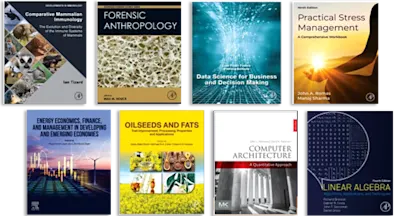
Systems Biology and In-Depth Applications for Unlocking Diseases
Principles, Tools, and Application to Disease
- 1st Edition - November 5, 2024
- Imprint: Academic Press
- Editor: Babak Sokouti
- Language: English
- Paperback ISBN:9 7 8 - 0 - 4 4 3 - 2 2 3 2 6 - 6
- eBook ISBN:9 7 8 - 0 - 4 4 3 - 2 2 3 2 7 - 3
Systems Biology and In-Depth Applications for Unlocking Diseases provides the essence of systems biology approaches in a practical manner illustrating the basic principles essen… Read more
Purchase options
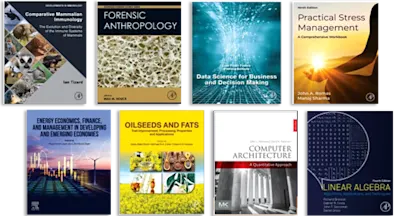
Systems Biology and In-Depth Applications for Unlocking Diseases provides the essence of systems biology approaches in a practical manner illustrating the basic principles essential to develop and model in real life science applications. Methodologies covered show how to interrogate biological data, with the purpose of obtaining insight about disease diagnosis, prognosis, and treatment.
Systematically written in 4 parts, this book first provides an introduction and history of systems biology; second, it provides the tools and resources needed for the structure and function of biological systems; next, it provides the evidence of systems biology in action to better understand disease connections; and finally, it provides the extensions of systems biology in various scientific fields including pharmacology, immunology, vaccinology, neuroscience, virology, and medicine. Examples include big data techniques, scale networks, mathematical model development, and much more.
This is the perfect reference to provide the fundamental base of knowledge needed for systems biologists, professionals in systems medicine, computational biologists, and bioinformaticians, whether needed for immediate application or for building a comprehensive understanding of the field.
- Provides detailed and comprehensive coverage of the field of systems biology
- Delivers instruction on how to interrogate biological data, with the purpose of obtaining insight about disease diagnosis, prognosis, and treatment
- Makes effective steps towards personalized medicine in the treatment of disease
- Explains effective disease treatment strategies at early diagnosis stages
Systems biologists, professionals in systems medicine, computational biologists, and bioinformaticians, Pharmaceutical industries, biotechnologists
- Systems biology at a glance
- Brief information on cellular biology and signaling pathways
- The multiscale causality nature of human cancer: A systemic approach
- From sketch to landscape: Transforming neuronal concepts across technological change
- Challenges in evolutionary computing in the context of integrated bioinformatics
- Kinetics and dynamics of biological systems
- Biological data sources for advancement in system biology
- High-throughput data analysis in systems biology: Techniques, challenges and applications in modern scientific research
- High throughput data: Single-nucleotide polymorphisms in depth
- The significance and evolution of biological databases in systems biology
- Programming, tools, and software
- Big data analysis techniques
- Reconstruction of genomic and proteomic scale network structures and functions
- Validation strategies in systems biology research
- Systems in pharmacology – principles, methods, and applications
- Systems immunology
- Systems virology
- Systems vaccinology
- Systems neuroscience
- Implications of systems in biology understanding the pathophysiology of neurological diseases
- Systems medicine
- From systems thinking to P4 medicine
- Systems biology of cancer
- Repurposing, effects, design, and discovery of drugs in systems biology
- Future directions on systems biology
- Edition: 1
- Published: November 5, 2024
- Imprint: Academic Press
- Language: English
BS