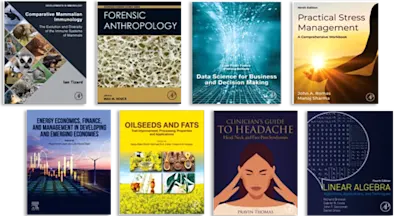
Reliable Non-Parametric Techniques for Energy System Operation and Control
Fundamentals and Applications of Constraint Learning and Safe Reinforcement Learning Methods
- 1st Edition - July 1, 2025
- Imprint: Elsevier
- Authors: Hongcai Zhang, Yonghua Song, Ge Chen, Peipei Yu
- Language: English
- Paperback ISBN:9 7 8 - 0 - 4 4 3 - 3 6 4 9 2 - 1
- eBook ISBN:9 7 8 - 0 - 4 4 3 - 3 6 4 9 3 - 8
Reliable Non-Parametric Techniques for Energy System Operation and Control: Fundamentals and Applications of Constraint Learning and Safe Reinforcement Learning Methods, a new Vo… Read more
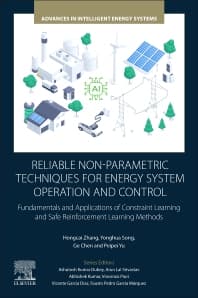
Purchase options
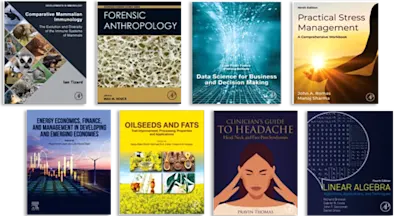
Institutional subscription on ScienceDirect
Request a sales quoteReliable Non-Parametric Techniques for Energy System Operation and Control: Fundamentals and Applications of Constraint Learning and Safe Reinforcement Learning Methods, a new Volume in the Advances in Intelligent Energy Systems, is a comprehensive guide to modern smart methods in energy system operation and control. This book covers fundamental concepts and applications in both deterministic and uncertain environments. It addresses the challenge of accuracy in imbalanced datasets and the limitations of measurements. The book delves into advanced topics such as safe reinforcement learning for energy system control, including training-efficient intrinsic-motivated reinforcement learning, and physical layer-based control, and more.
Other chapters cover barrier function-based control and CVaR-based control for systems without hard operation constraints. Designed for graduate students, researchers, and engineers, this book stands out for its practical approach to advanced methods in energy system control, enabling sustainable developments in real-world conditions.
Other chapters cover barrier function-based control and CVaR-based control for systems without hard operation constraints. Designed for graduate students, researchers, and engineers, this book stands out for its practical approach to advanced methods in energy system control, enabling sustainable developments in real-world conditions.
- Bridges the gap between theory and practice, providing essential insights for graduate students, researchers, and engineers
- Includes visual elements, data and code, and case studies for easy understanding and implementation
- Provides the latest release in the Advances in Intelligent Energy Systems series, bringing together the latest innovations in smart, sustainable energy
Graduate and upper-level undergraduate students, engineers, and researchers working on energy system operation and control
1. Introduction
PART I: ENERGY SYSTEM OPERATION BASED ON CONSTRAINT LEARNING
2. Fundamentals of Constraint Learning and Its Application in Deterministic Energy System Operation Problems
3. Extending Constraint Learning to Energy System Operations under Uncertain Environments
4. Ensuring Accuracy of Constraint learning in the Face of Imbalanced Operational Datasets
5. Overcoming Measurement Limitations by Combining Constraint Learning with Measurement Recovery
6. Mathematical Insights and Computationally-efficient Implementations of Constraint Learning
PART II: ENERGY SYSTEM CONTROL BASED ON SAFE-REINFORCEMENT LEARNING
7. Training-efficient Intrinsic-motived Reinforcement Learning Control for Energy Systems with Soft Operation Constraint
8. Physical Layer-based Safe Reinforcement Learning Control for Energy Systems with Accurate Formula of Hard Operation Constraint
9. Barrier Function-based Safe Reinforcement Learning Control for Energy Systems with Partially Formulable Hard Operation Constraint
10. CVaR-based Safe Reinforcement Learning Control for Energy Systems without Formula of Hard Operation Constraint
11. Conclusion
PART I: ENERGY SYSTEM OPERATION BASED ON CONSTRAINT LEARNING
2. Fundamentals of Constraint Learning and Its Application in Deterministic Energy System Operation Problems
3. Extending Constraint Learning to Energy System Operations under Uncertain Environments
4. Ensuring Accuracy of Constraint learning in the Face of Imbalanced Operational Datasets
5. Overcoming Measurement Limitations by Combining Constraint Learning with Measurement Recovery
6. Mathematical Insights and Computationally-efficient Implementations of Constraint Learning
PART II: ENERGY SYSTEM CONTROL BASED ON SAFE-REINFORCEMENT LEARNING
7. Training-efficient Intrinsic-motived Reinforcement Learning Control for Energy Systems with Soft Operation Constraint
8. Physical Layer-based Safe Reinforcement Learning Control for Energy Systems with Accurate Formula of Hard Operation Constraint
9. Barrier Function-based Safe Reinforcement Learning Control for Energy Systems with Partially Formulable Hard Operation Constraint
10. CVaR-based Safe Reinforcement Learning Control for Energy Systems without Formula of Hard Operation Constraint
11. Conclusion
- Edition: 1
- Published: July 1, 2025
- Imprint: Elsevier
- No. of pages: 350
- Language: English
- Paperback ISBN: 9780443364921
- eBook ISBN: 9780443364938
HZ
Hongcai Zhang
Hongcai Zhang is currently an Assistant Professor with the State Key Laboratory of Internet of Things for Smart City and the Department of Electrical and Computer Engineering at the University of Macau, China. Prior to this, he was a postdoctoral scholar with the University of California, USA, from 2018-2019. His current research interests include Internet of Things for smart energy, optimal operation and optimization of power and transportation systems, and grid integration of distributed energy resources. He has published over 70 JCR Q1/Q2 journal papers with 3 identified as ESI highly cited papers, and is an Associate Editor for IEEE Transactions on Power Systems and the Journal of Modern Power Systems and Clean Energy.
Affiliations and expertise
Assistant Professor, State Key Laboratory of Internet of Things for Smart City, University of Macau, ChinaYS
Yonghua Song
Yonghua Song is currently a Chair Professor and the Director of the State Key Laboratory in the Internet of Things for Smart City, both at the University of Macau, China. He is also the Vice President of Chinese Electrotechnical Society, an international member of Academia Europaea, a fellow of the Royal Academy of Engineering (UK), and an IEEE fellow. He has long been engaged in developing renewable electrical power systems and smart energy research. He has published over 200 scientific journal papers and authored/edited 10 books. He has won the second prize of the State Scientific and Technological Progress Award and the prize for Scientific and Technological Progress from the Ho Leung Ho Lee Foundation, China.
Affiliations and expertise
Chair Professor and Director, State Key Laboratory in the Internet of Things for Smart City, University of Macau, ChinaGC
Ge Chen
Ge Chen is currently a Postdoctoral Research Associate with Purdue University, USA. His research interests include the Internet of Things for smart energy, optimal operation, and data-driven optimization under uncertainty.
Affiliations and expertise
Postdoctoral Research Associate, Purdue University, USAPY
Peipei Yu
Peipei Yu is currently a Ph.D. candidate in electrical and computer engineering at the University of Macau, China. Her research interests include learning-based control, ancillary services for demand response, and integrated energy systems. She has published 7 JCR Q1/Q2 journal papers.
Affiliations and expertise
PhD Candidate, University of Macau, China