SUSTAINABLE DEVELOPMENT
Innovate. Sustain. Transform.
Save up to 30% on top Physical Sciences & Engineering titles!
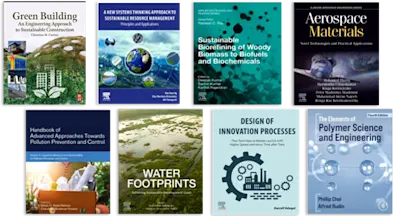
Synthetic Aperture Radar Image Processing Algorithms for Nonlinear Oceanic Turbulence and Front Modelling is both a research and practice-based reference that bridges the gap betwe… Read more
SUSTAINABLE DEVELOPMENT
Save up to 30% on top Physical Sciences & Engineering titles!
Synthetic Aperture Radar Image Processing Algorithms for Nonlinear Oceanic Turbulence and Front Modelling is both a research and practice-based reference that bridges the gap between the remote sensing field and the dynamic oceanography exploration field. In this perspective, the book explicates how to apply techniques in synthetic aperture radar and quantum interferometry synthetic aperture radar (QInSAR) for oceanic turbulence and front simulation and modeling. It includes detailed algorithms to enable readers to better understand and implement practices covered in their own work and apply QInSAR to their own research.
This multidisciplinary reference is useful for researchers and academics in dynamic oceanography and modeling, remote sensing and aquatic science, as well as geographers, geophysicists, and environmental engineers.
Researchers and academics in dynamic oceanography and modelling, remote sensing and aquatic science Geographers, Geophysicists, Environmental Engineers
MM
Distinguished Professor Dr. Maged Marghany, the visionary behind the innovative theory titled "Quantized Marghany’s Front," currently holds the esteemed position of Director at Global Geoinformation in Malaysia. Acknowledged globally for his exceptional contributions, Dr. Marghany achieved recognition by Stanford University, USA, by being listed among the top 2% of scientists for four consecutive years - 2020, 2021, 2022, and 2023. Furthermore, his profound impact is reflected in the recognition of two of his books, which were acknowledged among the best genetic algorithm books of all time. Dr. Marghany's ongoing commitment to excellence continues to shape the landscape of scientific thought and geoinformation expertise.
Additionally, Dr. Maged Marghany achieved the remarkable distinction of being ranked first among oil spill scientists in a global list spanning the last 50 years, compiled by the prestigious Universidade Estadual de Feira de Santana in Brazil. His expertise also extended to the role of a prominent visiting professor at Syiah Kuala University in Indonesia.
In previous roles, Dr. Marghany directed the Institute of Geospatial Applications at the University of Geomatica College. His educational journey includes a post-doctoral degree in radar remote sensing, a PhD in environmental remote sensing, and a Master of Science in physical oceanography. With over 250 papers and influential books," Dr. Marghany ‘s significant contributions shape global perspectives in remote sensing, geospatial applications, and environmental science.