 IETE N V Gadadhar Memorial Award, the MAEER Pune’s Ideal Teacher Award 2015, and the Young Scientist Research Grant from the Department of Science and Technology, Government of India, in 2003. He was appointed as a Visiting Scientist (Simons Associate/Regular Associate) to Wireless Network Research Group, ICTP, Trieste, Italy from January 2007 to December 2020. He is a Senior Member of IEEE, Fellow of IETE, Fellow of IE (I), and a Founder Chairman of the IEEE Pune Section (2010 – 2011). He also chaired the IEEE Communications Society, Pune Chapter. He serves as a Program Evaluator of the Engineering Accreditation Commission (EAC) of ABET, United States, for accreditation of computer, communications, and similar engineering programs. His current research interest includes LPWANs, AIoT, TinyML, and Si-Photonics.
SN
Sheetal N Ghorpade
Dr. Sheetal Ghorpade is working as Director (Data Sciences) at Rubiscape Pvt. Limited, Pune, Maharashtra, India, where she is actively involved in the research and development of data science products. She received her PhD in Applied Mathematics and MSc in Mathematics from the University of Pune, India. She is a Regular Associate (Visiting Scientist) at the Abdus Salam International Centre for Theoretical Physics in Trieste, Italy. Her career represents a great mix of data analytics and high-quality internationally collaborative research on algorithms and optimization for Industry problems. Her research interests are Optimization Techniques, Artificial Intelligence, Tiny ML, etc. She is instrumental in bridging the institute-industry gap and is passionate about democratizing data science. She is on the advisory board of various engineering and management institutes.
MZ
Marco Zennaro
Dr. Marco Zennaro is a Research Scientist at the Abdus Salam International Centre for Theoretical Physics in Trieste, Italy, where he coordinates the Science, Technology, and Innovation Unit. He received his PhD from KTH-Royal Institute of Technology, Stockholm, and his MSc in Electronic Engineering from the University of Trieste. He is a visiting Professor at KIC-Kobe Institute of Computing, Japan, and a Senior Member of IEEE and ACM. His research interest is in ICT4D, the use of ICT for Development, and in particular, he investigates the use of IoT in Developing Countries. He is TinyML4D Chair and Academic Network Co-Chair in the framework of the TinyML4D initiative. He has given lectures on wireless technologies in more than 30 different countries. More info here: https://www.ictp.it/member/marco-zennaro#biography
RP
Rytis Paškauskas
Dr. Rytis Paškauskas is a Postdoctoral Fellow at the Abdus Salam International Centre for Theoretical Physics in Trieste, Italy, where he works on embedded machine-learning applications. He received his PhD in Physics from Georgia Institute of Technology and has worked at ELETTRA Sincrotrone Trieste, CNR Pisa, CNRS Lyon, and the National Institute for Theoretical Physics (NiTheP) in Stellenbosch, South Africa.