Limited Offer
Save 50% on book bundles
Immediately download your ebook while waiting for your print delivery. No promo code needed.
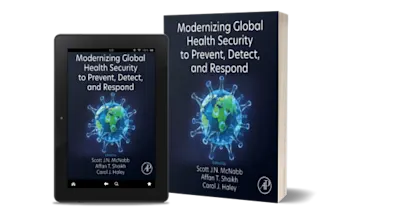
The Era of Artificial Intelligence, Machine Learning and Data Science in the Pharmaceutical Industry examines the drug discovery process, assessing how new technologies h… Read more
Limited Offer
The Era of Artificial Intelligence, Machine Learning and Data Science in the Pharmaceutical Industry examines the drug discovery process, assessing how new technologies have improved effectiveness. Artificial intelligence and machine learning are considered the future for a wide range of disciplines and industries, including the pharmaceutical industry. In an environment where producing a single approved drug costs millions and takes many years of rigorous testing prior to its approval, reducing costs and time is of high interest. This book follows the journey that a drug company takes when producing a therapeutic, from the very beginning to ultimately benefitting a patient’s life.
This comprehensive resource will be useful to those working in the pharmaceutical industry, but will also be of interest to anyone doing research in chemical biology, computational chemistry, medicinal chemistry and bioinformatics.
Academic and industrial researchers interested in drug discovery, chemical biology, computational chemistry, medicinal chemistry, and bioinformatics
SA