Neural Systems for Control
- 1st Edition - February 12, 1997
- Authors: Omid Omidvar, David L. Elliott
- Language: English
- Hardback ISBN:9 7 8 - 0 - 1 2 - 5 2 6 4 3 0 - 3
- Paperback ISBN:9 7 8 - 0 - 1 2 - 3 9 9 2 4 8 - 2
- eBook ISBN:9 7 8 - 0 - 0 8 - 0 5 3 7 3 9 - 9
Control problems offer an industrially important application and a guide to understanding control systems for those working in Neural Networks. Neural Systems for Control repres… Read more
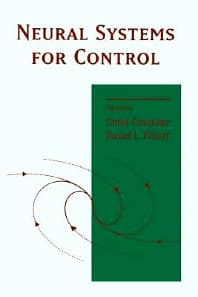
Purchase options
Institutional subscription on ScienceDirect
Request a sales quoteControl problems offer an industrially important application and a guide to understanding control systems for those working in Neural Networks. Neural Systems for Control represents the most up-to-date developments in the rapidly growing aplication area of neural networks and focuses on research in natural and artifical neural systems directly applicable to control or making use of modern control theory. The book covers such important new developments in control systems such as intelligent sensors in semiconductor wafer manufacturing; the relation between muscles and cerebral neurons in speech recognition; online compensation of reconfigurable control for spacecraft aircraft and other systems; applications to rolling mills, robotics and process control; the usage of past output data to identify nonlinear systems by neural networks; neural approximate optimal control; model-free nonlinear control; and neural control based on a regulation of physiological investigation/blood pressure control. All researchers and students dealing with control systems will find the fascinating Neural Systems for Control of immense interest and assistance.
- Focuses on research in natural and artifical neural systems directly applicable to contol or making use of modern control theory
- Represents the most up-to-date developments in this rapidly growing application area of neural networks
- Takes a new and novel approach to system identification and synthesis
Researchers and practicioners in the fields of neural networks, control engineering, mechanical engineering, and electrical engineering
Introduction: Neural Networks and Automatic Control. Reinforcement Learning. Neurocontrol in Sequence Recognition. A Learning Sensorimotor Map of Arm Movements: A Step Toward Biological Arm Control. Neuronal Modeling of the Baroceptor Reflex with Applications in Process Modeling and Control. Identification of Nonlinear Dynamical Systems Using Neural Networks. Neural Network Control of Robot Arms and Nonlinear Systems. Neual Networks for Intelligent Sensors and Control-PracticalIssues and Some Solutions. Approximation of Time-Optimal Control for an Industrial Production Plant with General Regression Neural Network. Neuro-Control Design: Reconfigurable Neural Control in Precision Space Structural Platforms. Neural Approximationsfor Finite- and Infinite-Horizon Optimal Control. Index.
- No. of pages: 358
- Language: English
- Edition: 1
- Published: February 12, 1997
- Imprint: Academic Press
- Hardback ISBN: 9780125264303
- Paperback ISBN: 9780123992482
- eBook ISBN: 9780080537399
OO
Omid Omidvar
Omid Omidvar is a professor of Computer Science at the University of Computer Science at the University of the District of Columbia, Washington, D.C. He is also a technical director of SPPARC center; a supercomputing facility funded by NSF. He received his Ph.D. from the University of Oklahoma in 1967 and has done extensive work in applications of Neural Networks in Optical Character Recognition and Finger Print for the National Institute of Standards and Technology. Dr. Omidvar has been a consultant to many of the world's most important corporations including IBM, Sun, Gumann, and has completed a five year project for the District of Columbia NASA Consortium in design and performance evaluation of neurocontrollers. Dr. Omidvar is also the Editor-in-Chief of the Journal of Artificial Neural Networks, has been an editor of Progress in Neural Network Series since 1990, and has published a large number of journal and conference publications. In addition to teaching, Dr. Omidvar is also currently working as a computer scientist in the Image Recognition Group, Advanced System Division, at NIST.
Affiliations and expertise
University of the District of ColumbiaDE
David L. Elliott
David Elliott learned automatic theory control as an applied mathematician at the Naval Ocean Systems Center and received his Ph.D. in Engineering from UCLA. His dissertation was the first to apply a differential geometric approach to stochastic nonlinear systems. Dr. Elliott helped to found the unique and well-known Department of Systems Science and Mathematics at Washington University, where he is now Professor Emeritus. Since 1992, he has been associated with the University of Maryland where he continues to advise doctoral students and perform research in nonlinear systems. His current research is supported by NeuroDyne, Inc., a company which develops new methods of system control and identification for government and industry. He has served as Associate Editor for the Control Society Newsletter, SIAM Review, Mathematical Systems Theor, and System and Control Letters, and is Associate Editor at Large for IEEE Transactions on automatic control. His research has recently been honored by advancement to Fellow of IEEE.
Affiliations and expertise
Institute of Systems Research, University of MarylandRead Neural Systems for Control on ScienceDirect