Limited Offer
Save 50% on book bundles
Immediately download your ebook while waiting for your print delivery. No promo code needed.
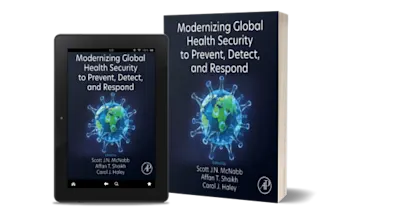
Personalized Predictive Modeling in Diabetes features state-of-the-art methodologies and algorithmic approaches which have been applied to predictive modeling of glucose concentra… Read more
Limited Offer
Personalized Predictive Modeling in Diabetes features state-of-the-art methodologies and algorithmic approaches which have been applied to predictive modeling of glucose concentration, ranging from simple autoregressive models of the CGM time series to multivariate nonlinear regression techniques of machine learning. Developments in the field have been analyzed with respect to: (i) feature set (univariate or multivariate), (ii) regression technique (linear or non-linear), (iii) learning mechanism (batch or sequential), (iv) development and testing procedure and (v) scaling properties. In addition, simulation models of meal-derived glucose absorption and insulin dynamics and kinetics are covered, as an integral part of glucose predictive models.
This book will help engineers and clinicians to: select a regression technique which can capture both linear and non-linear dynamics in glucose metabolism in diabetes, and which exhibits good generalization performance under stationary and non-stationary conditions; ensure the scalability of the optimization algorithm (learning mechanism) with respect to the size of the dataset, provided that multiple days of patient monitoring are needed to obtain a reliable predictive model; select a features set which efficiently represents both spatial and temporal dependencies between the input variables and the glucose concentration; select simulation models of subcutaneous insulin absorption and meal absorption; identify an appropriate validation procedure, and identify realistic performance measures.
Bioengineers, Clinicians, graduate and undergraduate students in the field of medicine and biomedical engineering
EG
DF
ST