State Estimation Strategies in Lithium-ion Battery Management Systems
- 1st Edition - July 14, 2023
- Authors: Kailong Liu, Yujie Wang, Daniel-Ioan Stroe, Carlos Fernandez, Josep M. Guerrero, Shunli Wang
- Language: English
- Paperback ISBN:9 7 8 - 0 - 4 4 3 - 1 6 1 6 0 - 5
- eBook ISBN:9 7 8 - 0 - 4 4 3 - 1 6 1 6 1 - 2
State Estimation Strategies in Lithium-ion Battery Management Systems presents key technologies and methodologies in modeling and monitoring charge, energy, power and health of… Read more
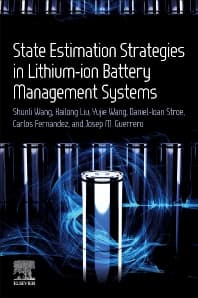
Purchase options
Institutional subscription on ScienceDirect
Request a sales quoteState Estimation Strategies in Lithium-ion Battery Management Systems presents key technologies and methodologies in modeling and monitoring charge, energy, power and health of lithium-ion batteries. Sections introduce core state parameters of the lithium-ion battery, reviewing existing research and the significance of the prediction of core state parameters of the lithium-ion battery and analyzing the advantages and disadvantages of prediction methods of core state parameters. Characteristic analysis and aging characteristics are then discussed. Subsequent chapters elaborate, in detail, on modeling and parameter identification methods and advanced estimation techniques in different application scenarios.
Offering a systematic approach supported by examples, process diagrams, flowcharts, algorithms, and other visual elements, this book is of interest to researchers, advanced students and scientists in energy storage, control, automation, electrical engineering, power systems, materials science and chemical engineering, as well as to engineers, R&D professionals, and other industry personnel.
- Introduces lithium-ion batteries, characteristics and core state parameters
- Examines battery equivalent modeling and provides advanced methods for battery state estimation
- Analyzes current technology and future opportunities
2. Characteristic analysis of power lithium-ion batteries
3. Aging characteristics of lithium-ion batteries
4. Lithium-ion battery hysteresis characteristics and modeling
5. Lithium-ion battery aging mechanism and multiple regression model
6. Equivalent modeling and parameter identification of power lithium-ion batteries
7. Equivalent modeling study of aviation lithium-ion batteries
8. Battery SOC measurement and control model based on Internet platforms
9. High energy density lithium-ion battery SOC prognosis
10. SOC estimation strategy based on fractional-order model
11. SOC estimation method for large unmanned aerial vehicles
12. Construction of SOC estimation method for automotive ternary batteries
13. Estimation strategies for SOC and SOP of lithium-ion batteries
14. Collaborative energy and peak power status estimation
15. SOH estimation based on improved double-extended Kalman filter
16. Collaborative SOC and SOH estimation based on improved AUKF-UPF algorithm
- No. of pages: 376
- Language: English
- Edition: 1
- Published: July 14, 2023
- Imprint: Elsevier
- Paperback ISBN: 9780443161605
- eBook ISBN: 9780443161612
KL
Kailong Liu
YW
Yujie Wang
DS
Daniel-Ioan Stroe
CF
Carlos Fernandez
JG
Josep M. Guerrero
SW