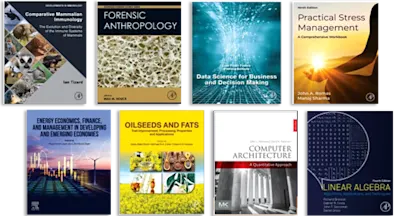
Python for Quantum Chemistry
A Full Stack Programming Guide
- 1st Edition, Volume 23 - June 24, 2025
- Imprint: Elsevier
- Author: Qiming Sun
- Language: English
- Paperback ISBN:9 7 8 - 0 - 4 4 3 - 2 3 8 3 7 - 6
- eBook ISBN:9 7 8 - 0 - 4 4 3 - 2 3 8 3 6 - 9
Quantum chemistry requires ever higher computational performance, with more and more sophisticated and dedicated Python scripts being required to solve challenging problems. Al… Read more
Purchase options
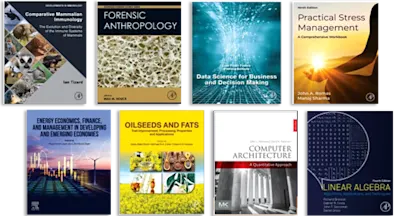
Quantum chemistry requires ever higher computational performance, with more and more sophisticated and dedicated Python scripts being required to solve challenging problems. Although resources for basic use of Python are widely (and often freely) available online and in literature, truly cohesive materials for advanced Python programming skills are lacking.
Qiming Sun, a developer of the popular Python package PySCF, provides a comprehensive, end-to-end practical resource for researchers and engineers who have basic Python programming experiences chiefly in computational chemistry but want to take their use of the software forwards to the next level, the book provides an insightful exploration of Numpy, Pandas, and other data analysis tools. Readers will learn how to manage their Python computational projects in a professional way, with various tools and protocols for computational chemistry research and general scientific computing tasks exhibited and analysed from a technical perspective. Multiple programming paradigms including object-oriented, functional, meta-programming, dynamic, concurrent, and vector-oriented are illustrated in various technology scenarios allowing readers to properly use them to enhance their program projects. Readers will also learn how to use the presented optimization technologies to speed up their Python applications, even to the level as fast as a native C++ implementation. The applications of these technologies are then demonstrated using quantum chemistry Python applications.
Python for Quantum Chemistry: A Full Stack Programming Guide is written primarily for graduate students, researchers and software engineers working primarily in the fields of theoretical chemistry, computational chemistry, condensed matter physics, material modelling, molecular simulations, and quantum computing.
- End-to end guide for advanced Python programming skills and tools related to quantum chemistry research
- Tackles the following questions: How can you ensure the Python runtime is manageable when the preliminary implementation becomes complicated or evolves many branches? How do I ensure that others' Python program works properly in my project? How do I make my Python project reusable for others?
- Covers in depth the crucial topic of Python code optimization methods with high-performance computing technologies
- Provides examples of Python applications with cutting-edge technologies such as automatic code generation, cloud computing, and GPGPU
- Includes discussion of Python runtime mechanism and advanced Python technologies
1. Research environment in Python
1.1 Python package system
1.1.1 Python runtime
1.1.2 pip
1.1.3 virtualenv
1.1.4 Conda
1.2 Managing Python projects
1.2.1 Package, subpackage, and module
1.2.2 Object-oriented programming
1.2.3 Inheritance and Mixins
1.2.4 Testing a Python program
1.2.5 Releasing a Python library
1.2.6 Version control
1.2.7 Python programs in docker
1.2.8 CI/CD and DevOps workflow
1.3 Coding Pythonically
1.3.1 PEP8 and coding style
1.3.2 Documenting Python code
1.4 Work with Ipython and Jupyter
1.4 Summary
2. Data processing
2.1 Vectorized operations with numpy
2.1.1 Introduction of numpy arrays
2.1.2 Universal functions
2.1.3 Mask array
2.1.4 Fancy-index
2.1.5 Memory view and numpy data structure
2.1.6 Vector oriented programming
2.2 Data with labels: pandas
2.2.1 Introduction of Pandas data objects
2.2.2 Indexing data in pandas
2.2.3 Missing data
2.2.4 The groupby function
2.2.5 eval and query in pandas
2.2.6 Using numpy to speed-up pandas
2.3 Visualizing the data
2.3.1 Matplotlib
2.3.2 Plotly
2.3.2 Mayavi
2.4 Data type and precision
2.4.1 The annoyed nan
2.4.2 Data with finite precision
2.4.3 Arbitrary precision
2.5 Summary
3. Scientific computing tools
3.1 Scipy
3.1.1 Linear algebra
3.1.1 Optimization with scipy.optimize
3.1.2 Fourier transforms
3.1.3 Sparse array
3.2 Convex optimization
4. IO
4.1 Data serialization
4.1.1 Pickle
4.1.2 Human-readable serialization
4.1.4 Memory map
4.2 JSON, YAML, CSV, XML
4.2 HDF5 for Numpy and Pandas data
4.3 Key-value data
4.4 Storing data in database
4.4.1 SQL
4.4.2 NoSQL
4.5 Summary
5. How to communicate with other programs
5.1 Common data format in chemistry
5.2 Human readable format
5.2.1 Format with molecular geometry
5.2.2 Format with wavefunction information
5.3 Binary format
5.4 Tools for format conversion
5.5 Visualization
5.6 Summary
6. Code generation
6.1 Meta-programming in Python
6.1.1 Manipulating class and function at runtime
6.1.2 eval and exec
6.1.3 The inspect module
6.2 Symbolic programming
6.2.1 Sympy
6.2.2 Programming for symbolic algebra
6.3 Automatic differentiation
6.3.1 Introductions and concepts
6.3.2 Torch
6.3.3 Jax
6.4 Summary
7. Workflow and job scheduler
7.1 Job scheduler
7.1.1 Dask
7.1.2 Ray
7.1.3 Joblib
7.1.4 Ipyparallel
7.2 Utilising cloud computing
7.2.1 Function as a service
7.2.2 Distributed workers
7.2.3 Object storage
Part II: High performance computing with Python
8. Combining Python with other programming languages
8.1 C/C++
8.1.1 Data types and data type conversion
8.1.2 How to compile c++ code to a Python module
8.1.3 Cython
8.1.4 Pybind11
8.2 Fortran
8.2.1 Data types and ABI conventions
8.2.2 How to compile a Fortran project to a Python module
8.2.3 The f2py compiler
8.3 Foreign language interface
8.4 Summary
9. Code performance optimization
9.1 Principles in performance optimization
9.1.1 Cost estimation
9.1.2 CPU bound, or IO bound
9.1.2 Test-driven
9.2 Profiling the program
9.2.1 Benchmark test
9.2.2 Python built-in profiling tools
9.2.3 Line profiler
9.2.4 Sampling profiler
9.2.5 The dis module
9.3 Compiling Python code
9.3.1 Just-in-time compilation
9.3.2 Python extensions with Cython and Pythran
9.3.3 Codon compiler
9.4 Optimization with compiling languages
9.5 Data-intensive applications
9.5.1 Optimising data structure for performance
9.5.2 Compressing data
9.5.3 Prefetching data
9.5.4 Utilising cache
9.5.5 Asynchronized computation
9.6 Precomputing and memoizing results
9.6.1 Side-effect free functions
9.6.2 Hashing input arguments
9.6.3 LRU cache
9.6.4 Dynamic programming
9.6.5 Tabulating results
9.7 Optimization with lazy evaluation
9.8 Summary
10. Tensor
10.1 Manipulating a tensor is more efficient, why?
10.2 Tensor operations in Numpy
10.2.1 dot and BLAS functions
10.2.2 tensordot
10.2.3 einsum
10.3 High performance tensor library
10.3.1 Tblis
10.3.2 Cupy.einsum and cutensor
10.4 Summary
11. Parallelism
11.1 Multithreading
11.2 Multiprocessing
11.3 Message queue
11.4 Interprocess communication
11.4.1 MPI
11.4.2 ZMQ
11.5 Summary
12. Python with GPU
12.1 Introduction to CUDA and GPU runtime
12.2 Cupy
12.3 Pycuda
Part III: Quantum chemistry method development with Python
13. Integral evaluation
13.1 Numerical integration
13.1.1 Integral quadrature
13.1.2 Integration with Fourier transform
13.1.3 Interpolation and extrapolation
13.2 Analytical and semi-analytical integral evaluation for Gaussian functions
13.3 Integral transformation
14. Numerical optimization methods
14.1 Newton and quasi-newton
14.1.1 BFGS
14.1.2 Augmented Hessian
14.2 Krylov subspace methods
14.3 Direct inversion in the iterative subspace (DIIS)
15. Mean-filed methods
15.1 The simplest self-consistency field code
15.1.1 Coulomb matrix and exchange matrix
15.1.2 Exchange-correlation functionals
15.1.3 Self-consistency iterations
15.2 Speeding up mean-field calculations
15.2.1 Cholesky decomposition and resolution of identity methods
15.2.2 Improving convergence
15.2.3 Improving initial guess
15.2.4 Reducing memory footprint with AO-driven technique
15.3 Localised molecular orbitals
16. Post-Hartree-Fock methods
16.1 Full configuration interaction
16.1.1 Building Hamiltonian with Slater-Condon rule
16.1.2 Diagonalization of a large matrix
16.1.3 String-based direct diagonalization
16.1.1 Speed up FCI with Cython
16.2 Coupled cluster
16.2.1 Implementation with tensor library
16.2.2 Optimising coupled cluster performance in Python
16.3 Many-body perturbation theory
16.4 Multi-reference methods
16.5 Quantum computing solver
16.5.1 Matrix product states
16.5.2 Quantum neural network wave-function
16.5.3 Qiskit and Openfermion
17. Molecular properties
17.1 Electron density in real space
17.2 Electrostatic potential and effective potential
17.3 Force and geometry optimization
17.3.1 Finite difference
17.3.2 Analytical nuclear gradients
17.3.3 Redundant internal coordinates for geometry optimization
17.4 Nuclear hessian and thermo-chemistry properties
17.5 Molecular properties visualisation
18. Symmetry
18.1 Point group symmetry
18.2 Translational symmetry
18.3 Angular momentum coupling
18.4 Permutation symmetry in tensors
- Edition: 1
- Volume: 23
- Published: June 24, 2025
- Imprint: Elsevier
- Language: English
QS