Smart Metro Station Systems
Data Science and Engineering
- 1st Edition - January 4, 2022
- Authors: Hui Liu, Chao Chen, Yanfei Li, Zhu Duan, Ye Li
- Language: English
- Paperback ISBN:9 7 8 - 0 - 3 2 3 - 9 0 5 8 8 - 6
- eBook ISBN:9 7 8 - 0 - 3 2 3 - 9 0 7 1 2 - 5
Smart Metro Station Systems: Data Science and Engineering introduces key technologies in data science and engineering for smart metro station systems. The book consists of three… Read more
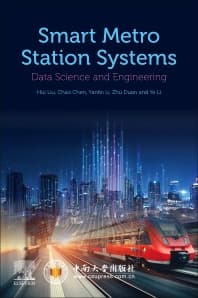
Purchase options
Institutional subscription on ScienceDirect
Request a sales quoteSmart Metro Station Systems: Data Science and Engineering introduces key technologies in data science and engineering for smart metro station systems. The book consists of three main parts, focusing on the environment, people and energy. Each chapter includes practical applications, along with information on metro traffic flow monitoring and passenger guidance, methods for behavior analysis and trajectory projection, clustering and anomaly detection in crowd hotspots, monitoring and prediction for station humidity, monitoring and spatial prediction for air pollutants, time series feature extraction and analysis of metro load, characteristic and correlation analysis of metro load, and prediction and intelligent ventilation control.
This volume offers a key reference on the emerging area of smart metro stations and will be useful to those working on smart railways, data science, engineering, artificial intelligence and aligned fields.
- Presents relevant core technologies of data science and engineering in smart metro station systems
- Describes systems based on holographic perception, terminal platform control and highly-autonomous operation
- Gives a large number of practical case studies and experimental designs
- Introduces state-of-the-art machine learning and data mining methods for smart metro station systems
- Offers a comprehensive, up-to-date research solution for the emerging area of smart metro stations
- Cover image
- Title page
- Table of Contents
- Copyright
- Preface
- Acknowledgments
- Chapter 1: Introduction
- Abstract
- 1.1: Overview of data science and engineering
- 1.2: Framework of smart metro station systems
- 1.3: Human and smart metro station systems
- 1.4: Environment and smart metro station systems
- 1.5: Energy and smart metro station systems
- 1.6: Scope of this book
- References
- Chapter 2: Metro traffic flow monitoring and passenger guidance
- Abstract
- 2.1: Introduction
- 2.2: Description of metro traffic flow data
- 2.3: Prediction of metro traffic flow based on Elman neural network
- 2.4: Prediction of metro traffic flow based on the deep echo state network
- 2.5: Passenger guidance strategy based on prediction results
- 2.6: Conclusions
- References
- Chapter 3: Individual behavior analysis and trajectory prediction
- Abstract
- 3.1: Introduction
- 3.2: Description of individual GPS data
- 3.3: Preprocessing of individual GPS data
- 3.4: Prediction of GPS trajectory based on optimized extreme learning machine
- 3.5: Prediction of GPS trajectory based on optimized support vector machine
- 3.6: Analysis of individual behavior based on prediction results
- 3.7: Conclusions
- References
- Chapter 4: Clustering and anomaly detection of crowd hotspot regions
- Abstract
- 4.1: Introduction
- 4.2: Description of crowd GPS data
- 4.3: Preprocessing of crowd GPS data
- 4.4: Clustering of crowd hotspot regions based on K-means
- 4.5: Clustering of crowd hotspot regions based on DBSCAN
- 4.6: Anomaly detection of crowd hotspot regions based on Markov chains
- 4.7: Conclusions
- References
- Chapter 5: Monitoring and deterministic prediction of station humidity
- Abstract
- 5.1: Introduction
- 5.2: Description of station humidity data
- 5.3: Deterministic prediction of station humidity based on optimization ensemble
- 5.4: Deterministic prediction of station humidity based on stacking ensemble
- 5.5: Evaluation of deterministic prediction results
- 5.6: Conclusions
- References
- Chapter 6: Monitoring and probabilistic prediction of station temperature
- Abstract
- 6.1: Introduction
- 6.2: Description of station temperature data
- 6.3: Interval prediction of station temperature based on quantile regression
- 6.4: Interval prediction of station temperature based on kernel density estimation
- 6.5: Evaluation of probabilistic prediction results
- 6.6: Conclusions
- References
- Chapter 7: Monitoring and spatial prediction of multidimensional air pollutants
- Abstract
- 7.1: Introduction
- 7.2: Description of multidimensional air pollutants data
- 7.3: Dimensionality reduction of multidimensional air pollutants data
- 7.4: Spatial prediction of air pollutants based on long short-term memory
- 7.5: Evaluation of spatial prediction results
- 7.6: Conclusions
- References
- Chapter 8: Time series feature extraction and analysis of metro loads
- Abstract
- 8.1: Introduction
- 8.2: Description of metro load data
- 8.3: Feature extraction of metro loads based on statistical methods
- 8.4: Feature extraction of metro loads based on transformation methods
- 8.5: Feature extraction of metro loads based on a model
- 8.6: Conclusions
- References
- Chapter 9: Characteristic and correlation analysis of metro loads
- Abstract
- 9.1: Introduction
- 9.2: The theoretical basis of correlation analysis
- 9.3: Description of metro load data
- 9.4: Correlation analysis of metro load and environment data
- 9.5: Correlation analysis of metro load and operation data
- 9.6: Comprehensive correlation ranking of metro load and related data
- 9.7: Conclusions
- References
- Chapter 10: Metro load prediction and intelligent ventilation control
- Abstract
- 10.1: Introduction
- 10.2: Description of short-term and long-term metro load data
- 10.3: Short-term prediction of metro load data based on ANFIS model
- 10.4: Long-term prediction of metro load data based on SARIMA model
- 10.5: Performance evaluation of prediction results
- 10.6: Intelligent ventilation control based on prediction results
- 10.7: Conclusions
- References
- Index
- No. of pages: 316
- Language: English
- Edition: 1
- Published: January 4, 2022
- Imprint: Elsevier
- Paperback ISBN: 9780323905886
- eBook ISBN: 9780323907125
HL
Hui Liu
CC
Chao Chen
YL
Yanfei Li
ZD
Zhu Duan
YL