Limited Offer
Save 50% on book bundles
Immediately download your ebook while waiting for your print delivery. No promo code needed.
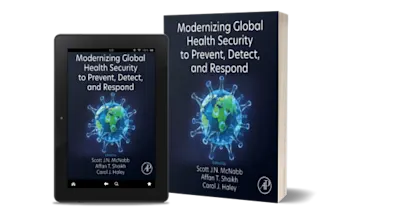
Probability Theory and Mathematical Statistics for Engineers focuses on the concepts of probability theory and mathematical statistics for finite-dimensional random variables… Read more
Limited Offer
Probability Theory and Mathematical Statistics for Engineers focuses on the concepts of probability theory and mathematical statistics for finite-dimensional random variables.
The book underscores the probabilities of events, random variables, and numerical characteristics of random variables. Discussions focus on canonical expansions of random vectors, second-order moments of random vectors, generalization of the density concept, entropy of a distribution, direct evaluation of probabilities, and conditional probabilities. The text then examines projections of random vectors and their distributions, including conditional distributions of projections of a random vector, conditional numerical characteristics, and information contained in random variables.
The book elaborates on the functions of random variables and estimation of parameters of distributions. Topics include frequency as a probability estimate, estimation of statistical characteristics, estimation of the expectation and covariance matrix of a random vector, and testing the hypotheses on the parameters of distributions. The text then takes a look at estimator theory and estimation of distributions.
The book is a vital source of data for students, engineers, postgraduates of applied mathematics, and other institutes of higher technical education.