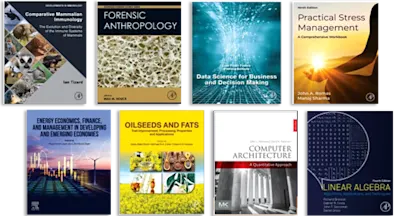
Revolutionizing Medical Systems using Artificial Intelligence
A Breakthrough in Healthcare
- 1st Edition - January 24, 2025
- Imprint: Academic Press
- Editors: Ashish Kumar, Divya Singh
- Language: English
- Paperback ISBN:9 7 8 - 0 - 4 4 3 - 3 2 8 6 2 - 6
- eBook ISBN:9 7 8 - 0 - 4 4 3 - 3 2 8 6 3 - 3
Revolutionizing Medical Systems using Artificial Intelligence: A Breakthrough in Healthcare provides an overview of various machine learning and deep learning techniques, addres… Read more
Purchase options
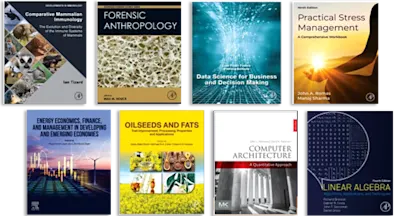
This title fulfills the needs of connected healthcare systems, providing insights into the role of Artificial Intelligence in the prognosis, diagnosis, and analysis of several diseases. It will be a valuable resource for health professionals, scientists and researchers, health practitioners, students, and all those who wish to broaden their knowledge in the challenging field of artificial intelligence in medical systems and diseases.
- Provides a wide range of coverage for various prediction and segmentation algorithms that are based on machine learning and deep learning technology
- Covers various predictive and segmentation algorithms exploited by various medical personnel to improve the accuracy of treatment to the patients
- Highlights improvements in quality and efficiency of medical decision-making in the early detection of critical diseases using AI
- Healthcare Management using Artificial Intelligence
- Background and research orientation of AI models for segmentation in Medical Imaging
- Benchmark Image and Clinical Datasets for Analysis in the Medical System
- Proactive Prognosis: Predicting the Course of Mental and Neurological Disorders with AI
- Exploring Brain Tumor Detection through Artificial Intelligence
- Empowering early detection: the role of Artificial Intelligence in breast cancer diagnosis
- AI based Disease Diagnosis Using Ultrasound Imaging
- Growth Optimization based SBLRNN model for Estimate Breast Cancer in IoT Healthcare Environment
- Detection of depression by utilizing Late Fusion of Sequential Actigraphy Features
- Developmental Pediatrics progression matched with Artificial Intelligence: A Growth Perspective in Healthcare
- AI in Precision Oncology: Advances in Cancer Treatment
- Impact of AI-bias in medical systems
- A Futuristic aspect towards Modern Health care system facilitated through Artificial Intelligence : A Comprehensive Perspective
- Satelliting Traditional to Smart Healthcare Advancement using Artificial Intelligence: A Paradigm Shift Routing Futuristic Slant- Redefining
- Welcoming the Evolution of Healthcare: A Transformative Change through AI and Robotics
- Edition: 1
- Published: January 24, 2025
- Imprint: Academic Press
- Language: English
AK
Ashish Kumar
Dr. Ashish Kumar, Ph.D., is working as an associate professor with Bennett University, Greater Noida, U.P., India till date. He has worked with Bharati Vidyapeeth’s College of Engineering (Affiliated to GGS Indraprastha University) from Aug 2009 to Jul 2022. He has completed his Ph.D. in Computer Science and Engineering from Delhi Technological University (formerly DCE), New Delhi, India in 2020. He received the Best Researcher award from the Delhi Technological University for his contribution to the computer vision domain. He has completed M. Tech with distinction in Computer Science and Engineering from GGS Indraprastha University, New Delhi. He has published more than 25 research papers in various reputed national and international journals and conferences. He has published 15+ book chapters in various Scopus-indexed books. He has authored/edited several books in AI, computer vision, and healthcare domains with reputed publishers. He is an active member of various international societies and clubs. He is a reviewer with many reputed journals and in the technical program committee of various national/ international conferences. Dr. Kumar also served as a session chair at many international and national conferences. His current research interests include object tracking, image processing, artificial intelligence, and medical imaging analysis.
DS
Divya Singh
Dr. Divya Singh is currently working as an assistant professor with the School of Computer Science Engineering and Technology, Bennett University, Greater Noida. She holds the degree of B.Tech in information technology and an M.Tech in Computer Science Engineering. She has completed Ph.D. degree in Computer Science Engineering and Technology from IIT, BHU, Varanasi. She has published research publications in various reputed international journals, conferences and patent. Her research interests include the areas of Image and Video Processing, Computer Vision, Artificial Intelligence (AI), Machine Learning and Deep learning.