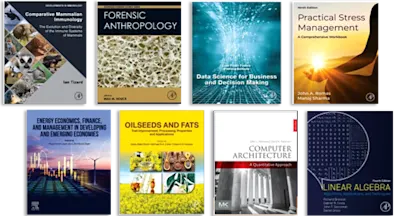
Robust Theoretical Models in Medicinal Chemistry
QSAR, Artificial Intelligence, Machine Learning, and Deep Learning
- 1st Edition - November 1, 2025
- Imprint: Elsevier
- Editors: Luciana Scotti, Marcus Tullius Scotti
- Language: English
- Paperback ISBN:9 7 8 - 0 - 4 4 3 - 2 7 4 2 0 - 6
- eBook ISBN:9 7 8 - 0 - 4 4 3 - 2 7 4 2 1 - 3
Robust Theoretical Models in Medicinal Chemistry: QSAR, Artificial Intelligence, Machine Learning, and Deep Learning serves as a valuable resource chock full of applications extend… Read more
Purchase options
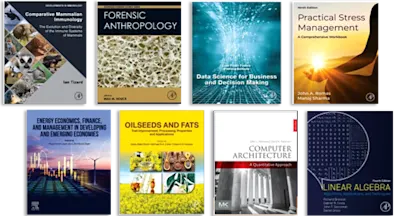
Robust Theoretical Models in Medicinal Chemistry: QSAR, Artificial Intelligence, Machine Learning, and Deep Learning serves as a valuable resource chock full of applications extending into multiple knowledge domains. The meticulous construction of a robust model holds significance, not only in drug discovery but also in engineering, chemistry, pharmaceutical, and food-related research, illustrating the broad spectrum of fields where QSAR methodologies can be instrumental. The activities considered in QSAR span chemical measurements and biological assays, making this approach a versatile tool applicable across various scientific domains. Currently, QSAR finds extensive use in diverse disciplines, prominently in drug design and environmental risk assessment.
Quantitative Structure-Activity Relationships (QSAR) represent a concerted effort to establish correlations between structural or property descriptors of compounds and their respective activities. These physicochemical descriptors encompass a wide array of parameters, accounting for hydrophobicity, topology, electronic properties, and steric effects, and can be determined empirically or, more recently, through advanced computational methods.
Quantitative Structure-Activity Relationships (QSAR) represent a concerted effort to establish correlations between structural or property descriptors of compounds and their respective activities. These physicochemical descriptors encompass a wide array of parameters, accounting for hydrophobicity, topology, electronic properties, and steric effects, and can be determined empirically or, more recently, through advanced computational methods.
- Provides specific introductions and discussions on QSAR theory and methods
- Analyzes QSAR applicability in Pharmaceutical Chemistry, Food Science, and Environmental Sciences
- Builds, validates, and interprets robust, predictive, and reliable QSAR models
Graduate students and researchers working with quantitative structure–activity relationship, Medicinal Chemistry, Deep learning and/or Machine learning applied to Medicinal Chemistry
1. Building QSAR models
2. Model, validation and prediction
3. Outliers and Negative Data
4. QSAR3- and 4D
5. QSAR and QSRP modelling
6. QSAR In Food Science
7. Interpretation of recent computational methods
8. Recent theoretical methods in the industry
9. Understanding the difference between machine learning and deep learning
10. Can artificial intelligence replace QSAR?
2. Model, validation and prediction
3. Outliers and Negative Data
4. QSAR3- and 4D
5. QSAR and QSRP modelling
6. QSAR In Food Science
7. Interpretation of recent computational methods
8. Recent theoretical methods in the industry
9. Understanding the difference between machine learning and deep learning
10. Can artificial intelligence replace QSAR?
- Edition: 1
- Published: November 1, 2025
- Imprint: Elsevier
- Language: English
LS
Luciana Scotti
Luciana Scotti is currently working as Senior Researcher at the Federal University of Paraíba, Brazil, where she completed her Ph.D. in Pharmacy. Her main area of interest focuses on Molecular and Computer Sciences. Her area of expertise includes Bioinformatics, Neglected Disease, Molecular Modelling, QSAR, and Natural Products. She has published 35 research articles in journals as well as 1 book and 2 book chapters contributed as author/co-author.
Affiliations and expertise
Senior Researcher, Federal University of Paraíba, BrazilMS
Marcus Tullius Scotti
Marcus Tullius Scotti studied chemical engineering at Universidade de São Paulo (USP - São Paulo University) and finished his degree in 1999. After, he worked for four years in a Brazilian electronics and telecommunications services company called Gradiente. At the same time, he started to study specialization on Industrial Administration at the University of São Paulo. After that, he started post-graduation in organic chemistry at the University of São Paulo in 2003 and finished his Master in 2005 and Ph.D. in 2008. In January of 2009, he moved to João Pessoa and started to work as Professor of Organic Chemistry at Universidade Federal da Paraíba (Federal University of Paraíba), Brazil. At beginning of 2014 he finished a post-doc in cheminformatics at Universidade Nova de Lisboa, Portugal. His research interests are in the chemistry of the natural products, acting on the following subjects: QSAR, Virtual Screening, molecular descriptors and chemotaxonomy using cheminformatics methods using statistical tools and machine learning algorithms. He has published mor than 240 papers, 19 book chapters and 155 abstracts in conferences.
Affiliations and expertise
Federal University of Paraíba, Paraíba, Brazil