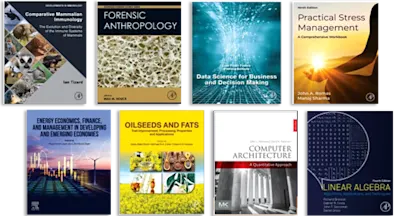
State of the Art in Neural Networks and Their Applications
Volume 1
- 1st Edition - July 26, 2021
- Imprint: Academic Press
- Editors: Ayman S. El-Baz, Jasjit S. Suri
- Language: English
- Paperback ISBN:9 7 8 - 0 - 1 2 - 8 1 9 7 4 0 - 0
- eBook ISBN:9 7 8 - 0 - 1 2 - 8 2 1 8 4 9 - 5
State of the Art in Neural Networks and Their Applications presents the latest advances in artificial neural networks and their applications across a wide range of clinical diagno… Read more
Purchase options
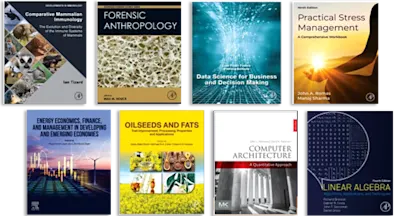
State of the Art in Neural Networks and Their Applications is presented in two volumes. Volume 1 covers the state-of-the-art deep learning approaches for the detection of renal, retinal, breast, skin, and dental abnormalities and more.
- Includes applications of neural networks, AI, machine learning, and deep learning techniques to a variety of imaging technologies
- Provides in-depth technical coverage of computer-aided diagnosis (CAD), with coverage of computer-aided classification, Unified Deep Learning Frameworks, mammography, fundus imaging, optical coherence tomography, cryo-electron tomography, 3D MRI, CT, and more
- Covers deep learning for several medical conditions including renal, retinal, breast, skin, and dental abnormalities, Medical Image Analysis, as well as detection, segmentation, and classification via AI
2. Detection of retinal abnormalities in fundus image using CNN Deep Learning Networks
3. A survey of Deep Learning Based Methods for Cryo-electron Tomography Data Analysis
4. Detection, Segmentation and Numbering of Teeth in Dental Panoramic Images with Mask RCNN
5. Accurate Identification of Renal Transplant Rejection: Convolutional Neural Networks and Diffusion MRI
6. Applications of the ESPNet Architecture in Medical Imaging
7. Achievements of Neural Network in Skin Lesions Classification
8. A Computer-aided-diagnosis System for Breast Cancer Molecular Subtypes Prediction in mammographic images
9. Computer-Aided Diagnosis of Renal Masses
10. Early Identification of Acute Rejection for Renal Allografts: A Machine Learning Approach
11. Deep Learning for Computer-Aided Diagnosis in Ophthalmology: A Review
12. Deep Learning for Ophthalmology using Optical Coherence Tomography
13. Generative Adversarial Networks in Medical Imaging
14. Deep Learning from Small Labeled Datasets Applied to Medical Image Analysis
- Edition: 1
- Published: July 26, 2021
- Imprint: Academic Press
- Language: English
AS
Ayman S. El-Baz
JS
Jasjit S. Suri
Dr. Jasjit Suri, PhD, MBA, is a renowned innovator and scientist. He received the Director General’s Gold Medal in 1980 and is a Fellow of several prestigious organizations, including the American Institute of Medical and Biological Engineering and the Institute of Electrical and Electronics Engineers. Dr. Suri has been honored with lifetime achievement awards from Marcus, NJ, USA, and Graphics Era University, India. He has published nearly 300 peer-reviewed AI articles, 100 books, and holds 100 innovations/trademarks, achieving an H-index of nearly 100 with about 43,000 citations. Dr. Suri has served as chairman of AtheroPoint, IEEE Denver section, and as an advisory board member to various healthcare industries and universities globally.