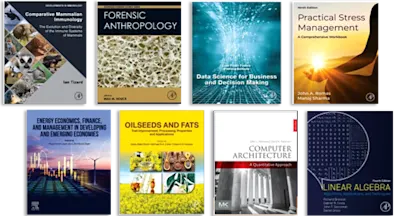
Radiomics and Radiogenomics in Neuro-Oncology
An Artificial Intelligence Paradigm - Volume 1: Radiogenomics Flow Using Artificial Intelligence
- 1st Edition - April 5, 2024
- Imprint: Academic Press
- Editors: Sanjay Saxena, Jasjit S. Suri
- Language: English
- Paperback ISBN:9 7 8 - 0 - 4 4 3 - 1 8 5 0 8 - 3
- eBook ISBN:9 7 8 - 0 - 4 4 3 - 1 8 5 0 7 - 6
Radiomics and Radiogenomics in Neuro-Oncology: An Artificial Intelligence Paradigm, Volume One: Radiogenomics Flow Using Artificial Intelligence broadly encompasses the study… Read more
Purchase options
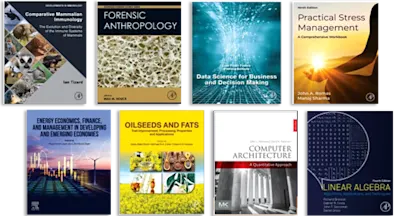
- Includes coverage on the foundational concepts of the emerging fields of radiomics and radiogenomics
- Covers neural engineering modeling and AI algorithms for the imaging, diagnosis, and predictive modeling of neuro-oncology
- Presents crucial technologies and software platforms, along with advanced brain imaging techniques such as quantitative imaging using CT, PET, and MRI
- Provides in-depth technical coverage of computational modeling techniques and applied mathematics for brain tumor segmentation and radiomics features such as extraction and selection
1. Fundamental pipelines of Radiomics and Radiogenomics(R-n-R)
Contributors - Ishan Ayus, Biswajit Jena, Sanjay Saxena
2. Artificial Intelligence, its Components and crucial technologies for implementation
Contributors - Rupsa Bhattacharjee, Snekha Thakran
3. Radiomics and Radiogenomics(R-n-R) with Artificial Intelligence: approaches, applications, advances, current challenges, future perspectives
Contributor - Pinar Kirci
Section 2: Genomics and Molecular Study of Brain Cancer
4. Brain Cancer and World Health Organization
Contributors - Moirangthem Gelson Singh , Sanjay Saxena , Suvendu Rup , Sarthak Padhi
5. Genomic and Genetic levels alteration in Brain Cancer
Contributors - Shantilata Palei, Sakshi Arora, Sanjay Saxena, Niranjan Kaushik
6. Role of Molecular Markers in Diagnosis and Prognosis of Gliomas(Brain Tumor)
Contributor - Anand Kumar Pandey
7. Multi-omics studies in Neuro-Oncology
Contributors - Mullapudi Venkata Sai Samartha, Sakshi Arora, Shantilata Palei, Vitthal Gupta, Sanjay Saxena
Section 3: Medical Imaging Modalities and Analysis in Neuro-Oncology
8. Medical image analysis steps: Medical image acquisition to classification(or regression) in Neuro-Oncology
Contributors - Suchismita Das, Meghna, Sanjay Saxena
9. MRI: An important Biomarker for radiomics study of brain cancer and Its uses in Machine Learning
Contributors - Sudip Paul and Vinayak Majhi
10. Deep learning algorithms for imaging gliomas for diagnosis, prognosis, and treatment strategies predictions
Contributor- Anand Kumar Pandey
Section 4: Radiomics and Radiogenomics in Neuro-Oncology
11. Radiomics and Radiogenomics of Central Nervous System Metastatic Lesions
Contributor - Teresa Perillo
12. Clinical Applications implementation in Neuro-Oncology using Machine Learning Approaches
Contributors - Biswajit Jena, Ishan Ayus, Sanjay Saxena
13. Applications and Constraints of Artificial Intelligence in Radiomics and Radiogenomics(R-n-R) Studies in Neuro-Oncology
Contributors - Shovna Panda, Sarthak Padhi, Vitthal Gupta, Jasjit S. Suri, Sanjay Saxena
- Edition: 1
- Published: April 5, 2024
- Imprint: Academic Press
- Language: English
SS
Sanjay Saxena
Dr. Sanjay Saxena is an Assistant Professor in the Department of Computer Science and Engineering at IIIT Bhubaneswar, India. He obtained his Ph.D. from the Indian Institute of Technology (BHU), Varanasi, and completed his postdoctoral research at the Artificial Intelligence in Biomedical Imaging Lab, University of Pennsylvania, USA. Dr. Saxena's primary research area involves developing AI-based methods for brain cancer analysis, with a broader focus on Data Science, Machine Learning, Deep Learning, and the emerging fields of Radiomics and Radiogenomics. His tenure under Prof. Davatzikos at the University of Pennsylvania was instrumental in his significant learning about Radiomics/Radiogenomics. Dr. Saxena is a member of prestigious organisations such as IEEE, the Society of Neuro-Oncology, ACM, and the New York Academy of Science. He has presented his work at globally recognised universities such as like Imperial College London, Stony Brook University, and Vienna University of Technology. and has made substantial scholarly contributions, with several peer-reviewed Journals, conference publications, book chapters, and two books to his name, advancing the intersection of AI and human cancer research.
JS
Jasjit S. Suri
Dr. Jasjit Suri, PhD, MBA, is a renowned innovator and scientist. He received the Director General’s Gold Medal in 1980 and is a Fellow of several prestigious organizations, including the American Institute of Medical and Biological Engineering and the Institute of Electrical and Electronics Engineers. Dr. Suri has been honored with lifetime achievement awards from Marcus, NJ, USA, and Graphics Era University, India. He has published nearly 300 peer-reviewed AI articles, 100 books, and holds 100 innovations/trademarks, achieving an H-index of nearly 100 with about 43,000 citations. Dr. Suri has served as chairman of AtheroPoint, IEEE Denver section, and as an advisory board member to various healthcare industries and universities globally.