AI & BIG DATA
Shaping today's innovations
Save up to 25% on AI & Big Data books, eBooks & Journals
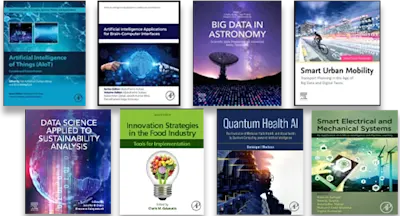
Practical Data Analytics for Innovation in Medicine: Building Real Predictive and Prescriptive Models in Personalized Healthcare and Medical Research Using AI, ML, and Relat… Read more
AI & BIG DATA
Save up to 25% on AI & Big Data books, eBooks & Journals
Practical Data Analytics for Innovation in Medicine: Building Real Predictive and Prescriptive Models in Personalized Healthcare and Medical Research Using AI, ML, and Related Technologies, Second Edition discusses the needs of healthcare and medicine in the 21st century, explaining how data analytics play an important and revolutionary role. With healthcare effectiveness and economics facing growing challenges, there is a rapidly emerging movement to fortify medical treatment and administration by tapping the predictive power of big data, such as predictive analytics, which can bolster patient care, reduce costs, and deliver greater efficiencies across a wide range of operational functions.
Sections bring a historical perspective, highlight the importance of using predictive analytics to help solve health crisis such as the COVID-19 pandemic, provide access to practical step-by-step tutorials and case studies online, and use exercises based on real-world examples of successful predictive and prescriptive tools and systems. The final part of the book focuses on specific technical operations related to quality, cost-effective medical and nursing care delivery and administration brought by practical predictive analytics.
GM
LM
SB
MG
RN
NW
TH