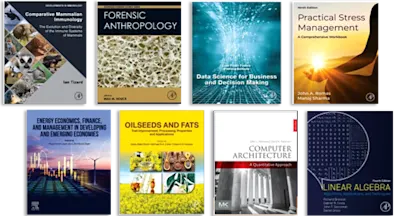
State of the Art in Neural Networks and Their Applications
Volume 2
- 1st Edition - December 2, 2022
- Imprint: Academic Press
- Editors: Jasjit S. Suri, Ayman S. El-Baz
- Language: English
- Paperback ISBN:9 7 8 - 0 - 1 2 - 8 1 9 8 7 2 - 8
- eBook ISBN:9 7 8 - 0 - 1 2 - 8 1 9 9 1 2 - 1
State of the Art in Neural Networks and Their Applications, Volume Two presents the latest advances in artificial neural networks and their applications across a wide range of… Read more
Purchase options
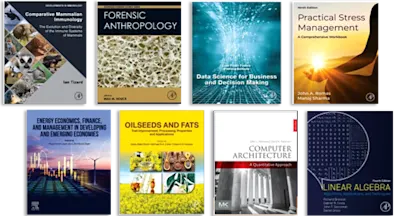
State of the Art in Neural Networks and Their Applications, Volume Two presents the latest advances in artificial neural networks and their applications across a wide range of clinical diagnoses. The book provides over views and case studies of advances in the role of machine learning, artificial intelligence, deep learning, cognitive image processing, and suitable data analytics useful for clinical diagnosis and research applications. The application of neural network, artificial intelligence and machine learning methods in biomedical image analysis have resulted in the development of computer-aided diagnostic (CAD) systems that aim towards the automatic early detection of several severe diseases.
State of the Art in Neural Networks and Their Applications is presented in two volumes. Volume One: Neural Networks in Oncology Imaging covers lung cancer, prostate cancer, and bladder cancer. Volume Two: Neural Networks in Brain Disorders and Other Diseases covers autism spectrum disorder, Alzheimer’s disease, attention deficit hyperactivity disorder, hypertension, and other diseases. Written by experienced engineers in the field, these two volumes will help engineers, computer scientists, researchers, and clinicians understand the technology and applications of artificial neural networks.
- Includes applications of neural networks, AI, machine learning, and deep learning techniques to a variety of oncology imaging technologies
- Provides in-depth technical coverage of computer-aided diagnosis (CAD), including coverage of computer-aided classification, unified deep learning frameworks, 3D MRI, PET/CT, and more
- Covers deep learning cancer identification from histopathological images, medical image analysis, detection, segmentation and classification via AI
2. Computational Applications in Brain and Breast Cancer
3. Deep Neural Networks and Advanced Computer Vision Algorithms in The Early Diagnosis of Skin Diseases
4. An Accurate Deep Learning-Based CAD System For Early Diagnosis Of Prostate Cancer
5. Adaptive Graph Convolutional Neural Network and its Biomedical Applications
6. Deep Slice Interpolation via Marginal Super-Resolution, Fusion and Refinement
7. New Explainable Deep CNN Design for Classifying Breast Tumor Response over Neoadjuvant Chemotherapy
8. Deep Learning Interpretability: Measuring The Relevance of Clinical Concepts in CNN Features
9. Computational Lung Sound Classification: A Review
10. Clinical Applications of Machine Learning in Heart Failure
11. Role of AI and Radiomics in Diagnosing Renal Tumors: A Survey
12. Texture-Centric Diagnostic Models for Thyroid-Cancer Using Convolutional Neural Networks: Bridging the Gap Between Radiomics and Microscopic Domains
- Edition: 1
- Published: December 2, 2022
- Imprint: Academic Press
- Language: English
JS
Jasjit S. Suri
Dr. Jasjit Suri, PhD, MBA, is a renowned innovator and scientist. He received the Director General’s Gold Medal in 1980 and is a Fellow of several prestigious organizations, including the American Institute of Medical and Biological Engineering and the Institute of Electrical and Electronics Engineers. Dr. Suri has been honored with lifetime achievement awards from Marcus, NJ, USA, and Graphics Era University, India. He has published nearly 300 peer-reviewed AI articles, 100 books, and holds 100 innovations/trademarks, achieving an H-index of nearly 100 with about 43,000 citations. Dr. Suri has served as chairman of AtheroPoint, IEEE Denver section, and as an advisory board member to various healthcare industries and universities globally.
AS