Limited Offer
Save 50% on book bundles
Immediately download your ebook while waiting for your print delivery. No promo code needed.
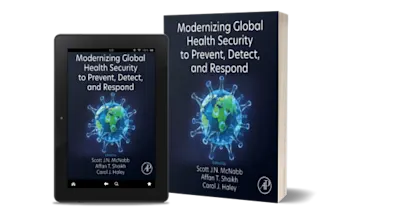
Radiomics and Radiogenomics in Neuro-Oncology: An Artificial Intelligence Paradigm—Volume 2: Genetics and Clinical Applications provides readers with a broad and detailed framew… Read more
Limited Offer
Radiomics and Radiogenomics in Neuro-Oncology: An Artificial Intelligence Paradigm—Volume 2: Genetics and Clinical Applications provides readers with a broad and detailed framework for radiomics and radiogenomics (R-n-R) approaches with AI in neuro-oncology. It delves into the study of cancer biology and genomics, presenting methods and techniques for analyzing these elements. The book also highlights current solutions that R-n-R can offer for personalized patient treatments, as well as discusses the limitations and future prospects of AI technologies.
Volume 1: Radiogenomics Flow Using Artificial Intelligence covers the genomics and molecular study of brain cancer, medical imaging modalities and their analysis in neuro-oncology, and the development of prognostic and predictive models using radiomics.
Volume 2: Genetics and Clinical Applications extends the discussion to imaging signatures that correlate with molecular characteristics of brain cancer, clinical applications of R-n-R in neuro-oncology, and the use of Machine Learning and Deep Learning approaches for R-n-R in neuro-oncology.
Biomedical Engineers and researchers in Neural Engineering, medical imaging, and neural networks. Other interested audiences will be comprised of radiologists, neurologists, neurosurgeons, computer scientists, AI researchers, and designers of Machine Learning applications. Another audience includes those interested in signal processing of the brain and classifying brain signals, Clinicians and researchers interested in neurological diseases and disorders, including their diagnosis and treatment. Tumor imaging oncologists will also be a secondary audience
SS
Dr. Sanjay Saxena is an Assistant Professor in the Department of Computer Science and Engineering at IIIT Bhubaneswar, India. He obtained his Ph.D. from the Indian Institute of Technology (BHU), Varanasi, and completed his postdoctoral research at the Artificial Intelligence in Biomedical Imaging Lab, University of Pennsylvania, USA. Dr. Saxena's primary research area involves developing AI-based methods for brain cancer analysis, with a broader focus on Data Science, Machine Learning, Deep Learning, and the emerging fields of Radiomics and Radiogenomics. His tenure under Prof. Davatzikos at the University of Pennsylvania was instrumental in his significant learning about Radiomics/Radiogenomics. Dr. Saxena is a member of prestigious organisations such as IEEE, the Society of Neuro-Oncology, ACM, and the New York Academy of Science. He has presented his work at globally recognised universities such as like Imperial College London, Stony Brook University, and Vienna University of Technology. and has made substantial scholarly contributions, with several peer-reviewed Journals, conference publications, book chapters, and two books to his name, advancing the intersection of AI and human cancer research.
JS
Dr. Jasjit Suri, PhD, MBA, is an innovator, visionary, scientist, and internationally known world leader. Dr Suri received the Director General’s Gold medal in 1980 and Fellow of (i) American Institute of Medical and Biological Engineering, awarded by the National Academy of Sciences, Washington DC, (ii) Institute of Electrical and Electronics Engineers, (iii) American Institute of Ultrasound in Medicine, (iv) Society of Vascular Medicine, (v) Asia Pacific Vascular Society, and (vi) Asia Association of Artificial Intelligence. Dr. Suri was honored with life time achievement awards by Marcus, NJ, USA and Graphics Era University, Dehradun, India. He has published nearly 300 peer-reviewed Artificial Intelligence articles, nearly 2000 Google Scholar Publications, 100 books, and 100 innovations/trademarks leading to an H-index of nearly 100 with about 43,000 citations. He has held positions as chairman of AtheroPoint, CA, USA, IEEE Denver section, Colorado, USA, and advisory board member to healthcare industries and several universities in the United States of America and abroad.