SUSTAINABLE DEVELOPMENT
Innovate. Sustain. Transform.
Save up to 30% on top Physical Sciences & Engineering titles!
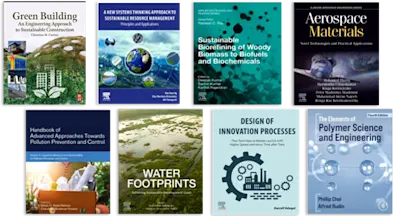
Predictive Modeling of Drug Sensitivity gives an overview of drug sensitivity modeling for personalized medicine that includes data characterizations, modeling technique… Read more
SUSTAINABLE DEVELOPMENT
Save up to 30% on top Physical Sciences & Engineering titles!
Predictive Modeling of Drug Sensitivity gives an overview of drug sensitivity modeling for personalized medicine that includes data characterizations, modeling techniques, applications, and research challenges. It covers the major mathematical techniques used for modeling drug sensitivity, and includes the requisite biological knowledge to guide a user to apply the mathematical tools in different biological scenarios.
This book is an ideal reference for computer scientists, engineers, computational biologists, and mathematicians who want to understand and apply multiple approaches and methods to drug sensitivity modeling. The reader will learn a broad range of mathematical and computational techniques applied to the modeling of drug sensitivity, biological concepts, and measurement techniques crucial to drug sensitivity modeling, how to design a combination of drugs under different constraints, and the applications of drug sensitivity prediction methodologies.
Computer scientists, engineers, computational biologists, and mathematicians
Chapter 1: Introduction
Chapter 2: Data characterization
Chapter 3: Feature selection and extraction from heterogeneous genomic characterizations
Chapter 4: Validation methodologies
Chapter 5: Tumor growth models
Chapter 6: Overview of predictive modeling based on genomic characterizations
Chapter 7: Predictive modeling based on random forests
Chapter 8: Predictive modeling based on multivariate random forests
Chapter 9: Predictive modeling based on functional and genomic characterizations
Chapter 10: Inference of dynamic biological networks based on perturbation data
Chapter 11: Combination therapeutics
Chapter 12: Online resources
Chapter 13: Challenges
RP