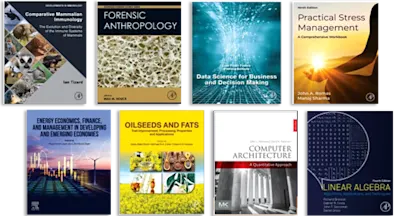
The Spectral Analysis of Time Series
Probability and Mathematical Statistics, Vol. 22
- 1st Edition - May 12, 2014
- Imprint: Academic Press
- Author: L. H. Koopmans
- Editors: Z. W. Birnbaum, E. Lukacs
- Language: English
- eBook ISBN:9 7 8 - 1 - 4 8 3 2 - 1 8 5 4 - 0
The Spectral Analysis of Time Series describes the techniques and theory of the frequency domain analysis of time series. The book discusses the physical processes and the basic… Read more
Purchase options
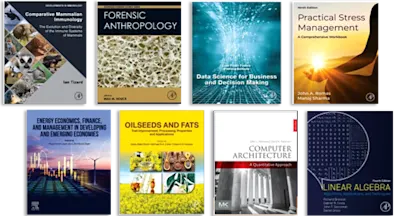
The Spectral Analysis of Time Series describes the techniques and theory of the frequency domain analysis of time series. The book discusses the physical processes and the basic features of models of time series. The central feature of all models is the existence of a spectrum by which the time series is decomposed into a linear combination of sines and cosines. The investigator can used Fourier decompositions or other kinds of spectrals in time series analysis. The text explains the Wiener theory of spectral analysis, the spectral representation for weakly stationary stochastic processes, and the real spectral representation. The book also discusses sampling, aliasing, discrete-time models, linear filters that have general properties with applications to continuous-time processes, and the applications of multivariate spectral models. The text describes finite parameter models, the distribution theory of spectral estimates with applications to statistical inference, as well as sampling properties of spectral estimates, experimental design, and spectral computations. The book is intended either as a textbook or for individual reading for one-semester or two-quarter course for students of time series analysis users. It is also suitable for mathematicians or professors of calculus, statistics, and advanced mathematics.
PrefaceAcknowledgmentsChapter 1 Preliminaries 1.1 Introduction 1.2 Time Series and Spectra 1.3 Summary of Vector Space Geometry 1.4 Some Probability Notations and PropertiesChapter 2 Models for Spectral Analysis—The Univariate Case 2.1 Introduction 2.2 The Wiener Theory of Spectral Analysis 2.3 Stationary and Weakly Stationary Stochastic Processes 2.4 The Spectral Representation for Weakly Stationary Stochastic Processes—A Special Case 2.5 The General Spectral Representation for Weakly Stationary Processes 2.6 The Discrete and Continuous Components of the Process 2.7 Physical Realizations of the Different Kinds of Spectra 2.8 The Real Spectral Representation 2.9 Ergodicity and the Connection between the Wiener and Stationary Process Theories 2.10 Statistical Estimation of the Autocovariance and the Mean Ergodic Theorem Appendix to Chapter 2Chapter 3 Sampling, Aliasing, and Discrete-Time Models 3.1 Introduction 3.2 Sampling and the Aliasing Problem 3.3 The Spectral Model for Discrete-Time SeriesChapter 4 Linear Filters—General Properties with Applications to Continuous-Time Processes 4.1 Introduction 4.2 Linear Filters 4.3 Combining Linear Filters 4.4 Inverting Linear Filters 4.5 Nonstationary Processes Generated by Time Varying Linear Filters Appendix to Chapter 4Chapter 5 Multivariate Spectral Models and Their Applications 5.1 Introduction 5.2 The Spectrum of a Multivariate Time Series-Wiener Theory 5.3 Multivariate Weakly Stationary Stochastic Processes 5.4 Linear Filters for Multivariate Time Series 5.5 The Bivariate Spectral Parameters, Their Interpretations and Uses 5.6 The Multivariate Spectral Parameters, Their Interpretations and Uses Appendix to Chapter 5Chapter 6 Digital Filters 6.1 Introduction 6.2 General Properties of Digital Filters 6.3 The Effect of Finite Data Length 6.4 Digital Filters with Finitely Many Nonzero Weights 6.5 Digital Filters Obtained by Combining Simple Filters 6.6 Filters with Gapped Weights and Results Concerning the Filtering of Series with Polynomial Trends Appendix to Chapter 6Chapter 7 Finite Parameter Models, Linear Prediction, and Real-Time Filtering 7.1 Introduction 7.2 Moving Averages 7.3 Autoregressive Processes 7.4 The Linear Prediction Problem 7.5 Mixed Autoregressive-Moving Average Processes and Recursive Prediction 7.6 Linear Filtering in Real Time Appendix to Chapter 7Chapter 8 The Distribution Theory of Spectral Estimates with Applications to Statistical Inference 8.1 Introduction 8.2 Distribution of the Finite Fourier Transform and the Periodogram 8.3 Distribution Theory for Univariate Spectral Estimators 8.4 Distribution Theory for Multivariate Spectral Estimators with Applications to Statistical Inference Appendix to Chapter 8Chapter 9 Sampling Properties of Spectral Estimates, Experimental Design, and Spectral Computations 9.1 Introduction 9.2 Properties of Spectral Estimators and the Selection of Spectral Windows 9.3 Experimental Design 9.4 Methods for Computing Spectral Estimators 9.5 Data Processing Problems and Techniques Appendix to Chapter 9ReferencesIndex
- Edition: 1
- Published: May 12, 2014
- Imprint: Academic Press
- Language: English
EL
E. Lukacs
Affiliations and expertise
Bowling Green State UniversityRead The Spectral Analysis of Time Series on ScienceDirect