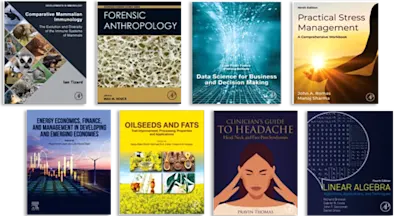
Physics-Aware Machine Learning for Integrated Energy Systems Management
- 1st Edition - August 1, 2025
- Imprint: Elsevier
- Editors: Mohammadreza Daneshvar, Behnam Mohammadi-Ivatloo, Kazem Zare, Jamshid Aghaei
- Language: English
- Paperback ISBN:9 7 8 - 0 - 4 4 3 - 3 2 9 8 4 - 5
- eBook ISBN:9 7 8 - 0 - 4 4 3 - 3 2 9 8 5 - 2
Physics-Aware Machine Learning for Integrated Energy Systems Management, a new release in the Advances in Intelligent Energy Systems series, guides the reader through this state-… Read more
Purchase options
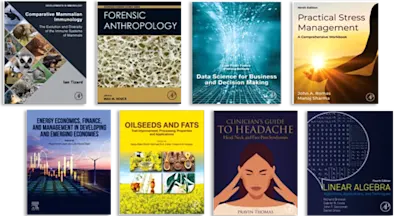
Institutional subscription on ScienceDirect
Request a sales quotePhysics-Aware Machine Learning for Integrated Energy Systems Management, a new release in the Advances in Intelligent Energy Systems series, guides the reader through this state-of-the-art approach to computational methods, from data input and training to application opportunities in integrated energy systems. The book begins by establishing the principles, design, and needs of integrated energy systems in the modern sustainable grid before moving into assessing aspects such as sustainability, energy storage, and physical-economic models. Detailed, step-by-step procedures for utilizing a variety of physics-aware machine learning models are provided, including reinforcement learning, feature learning, and neural networks.
Supporting students, researchers, and industry engineers to make renewable-integrated grids a reality, this book is a holistic introduction to an exciting new approach in energy systems management.
Supporting students, researchers, and industry engineers to make renewable-integrated grids a reality, this book is a holistic introduction to an exciting new approach in energy systems management.
- Outlines the challenges, opportunities, and applications for utilizing physics-aware machine learning to support renewable energy integration to the modern grid
- Covers a wide variety of techniques, from fundamental principles to security concerns
- Represents the latest offering in the cutting-edge series, Advances in Intelligent Energy Systems, which introduces these essential multidisciplinary skills to modern energy engineers
Upper-level undergraduate and graduate students, researchers, and engineers working in modern and sustainable energy systems
1. Introduction
2. The Need for Integrated Energy Systems Management
3. Attributes of Integrated Energy Systems in Modern Energy Grids
4. Physical-economic Models for Integrated Energy Systems Management
5. Decision-making Tools for the Optimal Operation and Planning of Integrated Energy Systems
6. Energy Storage Systems for Integrated Energy Systems Management
7. Applicability of Machine Learning Techniques in Managing Integrated Energy Systems
8. Physics-aware Machine Learning for Integrated Energy Systems Management
9. Physics-aware Machine Learning for Improving the Sustainability of Integrated Energy Systems
10. Physics-aware Machine Learning for Cyber-security Assessment of Integrated Energy Systems Management
11. Physics-aware Reinforcement Learning for Integrated Energy Systems Management
12. Physics-aware Feature Learning for Integrated Energy Systems Management
13. Physics-aware Neural Networks for Integrated Energy Systems Management
14. Physics-aware Machine Learning for Integrated Energy Interaction Management
2. The Need for Integrated Energy Systems Management
3. Attributes of Integrated Energy Systems in Modern Energy Grids
4. Physical-economic Models for Integrated Energy Systems Management
5. Decision-making Tools for the Optimal Operation and Planning of Integrated Energy Systems
6. Energy Storage Systems for Integrated Energy Systems Management
7. Applicability of Machine Learning Techniques in Managing Integrated Energy Systems
8. Physics-aware Machine Learning for Integrated Energy Systems Management
9. Physics-aware Machine Learning for Improving the Sustainability of Integrated Energy Systems
10. Physics-aware Machine Learning for Cyber-security Assessment of Integrated Energy Systems Management
11. Physics-aware Reinforcement Learning for Integrated Energy Systems Management
12. Physics-aware Feature Learning for Integrated Energy Systems Management
13. Physics-aware Neural Networks for Integrated Energy Systems Management
14. Physics-aware Machine Learning for Integrated Energy Interaction Management
- Edition: 1
- Published: August 1, 2025
- No. of pages (Paperback): 300
- Imprint: Elsevier
- Language: English
- Paperback ISBN: 9780443329845
- eBook ISBN: 9780443329852
MD
Mohammadreza Daneshvar
Mohammadreza Daneshvar is a Research Associate with the Smart Energy Systems Lab in the Department of Electrical and Computer Engineering at the University of Tabriz. He is the editor of more than 40 journal and conference papers in the field of multi-energy systems, grid modernization, transactive energy, and optimizing the multi-carrier energy grids. He is the author and editor of three books with Springer, Elsevier, and Wiley-IEEE. He serves as an active reviewer with IEEE, Elsevier, Springer, Wiley, Taylor & Francis, and IOS Press, and was ranked among the top 1% of reviewers in Engineering and Cross-Field based on Publons global reviewer database. His research interests include smart grids, transactive energy, energy management, renewable energy sources, multi-carrier energy systems, grid modernization, electrical energy storage systems, microgrids, energy hubs, machine learning and deep learning, blockchain technology, and optimization techniques.
Affiliations and expertise
Research Assistant, Smart Energy Systems Lab in Electrical Power Systems Engineering at the University of Tabriz, IranBM
Behnam Mohammadi-Ivatloo
Behnam Mohammadi-Ivatloo, Ph.D., is a Professor with the Faculty of Electrical and Computer Engineering, University of Tabriz, Tabriz, Iran. He was previously a Senior Research Fellow at Aalborg University, Denmark. Before joining the University of Tabriz, he was a research associate at the Institute for Sustainable Energy, Environment and Economy at the University of Calgary. He obtained MSc and Ph.D. degrees in electrical engineering from the Sharif University of Technology. His main research interests are renewable energies, microgrid systems, and smart grids. He has authored and co-authored more than 200 technical publications in his domain of interest, more than 30 book chapters, and 10 books.
Affiliations and expertise
Department of Electrical and Computer Engineering, University of Tabriz, Tabriz, IranKZ
Kazem Zare
Kazem Zare PhD, SMIEEE received the B.Sc. and M.Sc. degrees in electrical engineering from University of Tabriz, Tabriz, Iran, in 2000 and 2003, respectively, and Ph.D. degree from Tarbiat Modares University, Tehran, Iran, in 2009. Currently, he is an Associate Professor of the Faculty of Electrical and Computer Engineering, University of Tabriz. His research areas include distribution networks operation and planning, power system economics, microgrid and energy management.
Affiliations and expertise
Associate Professor, Faculty of Electrical and Computer Engineering, University of Tabriz, Tabriz, IranJA
Jamshid Aghaei
Jamshid Aghaei is currently a Full Professor with the School of Engineering and Technology at Central Queensland University, Australia. His research interests include smart grids, renewable energy systems, electricity markets, and power system operation, optimization, and planning. He was a Guest Editor of the Special Section on “Industrial and Commercial Demand Response” of the IEEE Transactions on Industrial Informatics, in November 2018, and the Special Issue on “Demand Side Management and Market Design for Renewable Energy Support and Integration” of the IET Renewable Power Generation, in April 2019. He is an Associate Editor of the IEEE Transactions on Smart Grid, IEEE Systems Journal, IEEE Transactions on Cloud Computing, IEEE Open Access Journal of Power and Energy, and IET Renewable Power Generation, and a Subject Editor of IET Generation Transmission and Distribution.
Affiliations and expertise
Professor, School of Engineering and Technology, Central Queensland University, Australia