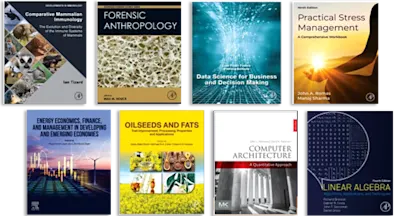
Nonlinear Ocean Dynamics
Synthetic Aperture Radar
- 1st Edition - February 12, 2021
- Imprint: Elsevier
- Author: Maged Marghany
- Language: English
- Paperback ISBN:9 7 8 - 0 - 1 2 - 8 2 0 7 8 5 - 7
- eBook ISBN:9 7 8 - 0 - 1 2 - 8 2 0 9 2 5 - 7
Nonlinear Ocean Dynamics: Synthetic Aperture Radar delivers the critical tools needed to understand the latest technology surrounding the radar imaging of nonlinear waves, pa… Read more
Purchase options
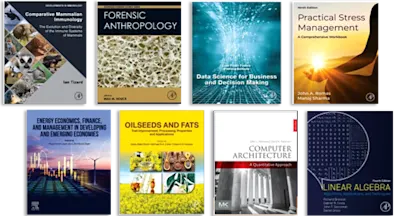
Nonlinear Ocean Dynamics: Synthetic Aperture Radar delivers the critical tools needed to understand the latest technology surrounding the radar imaging of nonlinear waves, particularly microwave radar, as a main source to understand, analyze and apply concepts in the field of ocean dynamic surface. Filling the gap between modern physics quantum theory and applications of radar imaging of ocean dynamic surface, this reference is packed with technical details associated with the potentiality of synthetic aperture radar (SAR). The book also includes key methods needed to extract the value-added information necessary, such as wave spectra energy, current pattern velocity, internal waves, and more. This book also reveals novel speculation of a shallow coastal front: named as Quantized Marghany's Front.
Rounding out with practical simulations of 4-D wave-current interaction patterns using using radar images, the book brings an effective new source of technology and applications for today’s coastal scientists and engineers.
- Solves specific problems surrounding the nonlinearity of ocean surface dynamics in synthetic aperture radar data
- Helps develop new algorithms for retrieving ocean wave spectra and ocean current movements from synthetic aperture radar
- Includes over 100 equations that illustrate how to follow examples in the book
Coastal Scientists and researchers, postgraduate students, Physical Oceanographers
- Edition: 1
- Published: February 12, 2021
- Imprint: Elsevier
- Language: English
MM
Maged Marghany
Distinguished Professor Dr. Maged Marghany, the visionary behind the innovative theory titled "Quantized Marghany’s Front," currently holds the esteemed position of Director at Global Geoinformation in Malaysia. Acknowledged globally for his exceptional contributions, Dr. Marghany achieved recognition by Stanford University, USA, by being listed among the top 2% of scientists for four consecutive years - 2020, 2021, 2022, and 2023. Furthermore, his profound impact is reflected in the recognition of two of his books, which were acknowledged among the best genetic algorithm books of all time. Dr. Marghany's ongoing commitment to excellence continues to shape the landscape of scientific thought and geoinformation expertise.
Additionally, Dr. Maged Marghany achieved the remarkable distinction of being ranked first among oil spill scientists in a global list spanning the last 50 years, compiled by the prestigious Universidade Estadual de Feira de Santana in Brazil. His expertise also extended to the role of a prominent visiting professor at Syiah Kuala University in Indonesia.
In previous roles, Dr. Marghany directed the Institute of Geospatial Applications at the University of Geomatica College. His educational journey includes a post-doctoral degree in radar remote sensing, a PhD in environmental remote sensing, and a Master of Science in physical oceanography. With over 250 papers and influential books," Dr. Marghany ‘s significant contributions shape global perspectives in remote sensing, geospatial applications, and environmental science.