Spatial Autocorrelation
A Fundamental Property of Geospatial Sciences
- 1st Edition - June 1, 2025
- Authors: Daniel Griffith, Bin Li
- Language: English
- Paperback ISBN:9 7 8 - 0 - 4 4 3 - 4 1 7 4 3 - 6
- eBook ISBN:9 7 8 - 0 - 4 4 3 - 4 1 7 4 4 - 3
Spatial Autocorrelation: A Fundamental Property of Geospatial Sciences offers a comprehensive exploration of one of the most critical concepts in spatial analysis. Beginning with f… Read more
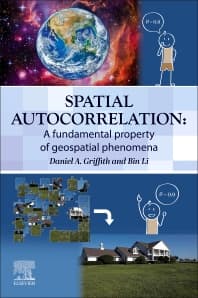
Purchase options
Institutional subscription on ScienceDirect
Request a sales quoteSpatial Autocorrelation: A Fundamental Property of Geospatial Sciences offers a comprehensive exploration of one of the most critical concepts in spatial analysis. Beginning with foundational theories and clear definitions, this book thoroughly sets out the concepts and theory of spatial autocorrelation through detailed conceptualisation and practical examples. The detailed case studies illustrate the pervasive influence of spatial patterns in scientific inquiry, with an eye toward future research and innovative techniques. It provides practical methodologies for quantifying spatial autocorrelation, complete with step-by-step guidance and real-world applications. Spatial Autocorrelation equips graduate students, researchers, and professionals with the knowledge and tools to confidently navigate and apply spatial analysis in their respective domains, making it a vital addition to a number of disciplines that utilise spatial analysis.
- Explores a fundamental geospatial concept through a blend of multidisciplinary topics
- Provides an educational focus on spatial autocorrelation in a conceptually-friendly manner
- Offers a progressive, iterative layout spanning basic to advanced concepts
- Emphasizes novel as well as benchmark empirical examples and simulation experiments
- Covers in-depth spatial autocorrelation in various disciplines, being nascent in some of them
Geospatial students, scientists and professionals, as well as any scholars involved in spatial autocorrelation as a fundamental property of georeferenced data
TABLE OF CONTENTS
Forward by J. Keith Ord
Prologue
TOC
Chapter 1 What is spatial autocorrelation? A conceptualization
1.1. The Jigsaw puzzle metaphor: an accessible pedagogic tool
1.2. A formal definition and meaning of SA
1.2.1. SWM specifications
1.2.2. SWM eigenfunctions
1.3. Zero SA: ZSA
1.4. Positive SA: PSA
1.5. Negative SA: NSA
1.6. PSA-NSA mixtures: an innovative perspective
1.7. Chapter 1 dénouement
References
Chapter 2 SA is everywhere!
2.1. Beyond the jigsaw puzzle: other imperfect SA metaphors
2.1.1. A slider puzzle metaphor
2.1.2. A magnetic desk sculpture metaphor
2.2. SA in the popular media: common and widespread occurrences
2.2.1. Cartoon and comic portrayals of SA
2.2.2. Public press editorials and essays about SA
2.3. SA in nature: spatially correlated phenomena in the wild
2.3.1. Synchronous fireflies: direct SA
2.3.2. Discovering a new firefly genus: SA in action
2.3.3. Bandit bumblebee behavior: differential SA across geographic scales
2.4. SA in academia: the natural and social sciences
2.5. SA in the medical sciences: epidemiology and beyond
2.5.1. Epidemiology
2.5.2. Visualizing the invisible: picturing anatomy and physiology shrouded by skin and bones
2.6. SA in engineering: integrating technology and self-correlation
2.7. SA in real estate: everyday spatial dependencies―doing the awkward math!
2.8. SA in art and music: a cultural emergence
2.8.1. Art: a humanities-quantitative geography/GIScience-mathematics intersection
2.8.2. Music: the final SA frontier?
2.9. SA implicit in government regulations: unlikely partners
2.10. Geography: the foundation of SA
2.11. Urban planning: a natural SA receptacle
2.12. Chapter 2 dénouement
References
Chapter 3 Quantifying spatial autocorrelation: an intuitive approach with few equations
3.1. From Pearson correlation to SA
3.1.1. Equi-correlation theory and practice
3.1.2. From the equi-correlation matrix to SWMs
3.1.3. Inferential interpretations of SA
3.1.4. Substantive interpretations of SA
3.2. Formal quantifications of SA: MC, GR, join counts, and their interrelationships
3.3. SWM eigenfunctions: a deeper dive
3.3.1. Eigenfunctions in a nutshell
3.3.2. SWM eigenfunctions: a primer
3.3.3. A degenerate case: the geographic distribution of a constant c
3.4. Semivariogram models: an elementary overview
3.5. Local SA statistics: an introduction
3.5.1. LISA and PSA in Puerto Rico
3.5.2. NSA clusters in Puerto Rico
3.6. Chapter 3 dénouement
References
Chapter 4 Reflections on SA model specifications for beginners
4.1. Revisiting SWM and geostatistical model specifications
4.2. A critical review of auto-model specifications
4.3. Sui- models: a new mechanism for embedding SA in probability functions
4.3.1. Simulating spatially autocorrelated sui- RVs
4.3.1.1. Preparations for simulating Puerto Rican sui- RVs
4.3.1.2. Visualizing simulated georeferenced data for Puerto Rico: A proof of concept
4.3.2. Impacts of SA on RV frequency distributions
4.3.3. Leveraging SA in sui- RVs
4.4. Chapter 4 dénouement
Appendix 4-A Implicit overdispersion in Bernoulli RVs
References
Chapter 5 Geographic distributions: univariate SA
5.1. SA: catalyzing geography’s first quantitative revolution
5.2. Normal versus non-normal georeferenced data: assessing the validity of assumptions
5.3. Variance in georeferenced RVs: homogeneity, stationarity, and stability
5.3.1. Variance homogeneity
5.3.2. Variance stationarity
5.3.3. Variance stability and theoretical dispersion
5.4. Heterogeneous SA: from global patterns to local clusters
5.5. Impacts of SA on RV properties
5.6. Chapter 5 dénouement
References
Chapter 6 Areal associations: multivariate SA
6.1. SA and covarying geographic distributions
6.2. SA, multicollinearity, and dimensions of geographic distributions: PCA and FA
6.3. SA, multicollinearity, and areal differentiation: MANOVA and DFA
6.3.1. Municipios as regions: DFA findings
6.3.2. SA and hierarchical geographic configurations
6.4. SA and geographic gradients: CC
6.4.1. CC with SA
6.4.2. CC capturing SA
6.4.3. CC without SA
6.5. SA and regionalization: CA
6.5.1. Deriving not necessarily contiguous Puerto Rican regions using coordinates
6.5.2. Deriving contiguous Puerto Rican regions using spectral indices
6.6. Chapter 6 dénouement
Appendix 6A A MESF linear correlation coefficient decomposition example
Appendix 6B Evaluating an enhanced vegetation spectral index (EVI)
Appendix 6C SAR estimation with the rook’s adjacency definition
References
Chapter 7 SA and spatial interaction
7.1. Transitioning from inertia to movement in geospatial academic studies
7.2. A brief historical overview
7.3. A meta-analysis of SA in journey-to-work flows
7.4. An empirical example: Chicago journey-to-work flows
7.5. Pathways from intractable to tractable solutions
7.6. Chapter 7 dénouement
Appendix 7A Tallies of 2020 census tracts for the largest 36 MSAs
References
Chapter 8 Some SA final frontiers: a partial future research agenda
8.1. SA and art
8.1.1. SA components for regular square tessellations with a rook adjacency definition
8.1.2. Rosmarin’s paintings: hiding in “plane” sight
8.1.3. Art from space: from science to aesthetics
8.1.4. Showcasing world-class artistic talent
8.1.5. A meta-analysis of imagery
8.2. SA and spatial optimization
8.2.1. The p = 1 median problem and SA: what we know to date
8.2.2. The p = 2 median problem and SA: a cursory meta-analysis
8.2.3. P > 2 median problems, SA, and tessellation stratified random sampling
8.3. Chapter 8 dénouement and consolidated research agenda
References
Chapter 9 Summary and concluding remarks
9.1. Conceptual foundation constituents
9.1.1. Fundamentals of concept formation in the geospatial sciences
9.1.1.1. Variance inflation
9.1.1.2. SA is everywhere
9.1.2. SA indices
9.1.3. Sui- RVs
9.1.4. Univariate and multivariate spatial analysis
9.1.5. Spatial interaction
9.1.6. SA, art, and spatial optimization: promulgating the SA realm
9.1.7. SA and artificial intelligence
9.2. Conclusions
9.3. Chapter 9 dénouement
References
Epilogue
Spatial autocorrelation glossary
Forward by J. Keith Ord
Prologue
TOC
Chapter 1 What is spatial autocorrelation? A conceptualization
1.1. The Jigsaw puzzle metaphor: an accessible pedagogic tool
1.2. A formal definition and meaning of SA
1.2.1. SWM specifications
1.2.2. SWM eigenfunctions
1.3. Zero SA: ZSA
1.4. Positive SA: PSA
1.5. Negative SA: NSA
1.6. PSA-NSA mixtures: an innovative perspective
1.7. Chapter 1 dénouement
References
Chapter 2 SA is everywhere!
2.1. Beyond the jigsaw puzzle: other imperfect SA metaphors
2.1.1. A slider puzzle metaphor
2.1.2. A magnetic desk sculpture metaphor
2.2. SA in the popular media: common and widespread occurrences
2.2.1. Cartoon and comic portrayals of SA
2.2.2. Public press editorials and essays about SA
2.3. SA in nature: spatially correlated phenomena in the wild
2.3.1. Synchronous fireflies: direct SA
2.3.2. Discovering a new firefly genus: SA in action
2.3.3. Bandit bumblebee behavior: differential SA across geographic scales
2.4. SA in academia: the natural and social sciences
2.5. SA in the medical sciences: epidemiology and beyond
2.5.1. Epidemiology
2.5.2. Visualizing the invisible: picturing anatomy and physiology shrouded by skin and bones
2.6. SA in engineering: integrating technology and self-correlation
2.7. SA in real estate: everyday spatial dependencies―doing the awkward math!
2.8. SA in art and music: a cultural emergence
2.8.1. Art: a humanities-quantitative geography/GIScience-mathematics intersection
2.8.2. Music: the final SA frontier?
2.9. SA implicit in government regulations: unlikely partners
2.10. Geography: the foundation of SA
2.11. Urban planning: a natural SA receptacle
2.12. Chapter 2 dénouement
References
Chapter 3 Quantifying spatial autocorrelation: an intuitive approach with few equations
3.1. From Pearson correlation to SA
3.1.1. Equi-correlation theory and practice
3.1.2. From the equi-correlation matrix to SWMs
3.1.3. Inferential interpretations of SA
3.1.4. Substantive interpretations of SA
3.2. Formal quantifications of SA: MC, GR, join counts, and their interrelationships
3.3. SWM eigenfunctions: a deeper dive
3.3.1. Eigenfunctions in a nutshell
3.3.2. SWM eigenfunctions: a primer
3.3.3. A degenerate case: the geographic distribution of a constant c
3.4. Semivariogram models: an elementary overview
3.5. Local SA statistics: an introduction
3.5.1. LISA and PSA in Puerto Rico
3.5.2. NSA clusters in Puerto Rico
3.6. Chapter 3 dénouement
References
Chapter 4 Reflections on SA model specifications for beginners
4.1. Revisiting SWM and geostatistical model specifications
4.2. A critical review of auto-model specifications
4.3. Sui- models: a new mechanism for embedding SA in probability functions
4.3.1. Simulating spatially autocorrelated sui- RVs
4.3.1.1. Preparations for simulating Puerto Rican sui- RVs
4.3.1.2. Visualizing simulated georeferenced data for Puerto Rico: A proof of concept
4.3.2. Impacts of SA on RV frequency distributions
4.3.3. Leveraging SA in sui- RVs
4.4. Chapter 4 dénouement
Appendix 4-A Implicit overdispersion in Bernoulli RVs
References
Chapter 5 Geographic distributions: univariate SA
5.1. SA: catalyzing geography’s first quantitative revolution
5.2. Normal versus non-normal georeferenced data: assessing the validity of assumptions
5.3. Variance in georeferenced RVs: homogeneity, stationarity, and stability
5.3.1. Variance homogeneity
5.3.2. Variance stationarity
5.3.3. Variance stability and theoretical dispersion
5.4. Heterogeneous SA: from global patterns to local clusters
5.5. Impacts of SA on RV properties
5.6. Chapter 5 dénouement
References
Chapter 6 Areal associations: multivariate SA
6.1. SA and covarying geographic distributions
6.2. SA, multicollinearity, and dimensions of geographic distributions: PCA and FA
6.3. SA, multicollinearity, and areal differentiation: MANOVA and DFA
6.3.1. Municipios as regions: DFA findings
6.3.2. SA and hierarchical geographic configurations
6.4. SA and geographic gradients: CC
6.4.1. CC with SA
6.4.2. CC capturing SA
6.4.3. CC without SA
6.5. SA and regionalization: CA
6.5.1. Deriving not necessarily contiguous Puerto Rican regions using coordinates
6.5.2. Deriving contiguous Puerto Rican regions using spectral indices
6.6. Chapter 6 dénouement
Appendix 6A A MESF linear correlation coefficient decomposition example
Appendix 6B Evaluating an enhanced vegetation spectral index (EVI)
Appendix 6C SAR estimation with the rook’s adjacency definition
References
Chapter 7 SA and spatial interaction
7.1. Transitioning from inertia to movement in geospatial academic studies
7.2. A brief historical overview
7.3. A meta-analysis of SA in journey-to-work flows
7.4. An empirical example: Chicago journey-to-work flows
7.5. Pathways from intractable to tractable solutions
7.6. Chapter 7 dénouement
Appendix 7A Tallies of 2020 census tracts for the largest 36 MSAs
References
Chapter 8 Some SA final frontiers: a partial future research agenda
8.1. SA and art
8.1.1. SA components for regular square tessellations with a rook adjacency definition
8.1.2. Rosmarin’s paintings: hiding in “plane” sight
8.1.3. Art from space: from science to aesthetics
8.1.4. Showcasing world-class artistic talent
8.1.5. A meta-analysis of imagery
8.2. SA and spatial optimization
8.2.1. The p = 1 median problem and SA: what we know to date
8.2.2. The p = 2 median problem and SA: a cursory meta-analysis
8.2.3. P > 2 median problems, SA, and tessellation stratified random sampling
8.3. Chapter 8 dénouement and consolidated research agenda
References
Chapter 9 Summary and concluding remarks
9.1. Conceptual foundation constituents
9.1.1. Fundamentals of concept formation in the geospatial sciences
9.1.1.1. Variance inflation
9.1.1.2. SA is everywhere
9.1.2. SA indices
9.1.3. Sui- RVs
9.1.4. Univariate and multivariate spatial analysis
9.1.5. Spatial interaction
9.1.6. SA, art, and spatial optimization: promulgating the SA realm
9.1.7. SA and artificial intelligence
9.2. Conclusions
9.3. Chapter 9 dénouement
References
Epilogue
Spatial autocorrelation glossary
- No. of pages: 340
- Language: English
- Edition: 1
- Published: June 1, 2025
- Imprint: Elsevier
- Paperback ISBN: 9780443417436
- eBook ISBN: 9780443417443
DG
Daniel Griffith
Daniel A. Griffith is an Ashbel Smith Professor of Geospatial Information Sciences at the University of Texas at Dallas, affiliated professor in the College of Public Health at the University of South Florida, and adjunct professor in the Department of Resource Economics and Environmental Sociology at the University of Alberta. He holds degrees in Mathematics, Statistics, and Geography, and arguably is the inventor of Moran eigenvector spatial filtering. He is a two-time Fulbright Senior Specialist, an AAG Distinguished Research Honors awardee, and an elected fellow of the Royal Society of Canada, UCGIS, AAG, American Association for the Advancement of Science, American Statistical Association, Regional Science Association International, and Spatial Econometrics Association.
Affiliations and expertise
Professor of Geospatial Information Sciences, University of Texas at Dallas, Texas, USABL
Bin Li
Dr Li is a Professor at Central Michigan U. in the US, where he was the former chair of the Department of Geography and Environmental Studies. His previous position was at U. of Miami. He specializes in Geographic Information Science with research and teaching experiences in Spatial Statistics, Geographic Information Services, and Cartography. His recent journal publications and presentations focus on information redundancy in big data, visualization of spatial structures, and regression modeling with large spatial data sets. He authored three books on spatial statistics, and edited several books in GIScience. He serves on editorial boards of several academic journals, including the Annals of AAG and Geospatial Information Science.
Affiliations and expertise
Louisiana State University, USA