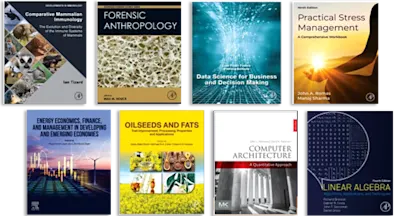
Supervised Learning in Remote Sensing and Geospatial Science
- 1st Edition - October 1, 2025
- Imprint: Elsevier
- Authors: Aaron E Maxwell, Christopher Ramezan, Yaqian He
- Language: English
- Paperback ISBN:9 7 8 - 0 - 4 4 3 - 2 9 3 0 6 - 1
- eBook ISBN:9 7 8 - 0 - 4 4 3 - 2 9 3 0 7 - 8
Supervised Learning in Remote Sensing and Geospatial Science is an invaluable resource focusing on practical applications of supervised learning in remote sensing and geospatia… Read more
Purchase options
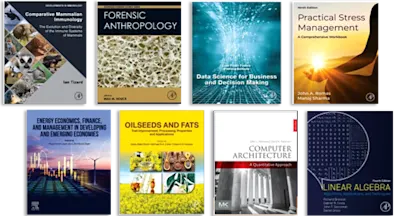
Supervised Learning in Remote Sensing and Geospatial Science is an invaluable resource focusing on practical applications of supervised learning in remote sensing and geospatial data science. Emphasizing practicality, the book delves into creating labeled datasets for training and evaluating models. It addresses common challenges like data imbalance and offers methods for assessing model performance. This guide bridges the gap between theory and practice, providing tools and techniques for extracting actionable information from raw geospatial data.
The book covers all aspects of supervised learning workflows, including preparing diverse remotely sensed and geospatial data inputs. It equips researchers, practitioners, and students with essential knowledge for applied mapping and modeling tasks, making it an indispensable reference for advancing geospatial science.
- Provides a practical handbook for implementing supervised machine learning techniques to geospatial data, with step-by-step methodologies and case studies
- Discusses the full spectrum of machine and deep learning methods for classification and regression tasks
- Focuses on applied implementation, common issues, pitfalls, and best practices, providing practical considerations on dealing with these problems
Remote sensing analysts, GIS analysts, geospatial analysts, geospatial data scientists, cartographers, data scientists, geographers, and research scientists. Also advanced/postgraduate students in the geosciences.
Geography, Geology, Geoscience, Earth Science, Sustainability, Geoinformatics, Forestry, Agriculture, Atmosphere, Ecology, Resource Management, or Engineering departments. Such individuals are often tasked with extracting actionable information from remotely sensed and geospatial data. Examples include generating land cover maps from satellite imagery, identifying disease-infected crops, extracting or identifying features of interest in imagery, and predicting a continuous measure at each pixel location (e.g., total aboveground live biomass or hurricane intensity). Such individuals could use this text to guide them in pre-processing data, developing training and validation samples, training and tuning models, improving models, dealing with poor model performance, and evaluating model output.
Part I: Supervised Learning and Key Principles
1. Introduction to the Supervised Learning Proces
What is supervised learning?
What is artificial intelligence and machine learning?
Uses of supervised learning in remote sensing and geospatial science
Supervised vs. unsupervised learning
Overview of the supervised learning process
Data requirements
Bias and variance
Model performance and assessment
Overfitting and generalization
Toward reproducibility and replicability
Introduction to provided R and Python examples
2. Training Data and Labels
Training data requirements and considerations
Collection and labelling methods
Sampling
Quality assurance and quality control
Optimizing reproducibility and replicability
3. Accuracy Assessment
Validation data characteristics and considerations
Assessment metrics
Estimating the uncertainty of predictions
Comparing model performance
4. Predictor Variables and Data Considerations
Remotely sensed data sources
Ancillary geospatial data
Complexities of working with multiple data sources
Feature space engineering
Data pre-processing
Part II: Supervised Learning Algorithms
5. Supervised Learning with Linear Methods
Linear regression
Multiple linear regression
Generalized linear models (GLMs)
Regression for binary classification
Generalized additive models (GAMs)
6. Machine Learning Algorithms
k-nearest neighbour (kNN) algorithm
Tree-based methods
Kernel-based methods
Artificial neural networks
Ensemble methods
7. Tuning Hyperparameter and Improving Models
Hyperparameter tuning
Improving models
8. Geographic Object-Based Image Analysis (GEOBIA)
Why use object-based methods?
Image segmentation algorithms
Multiscale image segmentation
Feature summarization
Classifying objects and selecting training and validation objects
Post-processing
Accuracy assessment considerations
Part III: Supervised Learning with Deep Learning
9. Deep Learning for Scene-Level Problems
Review of artificial neural networks (ANNs)
Tensor data model
Computational requirements
Loss metrics
Optimization
Activation functions
Training process
Fully connected neural networks
Convolutional neural networks (CNNs)
10. Deep Learning for Pixel-Level Problems
Upsampling and transpose convolution
Fully convolutional neural networks (FCNNs)
Encoder-decoder architectures
Spatial pyramid pooling and multiscale architectures
Encoder backbones
PointRend
Instance segmentation
Accuracy Assessment considerations
11. Improving Deep Learning Models
Preparing input data
Choosing an architecture
Dealing with small sample sizes
Class imbalance
Architectural manipulations
Augmenting the learning process
Semi-supervised learning
12. Frontiers and Supervised Learning at Scale
Vision transformers
Learning from unlabelled data
High performance computing
- Edition: 1
- Published: October 1, 2025
- Imprint: Elsevier
- Language: English
AE
Aaron E Maxwell
Aaron Maxwell is an Assistant Professor in the Department of Geology and Geography at West Virginia University. He is also the director of West Virginia View, an AmericaView member organization, and a faculty director of the West Virginia GIS Technical Center. He holds a PhD in Geology from West Virginia University and is a West Virginia native. The primary objectives of his work are to investigate computational methods to extract useful information from geospatial data that can inform decision making and to train students to be effective and thoughtful geospatial scientists and professionals. His teaching focuses on geographic information science (GISc), remote sensing, and geospatial data science. His research interests include spatial predictive modeling, accuracy assessment, applications of machine learning and deep learning in the geospatial sciences, digital terrain analysis, geographic object-based image analysis (GEOBIA), and geomorphic and forest mapping and modeling.
CR
Christopher Ramezan
Christopher Ramezan is an Assistant Professor in the Department of Management Information Systems at West Virginia University. He is also the program director of the Master of Science in Business Cybersecurity Management program in the John Chambers College of Business and Economics. He received his Ph.D. in 2019 in Geography from West Virginia University, specializing in remote sensing. His research interests in remote sensing include applied machine learning, sample selection, model optimization, image segmentation, geographic object-based image analysis (GEOBIA), and land-use land-cover classification. He currently teaches courses on data and network communications, enterprise security architecture, operational technology and industrial control systems security, and cybersecurity data analytics. He has over 10 years’ experience in the information technology field and was the former information security officer of the Eberly College of Arts and Sciences at West Virginia University. He also holds over 20 industry certifications including the CISSP, CISM, CASP, and CDPSE.
YH