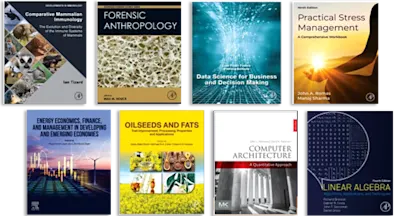
Statistical Modeling in Machine Learning
Concepts and Applications
- 1st Edition - November 7, 2022
- Imprint: Academic Press
- Editors: Tilottama Goswami, G. R. Sinha
- Language: English
- Paperback ISBN:9 7 8 - 0 - 3 2 3 - 9 1 7 7 6 - 6
- eBook ISBN:9 7 8 - 0 - 3 2 3 - 9 7 2 5 2 - 9
Statistical Modeling in Machine Learning: Concepts and Applications presents the basic concepts and roles of statistics, exploratory data analysis and machine learning. The vario… Read more
Purchase options
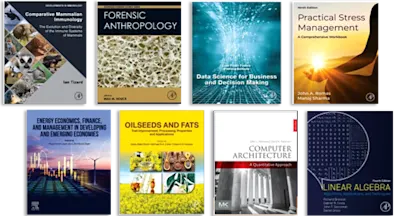
Statistical Modeling in Machine Learning: Concepts and Applications presents the basic concepts and roles of statistics, exploratory data analysis and machine learning. The various aspects of Machine Learning are discussed along with basics of statistics. Concepts are presented with simple examples and graphical representation for better understanding of techniques. This book takes a holistic approach – putting key concepts together with an in-depth treatise on multi-disciplinary applications of machine learning. New case studies and research problem statements are discussed, which will help researchers in their application areas based on the concepts of statistics and machine learning.
Statistical Modeling in Machine Learning: Concepts and Applications will help statisticians, machine learning practitioners and programmers solving various tasks such as classification, regression, clustering, forecasting, recommending and more.
- Provides a comprehensive overview of the state-of-the-art in statistical concepts applied to Machine Learning with the help of real-life problems, applications and tutorials
- Presents a step-by-step approach from fundamentals to advanced techniques
- Includes Case Studies with both successful and unsuccessful applications of Machine Learning to understand challenges in its implementation, along with worked examples
1. Introduction to Statistical Modelling in Machine Learning - A Case Study
2. A Technique of Data Collection- Web Scraping with Python
3. Analysis of Covid-19 using Machine Learning Techniques
4. Discriminative Dictionary Learning based on Statistical Methods
5. Artificial Intelligence based Uncertainty Quantification technique for External flow CFD simulations
6. Music Genres Classification
7. Classification Model of Machine Learning for Medical Data Analysis
8. Regression Models for Machine learning
9. Model Selection and Regularization
10. Data Clustering using Unsupervised Machine Learning
11. Emotion-based classification through fuzzy entropy enhanced FCM clustering
12. Fundamental Optimization Methods for Machine Learning
13. Stochastic Optimization of Industrial Grinding Operation through Data-Driven Robust Optimization
14. Dimensionality Reduction using PCAs in Feature Partitioning Framework
15. Impact of Mid-Day Meal Scheme in Primary Schools in India using Exploratory Data Analysis and Data Visualisation
16. Nonlinear System Identification of Environmental pollutants using Recurrent Neural Networks and Global Sensitivity Analysis
17. Comparative Study of Automated Deep Learning Techniques for Wind Time Series Forecasting
- Edition: 1
- Published: November 7, 2022
- Imprint: Academic Press
- Language: English
TG
Tilottama Goswami
GS