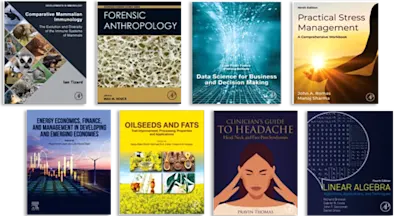
Probability for Deep Learning Quantum
A Many-Sorted Algebra View
- 1st Edition - January 21, 2025
- Imprint: Morgan Kaufmann
- Author: Charles R. Giardina
- Language: English
- Paperback ISBN:9 7 8 - 0 - 4 4 3 - 2 4 8 3 4 - 4
- eBook ISBN:9 7 8 - 0 - 4 4 3 - 2 4 8 3 5 - 1
Probability for Deep Learning Quantum provides readers with the first book to address probabilistic methods in the deep learning environment and the quantum technological area simu… Read more
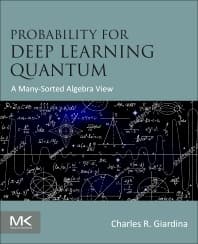
Purchase options
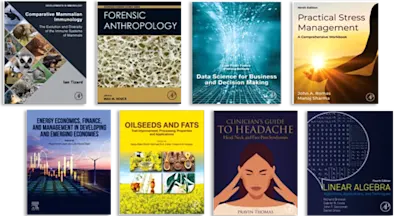
Institutional subscription on ScienceDirect
Request a sales quoteProbability for Deep Learning Quantum provides readers with the first book to address probabilistic methods in the deep learning environment and the quantum technological area simultaneously, by using a common platform: the Many-Sorted Algebra (MSA) view. While machine learning is created with a foundation of probability, probability is at the heart of quantum physics as well. It is the cornerstone in quantum applications. These applications include quantum measuring, quantum information theory, quantum communication theory, quantum sensing, quantum signal processing, quantum computing, quantum cryptography, and quantum machine learning. Although some of the probabilistic methods differ in machine learning disciplines from those in the quantum technologies, many techniques are very similar.
Probability is introduced in the text rigorously, in Komogorov’s vision. It is however, slightly modified by developing the theory in a Many-Sorted Algebra setting. This algebraic construct is also used in showing the shared structures underlying much of both machine learning and quantum theory. Both deep learning and quantum technologies have several probabilistic and stochastic methods in common. These methods are described and illustrated using numerous examples within the text. Concepts in entropy are provided from a Shannon as well as a von-Neumann view. Singular value decomposition is applied in machine learning as a basic tool and presented in the Schmidt decomposition. Besides the in-common methods, Born’s rule as well as positive operator valued measures are described and illustrated, along with quasi-probabilities. Author Charles R. Giardina provides clear and concise explanations, accompanied by insightful and thought-provoking visualizations, to deepen your understanding and enable you to apply the concepts to real-world scenarios.
- Provides readers with a resource that is loaded with hundreds of well-crafted examples illustrating the difficult concepts pertaining to quantum and stochastic processes
- Addresses probabilistic methods in the deep learning environment and in the quantum technological area
- Includes a rigorous and precise presentation of the algebraic underpinning of both quantum and deep learning
- Probability for Deep Learning Quantum
- Cover image
- Title page
- Table of Contents
- Copyright
- Dedication
- List of figures
- About the author
- Preface
- Acknowledgment
- 1 Introduction to a many-sorted algebra view
- Abstract
- Keywords
- 1.1 A many-sorted algebra view
- 1.2 Many-sorted algebra view of a metric space
- 1.3 Metrics for probability distributions
- 1.4 Many-sorted algebra view of a topological space
- 1.5 Classification of topological spaces
- 1.6 Manifolds, charts, and atlases
- 1.7 MSA for Riemannian and symplectic geometrical manifolds
- 1.8 Some properties of manifolds
- 1.9 Fiber bundles
- 1.10 Tangent vectors and tangent bundles
- 1.11 MSA for lie groups and lie algebras
- References
- 2 Information geometry decision theory
- Abstract
- Keywords
- 2.1 Brief overview of information geometry foundations of probabilistic modeling
- 2.2 Riemannian metric, length, connections, and parallel transport
- 2.3 Distance functions semidistance
- 2.4 Concentration inequalities
- 2.5 Loss functions and decision functions
- 2.6 Conditional probability and partitions of unity
- 2.7 Calculating the Fisher-Rao information matrix and metric
- 2.8 Shannon entropy as a measure of information
- 2.9 The empirical distribution
- 2.10 Manifolds of probability and natural parameters
- 2.11 Parametrization for the exponential family of distributions
- 2.12 Quantum mechanical information metric
- 2.13 Von Neumann entropy and other measures of information
- References
- 3 Symplectic tomographic probability
- Abstract
- Keywords
- 3.1 MSA for symplectic and metaplectic spaces
- 3.2 Liouville's classical theorem on invariance of probability in phase space
- 3.3 Radon transform in tomography
- 3.4 Pauli's conjecture and quantum tomography
- 3.5 Fourier transforms of Schwartz space functions
- 3.6 Fourier transforms of distributions
- 3.7 Fourier transforms in L1 and L2
- 3.8 Hilbert transform for tomography and probability
- 3.9 Radon inversion
- 3.10 Wigner function
- 3.11 Symplectic radon transform and metaplectic representation
- References
- 4 The Born rule for quantum probability calculations
- Abstract
- Keywords
- 4.1 Axioms for quantum
- 4.2 Born's rule for wave functions: A formal version
- 4.3 Ehrenfest's theorems and generalizations
- 4.4 Born rule discrete spectrum
- 4.5 Born rule continuous spectrum
- 4.6 MSA view of measurable and measure spaces
- 4.7 Direct integrals
- 4.8 Projection-valued measures
- 4.9 Rigged Hilbert spaces
- 4.10 Positive operator-valued measures
- 4.11 Gleason's theorem on Born rule
- 4.12 Generalized uncertainty principle in Hilbert space
- References
- 5 MSA view for a random variable algebra
- Abstract
- Keywords
- 5.1 MSA description of a probability space
- 5.2 Conditional probability
- 5.3 MSA for probability distribution functions
- 5.4 MSA view of joint measurable spaces
- 5.5 MSA for combining random variables, objective
- 5.6 MSA showing Unital ring and vector space of random variables
- 5.7 MSA, an algebra of random variables
- 5.8 MSA description for random variables in L0
- 5.9 MSA description for random variables in L1
- References
- 6 Algebra illustrations using probability indicators
- Abstract
- Keywords
- 6.1 Probability indicators
- 6.2 The sum of two random variables: A rigorous look
- 6.3 Fourier transform probability indicators
- 6.4 The MINUS operation
- 6.5 Ring with ONE
- 6.6 Inverse and field structure
- 6.7 MSA for a vector space of random variables
- 6.8 MSA for a normed vector space of random variables
- Reference
- 7 Algebras for complex and quaternion random variables
- Abstract
- Keywords
- 7.1 Measurable space for complex-valued random variables
- 7.2 Density functions for a complex-valued random variable
- 7.3 Banach algebra structure
- 7.4 Banach* and C* algebra structures
- 7.5 Characteristic function for complex-valued random variables
- 7.6 Euler's theorem for quaternions
- 7.7 MSA view of quaternion measurable space
- 7.8 Characteristic function of quaternion random variable
- Reference
- 8 MSA for stochastic processes, and large deviation theory
- Abstract
- Keywords
- 8.1 MSA view of stochastic processes
- 8.2 Levy stochastic processes
- 8.3 Wiener process and Brownian motion from a random walk process
- 8.4 Large deviation theory
- 8.5 Comparative decision criteria and distance measurements using large deviation principle
- 8.6 Asymptotically most favorable probability
- References
- 9 Probability in Fock space
- Abstract
- Keywords
- 9.1 Overview
- 9.2 Maxwell-Boltzman probability distribution
- 9.3 Maxwell-Boltzmann, Bose-Einstein, Fermi-Dirac distributing functions
- 9.4 Particles within Fock spaces and Fock space structure
- 9.5 The Bosonic occupation numbers and the ladder operators
- 9.6 Bose-Einstein thermal statistics
- 9.7 The Fermionic Fock space and the fermionic ladder operators
- 9.8 The Slater determinant
- 9.9 Unitary coupled cluster
- 9.10 One- and two-electron integrals
- 9.11 The variational quantum eigensolver
- References
- 10 Applied probability in quantum
- Abstract
- Keywords
- 10.1 Probability of quantum interference
- 10.2 Young's experiment explaining interference and support for the born rule
- 10.3 Probabilistic coupling
- 10.4 Probability current, probability flux, and the continuity equation
- 10.5 Levy's continuity theorem
- 10.6 Noether's theorem
- 10.7 Klein-Gordon probability density
- 10.8 Positive operator-valued measures
- 10.9 Mutually unbiased bases
- References
- 11 Entanglement
- Abstract
- Keywords
- 11.1 Decoherence time
- 11.2 The reduced density matrix and partial trace operation
- 11.3 Schmidt decomposition
- 11.4 Purification and partial isometries
- 11.5 The fidelity for density matrices
- 11.6 The swapping operation
- 11.7 Quantum circuit depth and width
- 11.8 The Rand index
- 11.9 Depolarizing channel probability
- 11.10 Query cost and quantum time complexity
- References
- 12 Quasiprobability
- Abstract
- Keywords
- 12.1 Quasiprobability basic concepts
- 12.2 Noncommuting operators in quantum
- 12.3 Ladder operator representations
- 12.4 Ladder operators for density functions
- 12.5 Displacement operators and characteristic functions
- 12.6 Operator-ordered representations
- 12.7 The quasiprobability density functions
- 12.8 Relationships between quasiprobability densities
- 12.9 Kirkwood-Dirac quasiprobability
- References
- 13 Noisy intermediate scale quantum NISQ computing
- Abstract
- Keywords
- 13.1 Testing NISC computers
- 13.2 Fidelity optimal mapping on NISQ swap gate
- 13.3 Fidelity optimal mapping on NISQ qubit layout
- 13.4 Fidelity optimal mapping on NISQ solutions
- 13.5 Testing NISQ computers randomized circuits
- References
- 14 Machine learning meets quantum
- Abstract
- Keywords
- 14.1 Quantum application of deep neural nets
- 14.2 Quantum machine learning VQE methods
- 14.3 Quantum machine learning clustering
- 14.4 Quantum advantage in machine learning
- 14.5 Adjusted clustering index classification
- 14.6 Adjusted clustering index probability
- References
- Appendix A1 MSA description for a ring, a field, a vector space and an inner product or Hilbert space
- Abstract
- Keywords
- A1.1 Field structure
- A1.2 Field substructures, such as groups, rings, and integral domains
- A1.3 Vector space structure
- A1.4 Inner product and Hilbert space
- References
- Appendix A2 MSA description for an algebra, a normed vector space, and a Banach space
- Abstract
- Keywords
- A2.1 Algebra structures
- A2.2 Normed vector space and Banach space structures
- Appendix A3 MSA description of a Banach algebra, a Banach* algebra, and a C* algebra
- Abstract
- Keywords
- A3.1 Banach algebra
- A3.2 Banach algebra* and C* algebra
- Reference
- Appendix A4 MSA description of the quaternion skew field
- Abstract
- Keywords
- A4.1 Quaternions as a skew field
- A4.2 The MSA showing quaternions are a vector space
- A4.3 The MSA showing quaternion inner product and Hilbert space
- A4.4 The MSA showing quaternions form a normed vector space and a Banach space
- A4.5 The MSA showing the vector space of quaternions is a unital associative algebra
- References
- Appendix A5 MSA description of Schwartz space and delta functions
- Abstract
- Keywords
- A5.1 The Schwartz space
- Test functions over Rn form a Schwartz space
- A5.2 Formal description of a Dirac delta function
- References
- Appendix A6 MSA description of a lattice
- Abstract
- Keywords
- A6.1 MSA description of lattices, valuation spaces, directed sets, and nets
- MSA view of weighted graphs and probability graphs for max-cut testing
- References
- Appendix A7 Classical and quantum probability
- Abstract
- Keywords
- A7.1 Expectations in terms of traces
- A7.2 Importance sampling
- A7.3 The Bernoulli distribution
- A7.4 Multinomial distribution
- Reference
- Index
- Edition: 1
- Published: January 21, 2025
- No. of pages (Paperback): 362
- No. of pages (eBook): 250
- Imprint: Morgan Kaufmann
- Language: English
- Paperback ISBN: 9780443248344
- eBook ISBN: 9780443248351
CG
Charles R. Giardina
Charles R. Giardina was born in the Bronx, NY, on December 29, 1942. He received the B.S. degree in mathematics from Fairleigh Dickinson University, Rutherford, NJ, and the M.S. degree in mathematics from Carnegie Institute of Technology, Pittsburgh, PA. He also received the M.E.E. degree in 1969, and the Ph.D. degree in mathematics and electrical engineering in 1970 from Stevens Institute of Technology, Hoboken, NJ. Dr. Giardina was Professor of Mathematics, Electrical Engineering, and Computer Science at Fairleigh Dickinson University from 1965 to 1982. From 1982 to 1986, he was a Professor at the Stevens Institute of Technology. From 1986 to 1996, he was a Professor at the College of Staten Island, City University of New York. From 1996, he was with Bell Telephone Laboratories, Whippany, NJ, USA. His research interests include digital signal and image processing, pattern recognition, artificial intelligence, and the constructive theory of functions. Dr. Giardina has authored numerous papers in these areas, and several books including, Mathematical Models for Artificial Intelligence and Autonomous Systems, Prentice Hall; Matrix Structure Image Processing, Prentice Hall; Parallel Digital Signal Processing: A Unified Signal Algebra Approach, Regency; Morphological Methods in Image and Signal Processing, Prentice Hall; Image Processing – Continuous to Discrete: Geometric, Transform, and Statistical Methods, Prentice Hall; and A Unified Signal Algebra Approach to Two-Dimensional Parallel Digital Signal Processing, Chapman and Hall/CRC Press.