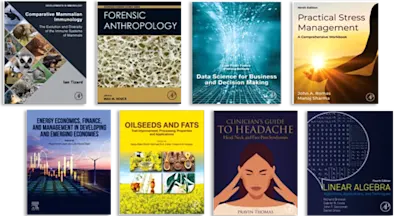
Theory of Random Functions
And Its Application to Control Problems
- 1st Edition - January 1, 1965
- Imprint: Pergamon
- Author: V. S. Pugachev
- Language: English
- eBook ISBN:9 7 8 - 1 - 4 8 3 1 - 5 6 2 5 - 5
Theory of Random Functions and Its Application to Control Problems presents insights into a branch of probability theory, the theory of random functions, which studies and takes… Read more
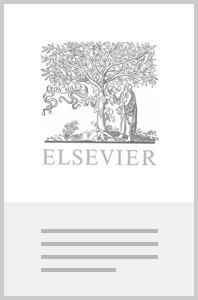
Purchase options
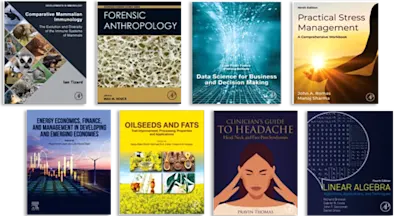
Institutional subscription on ScienceDirect
Request a sales quoteTheory of Random Functions and Its Application to Control Problems presents insights into a branch of probability theory, the theory of random functions, which studies and takes into account the effects of random factors on the functioning of control systems. The book does not require a high level of competency in the use of mathematical techniques and explains the basics of probability theory before focusing on the concepts of the theory of random functions. The selection also discusses in great detail the aspects of random functions and provides chapters that cover the determination and solution to problems of optimal systems. The text will be of value to telecommunications engineers, aeronautical engineers, meteorologists, seismologists, and other professionals engaged in applied sciences.
Foreword to First Edition
Foreword to Second Edition
Foreword to Third Edition
Chapter 1. The Probability of an Event and Its Properties
§ 1. Random Phenomena. The Object of Probability Theory
§ 2. Experimental Foundations of the Theory of Probability. Frequency and Probability
§ 3. The Addition Theorem of Frequencies. The Addition Principle of Probabilities
§ 4. Conditional Frequencies and Conditional Probabilities. Dependent and Independent Events
§ 5. The Formula of Total Probability. Bayes' Formula
§ 6. Repeated Trials
Chapter 2. Random Variables
§ 7. Distribution Functions
§ 8. Probability Density
§ 9. The Use of Impulse Functions and Generalization of the Concept of Probability Density
§ 10. Moments of Random Variables. Mathematical Expectation, Dispersion and Root Mean Square Deviation
§ 11. The Normal Distribution Law (Gaussian Distribution)
§ 12. The Poisson Distribution
§ 13. Approximate Analytical Representation of Probability Distributions
Chapter 3. Random Vector Variables
§ 14. Distribution Function of a Random Vector
§ 15. Probability Density Function of a Random Vector
§ 16. Conditional Distribution Functions and Conditional Probability Densities
§ 17. Moments of a Two-Dimensional Random Vector. The Covariance and the Correlation Coefficient
§ 18. Moments of a Multivariate Random Vector. The Variance-Covariance Matrix of a Random Vector
§ 19. The Mathematical Expectation of a Complex Random Variable. Properties of Mathematical Expectations
§ 20. Dispersions and Covariances of Complex Random Variables. Properties of Dispersions and Covariances
§ 21. The Transformation of a General Random Vector to One with Uncorrelated Components
§ 22. Bivariate Normal Distribution Law
§ 23. Multivariate Normal Distribution Law
§ 24. Root Mean Square Approximation to Random Vectors
Chapter 4. Characteristic Functions of a Random Variable
§ 25. The Characteristic Function of a Scalar Random Variable
§ 26. an Expression for the Probability Density Function in the Terms of the Characteristic Function
§ 27. The Relationship between the Characteristic Function and the Moments of a Random Variable
§ 28. The Characteristic Function of a Random Vector
§ 29. The Relation between the Characteristic Function and the Moments of a Random Vector
Chapter 5. Functions with Random Arguments
§ 30. Determination of the Moments of Functions of Random Variables
§ 31. Linearization of Functions as a Means of Approximately Determining the Moments of Non-linear Functions of Random Variables
§ 32. The Distribution Law of a Function of a Random Variable
§ 33. Another Method for Determining the Distribution Law of a Function of a Random Variable
§ 34. The Distribution Function of a Sum of Random Variables
§ 35. Application of Characteristic Functions to Determine the Distribution Laws of Functions of Random Variables
Chapter 6. The Law of Large Numbers
§ 36. Chebyshev's Inequality
§ 37. Theorems of Markov and Chebyshev. Convergence in Probability
§ 38. Theorems of Poisson and Bernoulli
§ 39. Theorems of Ljapunov and Laplace
§ 40. The Proof of Ljapunov's Theorem
Chapter 7. Fundamental Concepts of Information Theory
§ 41. Measuring the Uncertainty of Observational Results
§ 42. Entropy of a Discrete Random Variable
§ 43. The Entropy of a Continuous Random Variable
§ 44. Information and How to Measure It
§ 45. The Entropy of Uniform and Normal Distributions
§ 46. Uniqueness of the Definition of Entropy for Discrete Random Variables
§ 47. The Entropy of an Infinite Stochastic Sequence
Chapter 8. Random Functions
§ 48. Random Functions. Distributions of a Random Function
§ 49. The Mathematical Expectation and Covariance Function of a Random Function. The Cross Covariance Function of Two Random Functions
§ 50. Moments of Random Functions
§ 51. Properties of Covariance Functions
§ 52. The Addition of Random Functions
§ 53. Differentiation of a Random Function
§ 54. Integration of a Random Function
§ 55. Limit Theorem for the Mean Value of a Random Function. General Ergodic Theorem
Chapter 9. Canonical Expansions of Random Functions
§ 56. Two Types of Canonical Expansions for Random Functions
§ 57. General Formula for Co-ordinate Functions
§ 58. Canonical Expansion of a Random Function over a Discrete Series of Points
§ 59. A Practical Method of Constructing a Canonical Expansion for a Random Function over a Discrete Series of Points
§ 60. Canonical Expansion of a Random Function in a Prescribed Domain of the Argument
§ 61. A Practical Method of Forming the Canonical Expansion of a Random Function Over a Given Range of Variation of the Argument
§ 62. General Form of the Canonical Expansion of a Random Function
§ 63. The Construction of a Canonical Expansion for a Random Function from The Canonical Expansion of Its Covariance Function
§ 64. Methods of Forming Canonical Expansions for Covariance Functions
§ 65. A Method of Forming an Approximate Canonical Expansion of a Random Function
§ 66. The Series Expansion of a Random Function
§ 67. Integral Canonical Expansions of Random Functions
Chapter 10. Vector Random Functions
§ 68. The Relationship between Scalar and Vector Random Functions
§ 69. The Mathematical Expectation and Covariance Function of a Vector Random Function
§ 70. Canonical Expansions of Vector Random Functions
§ 71. Integral Canonical Expansions of Vector Random Functions
Chapter 11. Stationary Random Functions
§ 72. Definition of a Stationary Random Function
§ 73. The Stationary Vector Random Function
§ 74. The Ergodic Property of Stationary Random Functions
§ 75. Stationary Random Functions Which are Ergodic With Respect to the Covariance Function
§ 76. The Integral Canonical Expansion of a Stationary Random Function. The Spectral Density of a Stationary Random Function
§ 77. An Approximate Canonical Expansion of a Stationary Random Function
§ 78. The Integral Canonical Expansion of a Stationary Vector Random Function
§ 79. The Approximate Canonical Expansion of a Stationary Vector Random Function
§ 80. Random Functions Which Can Be Represented in Terms of Stationary Random Functions
Chapter 12. Characteristics of Dynamic Systems
§ 81. Transformation of Functions by Dynamic Systems. Operators
§ 82. The Operator of a Dynamic System as Its General Characteristic
§ 83. Weighting Functions of Uni-dimensional Linear Systems
§ 84. Uni-dimensional Linear Systems Described By Differential Equations
§ 85. Weighting Functions of Multi-Dimensional Linear Systems
§ 86. Other Characteristics of Linear Systems
§ 87. Stationary Linear Systems
Chapter 13. The Accuracy of Linear Systems
§ 88. Linear Transformation of Random Functions
§ 89. Linear Transformation of a Vector Random Function
§ 90. General Methods of Investigating the Accuracy of Linear Systems
§ 91. Methods of Calculating the Steady-State Systematic Errors of Stationary Linear Systems
§ 92. The Accuracy of a Uni-dimensional Stationary Linear System with One Stationary Random Input
§ 93. The Accuracy of a Uni-dimensional Stationary Linear System with One Non-Stationary Random Input
§ 94. The Accuracy of Quasi-Stationary Uni-dimensional Linear Systems
§ 95. The Accuracy of Multi-Dimensional Stationary Linear Systems
§ 96. The Accuracy of Multi-Dimensional Quasi-Stationary Linear Systems
§ 97. A Type of Integral Canonical Representation for Random Inputs
§ 98. The Transformation of a Random Function by a Random Linear Integral Operator
Chapter 14. Methods of Investigating the Accuracy of Non-linear Systems
§ 99. Methods of Investigating the Accuracy of Non-linear Systems
§ 100. General Principles of the Linearization of Operators
§ 101. Direct Linearization of the Equations of Non-linear Systems
§ 102. Linearization by Canonical Expansions
§ 103. Statistical Linearization
§ 104. The Use of Statistical Linearization in Investigating the Error of Stationary Systems
§ 105. The Use of Statistical Linearization in Investigating the Error of Nonstationary Systems
§ 106. Transforms of Random Functions Which are Reducible to Linearity
§ 107. Non-Linear Integral Transforms
§ 108. Application of the Method of Canonical Expansions to the Analysis of Non-Linear Transforms of Random Functions
Chapter 15. Determination of the Characteristics of Random Variables and Random Functions from the Results of Trials
§ 109. The Nature of the Problem
§ 110. Determination of the Probabilities of Events, Distribution Functions, and Probability Density Functions
§ 111. Determination of the Mathematical Expectations and Dispersions of Random Variables
§ 112. The Covariance between Random Variables
§ 113. Estimation of the Error in Experimental Determination of Probability Characteristics
§ 114. Determination of the Mathematical Expectation and Covariance Function of an Ergodic Stationary Random Function
§ 115. Estimation of the Mathematical Expectation of a Random Function by Smoothing Its Realizations
§ 116. Fundamentals of the Theory of Estimators
§ 117. Use of the Method of Maximum Likelihood to Construct an Estimator of the Mathematical Expectation of a Random Function
Chapter 16. Problems in the Theory of Optimal Systems
§ 118. Problems of Defining Optimal Systems
§ 119. Criteria of Optimality
§ 120. The General Condition of Minimum Mean Square Error
§ 121. The General Conditions for the Extremum of a Given Function of the Mathematical Expectation and Dispersion of Error
§ 122. Equations of Optimal Linear Operators
§ 123. Equations of the Optimal Non-Homogeneous Linear Transform
§ 124. General Analysis of the Equations of the Optimal Linear Operator
§ 125. Equations Determining the Weighting Functions of Optimal Linear Systems
§ 126. The Equation of the Optimal Non-Linear Integral Operator
Chapter 17. Methods of Determining Optimal Linear Systems
§ 127. The Optimal Uni-dimensional Linear System with White Noise Input
§ 128. A General Formula for the Weighting Function of an Optimal Uni-dimensional Linear System
§ 129. Formula for Determining Optimal Linear Systems for an Infinite Observation Interval and a Stationary Random Input Function
§ 130. Determination of the Optimal Linear System When the Input is Related to White Noise by a Linear Differential Equation
§ 131. Other Versions of the Method of Determining the Optimal Linear System When The Input is Related to White Noise by a Linear Differential Equation
§ 132. The Case When the Input is a Stationary Random Function with A Rational Fractional Spectral Density
§ 133. Determination of the Optimal Linear Operator by Integral Canonical Expansions in the General Case
§ 134. Determination of the Optimal Uni-dimensional Linear System by Canonical Expansions
§ 135. Determination of the Optimal Linear Operator by Canonical Expansions in the General Case
§ 136. Determination of the Optimal Linear Operator in Special Cases
§ 137. Uniqueness of the Solution and the Degree of approximation to the Optimal Linear Operator with the Criterion of Minimum Mean Square Error
Chapter 18. Methods of Determining Optimal Non-linear Systems
§ 138. The Optimal Operator in the Class of Operators Which Are Reducible to Linearity
§ 139. The Optimal Non-linear Integral Operator
§ 140. The Optimal Operator According to the Criterion of Minimum Mean Square Error
§ 141. The Optimal Operator According to the Criterion of Minimum Mean Risk for an Arbitrary Loss Function
§ 142. The Optimal Operator According to the Criterion of Minimum Mean Risk in Special Cases
§ 143. Normally Distributed Signal and Noise
§ 144. The Optimal Operator According to the Criterion of Minimum Mean Risk in the General Case
§ 145. The Case When the Loss Function is a Functional, and the Signal and Noise are Normally Distributed
Supplement
I. Basic Concepts of the Theory of Linear Transforms
II. Basic Concepts of the Theory of Linear Integral Equations with a Symmetric Kernel
III. Determination of the Minimum of a Function or Functional by the Method of Steepest Descent
Appendix. Tables of Formula and Tables of Functions
Table I. Formula for the Statistical Transfer Constants of Typical Non-linear Elements
Table II. Formula for Integrals of Rational Fractional Even Functions
Table III. Values of the Function Φ (u) =
Table IV. Values of the Function Φ’(u) =
Table V. Values of the Derivatives of Function Φ(u)
Table VI. Values of Tp, Defined by the Equality p = Sk(tp)
Table VII. Values of the Function Lk(Εp)
References
Supplementary References
Index
- Edition: 1
- Published: January 1, 1965
- No. of pages (eBook): 852
- Imprint: Pergamon
- Language: English
- eBook ISBN: 9781483156255
Read Theory of Random Functions on ScienceDirect