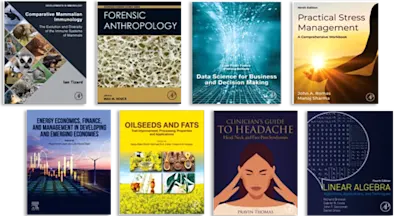
Statistical Modeling Using Local Gaussian Approximation
- 1st Edition - October 5, 2021
- Imprint: Academic Press
- Authors: Dag Tjøstheim, Håkon Otneim, Bård Støve
- Language: English
- Paperback ISBN:9 7 8 - 0 - 1 2 - 8 1 5 8 6 1 - 6
- eBook ISBN:9 7 8 - 0 - 1 2 - 8 1 5 4 4 5 - 8
Statistical Modeling using Local Gaussian Approximation extends powerful characteristics of the Gaussian distribution, perhaps, the most well-known and most used distribut… Read more
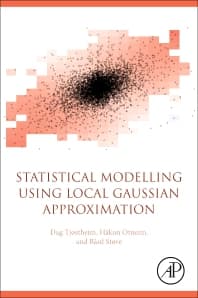
Purchase options
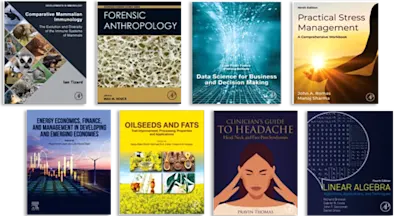
Institutional subscription on ScienceDirect
Request a sales quoteStatistical Modeling using Local Gaussian Approximation extends powerful characteristics of the Gaussian distribution, perhaps, the most well-known and most used distribution in statistics, to a large class of non-Gaussian and nonlinear situations through local approximation. This extension enables the reader to follow new methods in assessing dependence and conditional dependence, in estimating probability and spectral density functions, and in discrimination. Chapters in this release cover Parametric, nonparametric, locally parametric, Dependence, Local Gaussian correlation and dependence, Local Gaussian correlation and the copula, Applications in finance, and more.
Additional chapters explores Measuring dependence and testing for independence, Time series dependence and spectral analysis, Multivariate density estimation, Conditional density estimation, The local Gaussian partial correlation, Regression and conditional regression quantiles, and a A local Gaussian Fisher discriminant.
- Reviews local dependence modeling with applications to time series and finance markets
- Introduces new techniques for density estimation, conditional density estimation, and tests of conditional independence with applications in economics
- Evaluates local spectral analysis, discovering hidden frequencies in extremes and hidden phase differences
- Integrates textual content with three useful R packages
- Cover image
- Title page
- Table of Contents
- Copyright
- Dedication
- Biography
- Preface
- Chapter 1: Introduction
- Abstract
- 1.1. Computer code
- References
- Chapter 2: Parametric, nonparametric, locally parametric
- Abstract
- 2.1. Introduction
- 2.2. Parametric density models
- 2.3. Parametric regression models
- 2.4. Time series
- 2.5. Nonparametric density estimation
- 2.6. Nonparametric regression estimation
- 2.7. Fighting the curse of dimensionality
- 2.8. Quantile regression
- 2.9. Semiparametric models
- 2.10. Locally parametric
- References
- Chapter 3: Dependence
- Abstract
- 3.1. Introduction
- 3.2. Weaknesses of Pearson's ρ
- 3.3. The copula
- 3.4. Global dependence functionals and tests of independence
- 3.5. Test functionals generated by local dependence relationships
- References
- Chapter 4: Local Gaussian correlation and dependence
- Abstract
- 4.1. Introduction
- 4.2. Local dependence
- 4.3. Local Gaussian correlation
- 4.4. Limit theorems
- 4.5. Properties
- 4.6. Examples
- 4.7. Transforming the marginals: Normalized local correlation
- 4.8. Some practical considerations
- 4.9. The p-dimensional case
- 4.10. Proof of asymptotic results
- References
- Chapter 5: Local Gaussian correlation and the copula
- Abstract
- 5.1. Introduction
- 5.2. Local Gaussian correlation for copula models
- 5.3. Examples
- 5.4. Recognizing copulas by goodness-of-fit
- 5.5. A real-data study
- References
- Chapter 6: Applications in finance
- Abstract
- 6.1. Introduction
- 6.2. Conditional correlation and the bias problem
- 6.3. Empirical analysis of dependence of financial returns
- 6.4. The portfolio allocation problem
- 6.5. Financial contagion
- References
- Chapter 7: Measuring dependence and testing for independence
- Abstract
- 7.1. Introduction
- 7.2. Testing of independence in iid pairs of variables using local correlation functionals
- 7.3. Testing for serial independence in time series
- 7.4. Describing nonlinear dependence and tests of independence for two time series
- 7.5. Proofs
- References
- Chapter 8: Time series dependence and spectral analysis
- Abstract
- 8.1. Introduction
- 8.2. Local Gaussian spectral densities
- 8.3. Visualizations and interpretations
- References
- Chapter 9: Multivariate density estimation
- Abstract
- 9.1. Introduction
- 9.2. Description of the estimator
- 9.3. Asymptotic theory
- 9.4. Bandwidth selection
- 9.5. An example
- 9.6. Investigating performance in the multivariate case
- 9.7. A more flexible version of the LGDE
- 9.8. Proofs
- References
- Chapter 10: Conditional density estimation
- Abstract
- 10.1. Introduction
- 10.2. Estimating the conditional density
- 10.3. Asymptotic theory for dependent data
- 10.4. Examples
- 10.5. Proof of theorems
- References
- Chapter 11: The local Gaussian partial correlation
- Abstract
- 11.1. Introduction
- 11.2. The local Gaussian partial correlation
- 11.3. Properties
- 11.4. Estimation of the LGPC by local likelihood
- 11.5. Asymptotic theory
- 11.6. Examples
- 11.7. Testing for conditional independence
- 11.8. The multivariate LGPC
- References
- Chapter 12: Regression and conditional regression quantiles
- Abstract
- 12.1. Introduction
- 12.2. Comparison with additive regression modeling
- 12.3. Local Gaussian regression estimation
- 12.4. Asymptotic normality
- 12.5. Example
- 12.6. Conditional quantiles
- 12.7. Proof
- References
- Chapter 13: A local Gaussian Fisher discriminant
- Abstract
- 13.1. Introduction
- 13.2. A local Gaussian Fisher discriminant
- 13.3. Some asymptotics of Bayes risk
- 13.4. Choice of bandwidth
- 13.5. Illustrations
- 13.6. Summary remark
- References
- Author index
- Subject index
- Edition: 1
- Published: October 5, 2021
- No. of pages (Paperback): 458
- No. of pages (eBook): 458
- Imprint: Academic Press
- Language: English
- Paperback ISBN: 9780128158616
- eBook ISBN: 9780128154458
DT
Dag Tjøstheim
HO
Håkon Otneim
BS