Limited Offer
Save 50% on book bundles
Immediately download your ebook while waiting for your print delivery. No promo code needed.
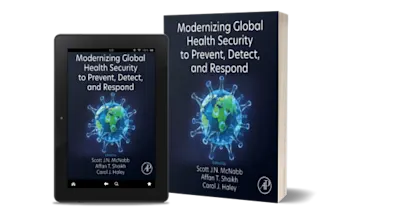
Responsible and Explainable Artificial Intelligence in Healthcare: Ethics and Transparency at the Intersection provides clear guidance on building trustworthy Artificial Intell… Read more
Limited Offer
AS
Prof. Akansha Singh, Professor at the School of Computer Science and Engineering, Bennett University, Greater Noida, boasts a comprehensive academic background with a B.Tech, M.Tech, and Ph.D. in Computer Science. Her doctoral studies, conducted at the prestigious IIT Roorkee, were focused on the cutting-edge fields of image processing and machine learning. A prolific author and scholar, Dr. Singh has contributed over 100 research papers and penned more than 25 books. Her editorial expertise is recognized by leading publishers such as Elsevier, Taylor and Francis, and Wiley, where she has edited books on a variety of emerging topics.Dr. Singh serves as the Associate Editor in IEEE Access, Discover Applied Science, PLOS One and guest editor in several journals. Her research interests are diverse and influential, spanning image processing, remote sensing, the Internet of Things (IoT), Blockchain and machine learning. Prof. Singh’s work in these areas not only advances the field of computer science but also significantly contributes to the broader scientific and technological community.
KS
Dr. Krishna Kant Singh, currently the esteemed Director of Delhi Technical Campus in Greater Noida, India, is a highly experienced educator and researcher in the field of engineering and technology. He is a B.Tech and M.Tech degree, a Postgraduate Diploma in Machine Learning and Artificial Intelligence from IIIT Bangalore, a Master of Science in Machine Learning and Artificial Intelligence from Liverpool John Moores University, United Kingdom, and a Ph.D. from IIT Roorkee. Dr. Singh has made significant contributions to the academic and research community. With over 19 years of teaching experience, he has played a vital role in educating and mentoring future professionals. Dr. Singh also serves as an Associate Editor at IEEE Access, an Editorial Board Member at Applied Computing and Geosciences (Elsevier), and a Guest Editor for Complex and Intelligent Systems. His extensive publication record includes over 132 research papers. His areas of interest include Machine Learning, Deep Learning, computer vision and so on.
II