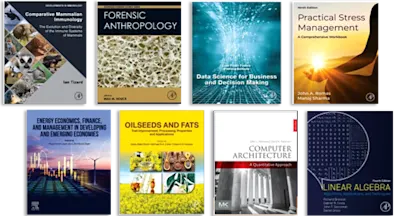
Soft Computing in Smart Manufacturing and Materials
- 1st Edition - January 22, 2025
- Imprint: Elsevier
- Editors: Sudan Jha, Shubhabrata Datta, Deepak Prashar, Sarbagya Ratna Shakya, Valentina Emilia Balas
- Language: English
- Paperback ISBN:9 7 8 - 0 - 4 4 3 - 2 9 9 2 7 - 8
- eBook ISBN:9 7 8 - 0 - 4 4 3 - 2 9 9 2 8 - 5
Soft Computing in Smart Manufacturing and Materials explains the role of soft computing in the manufacturing industries. It presents the techniques, concepts and design princi… Read more
Purchase options
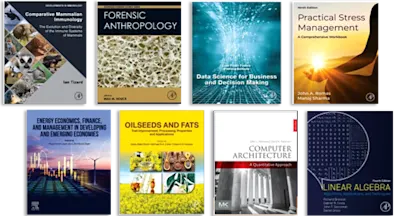
- Introduces soft computing techniques for the creation of sustainable solutions for smart materials and manufacturing
- Offers perspectives for design, development, and commissioning of intelligent applications
- Reviews the latest intelligent technologies and algorithms related to monitoring and mitigation of sustainable engineering
- Discusses the implementation of soft computing in the various areas of engineering materials
- Looks at future sustainable and intelligent monitoring techniques that will benefit manufacturing
1.1 Constituents of Soft Computing
1.2 Introduction to Swarm Intelligence
2: Design principles for Smart Soft Computing
3: Components for Smart Materials
4: Characterization of Advanced Smart Materials
4.1 Computational modelling of Advanced Materials
4.2 Optimization of controlling Parameters
4.3 Tribology of Advanced Materials
5: Role of Soft Computing in Manufacturing Industries
5.1 Self-Healing Materials in Biomedical Device
5.2 Personal Protective Equipment (PPE) in Healthcare Industry
5.3 Sensors and Actuators in Agriculture Industry
6: Applied Soft Computing Techniques in Manufacturing Process
6.1 Evolutionary Algorithm in Advanced Manufacturing processes
6.2 Optimizing Welding parameters by Fuzzy logic
6.3 Genetic Rule based algorithms in Additive Manufacturing
6.4 Cost Prediction for Fabrication of Materials by Artificial Neural Network
7: Artificial Intelligence in Industrial Application
7:1: Route path planning in Production Assembly
7.2: Transportation Problem
7:3: Implementation of AI in Industry 4.0
7:4: Optimizing Supply chain network by AI
8: Soft Computing and System Engineering
9: Manufacturing Optimization Prediction by Artificial Neural Network
10: Testing and Analysis correlation by Genetic Algorithms
11: Product Life Cycle Assessment
12: Recent Trends and Innovation in Soft Computing/Manufacturing
- Edition: 1
- Published: January 22, 2025
- Imprint: Elsevier
- Language: English
SJ
Sudan Jha
SD
Shubhabrata Datta
Dr. Shubhabrata Datta is a Research Professor in Mechanical Engineering of SRM Institute of Science and Technology, Kattanku lathur, Chennai, India. His research interest is in the domain of design of materials using artificial intelligence and machine learning techniques. Dr. Datta has more than 150 publications in journals and peer-reviewed conference proceedings. He was bestowed with the Exchange Scientist Award from Royal Academy of Engineering, UK and worked in the University of Sheffield, UK. He also worked in Dept of Materials Science and Engineering, Helsinki University of Technology, Finland, Dept of Materials Science and Engineering, Iowa State University, Ames, USA and Heat Engineering Lab, Dept of Chemical Engineering, Åbo Akademi University, Finland as Visiting Scientist. He is a Fellow of Institution of Engineers (India), Associate Editor, Journal of the Institution of Engineers (India): Series D, and editorial board member of several international journals.
DP
Deepak Prashar
Deepak Prashar received B. Tech in Computer Science and Engineering from Punjab Technical University, Pun- jab, India in 2007 and M. Tech. in Computer Science and Engineering from PEC University of Technology, Chandigarh, India in 2009. Presently he is working as a Professor and Coordinator of School of Computer Science & Engineering at Lovely Professional University, Punjab, India since 2009. He has completed his Ph. D. degree from Punjab Technical University (Punjab). He has published more than 90 research papers in reputed national, international conferences and journals, including SCOPUS and SCIE indexed journals. His research interest includes Wireless Sensor Networks, Soft Computing, Block chain, Machine Learning, IoT, Image Processing and Cyber security. He is involved in the review process of many SCI or SCIE indexed journals like IEEE, Springer, Wiley, Inderscience, etc. He is also the Editorial Board member of recognized journals and serving as a technical program committee member in reputed international conferences in India and abroad.
SS
Sarbagya Ratna Shakya
Sarbagya Ratna Shakya is working as an Assistant Professor at Eastern New Mexico University, New Mexico, USA. He received the B. Eng. in Electronics Engineering from National College of Engineering, Tribhuvan University of Nepal in 2009; M. Eng. in Computer Engineering from Nepal College of Information Technology, Pokhara University of Nepal in 2014. He has received his Ph. D in Computational Science (Computer Science) from the School of Computer Science and Computer Engineering, University of Southern Mississippi, USA. He has more than 10 years of teaching experience in undergraduate level courses. His research interest includes machine learning, deep learning, image processing, Internet of things and has published journals papers, conference papers, and book chapters in different domain on applications of machine learning and deep learning.
VE