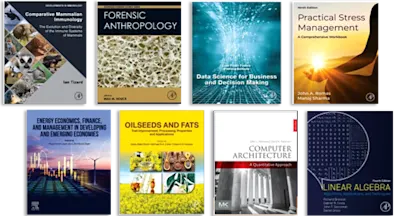
Neural Engineering Techniques for Autism Spectrum Disorder
Volume 1: Imaging and Signal Analysis
- 1st Edition - July 20, 2021
- Imprint: Academic Press
- Editors: Ayman S. El-Baz, Jasjit S. Suri
- Language: English
- Paperback ISBN:9 7 8 - 0 - 1 2 - 8 2 2 8 2 2 - 7
- eBook ISBN:9 7 8 - 0 - 1 2 - 8 2 3 0 6 5 - 7
Neural Engineering for Autism Spectrum Disorder, Volume One: Imaging and Signal Analysis Techniques presents the latest advances in neural engineering and biomedical engine… Read more
Purchase options
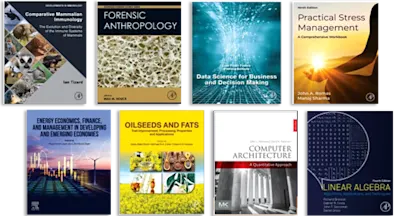
- Presents applications of Neural Engineering and other Machine Learning techniques for the diagnosis of Autism Spectrum Disorder (ASD)
- Includes in-depth technical coverage of imaging and signal analysis techniques, including coverage of functional MRI, neuroimaging, infrared spectroscopy, sMRI, fMRI, DTI, and neuroanatomy of autism
- Covers Signal Analysis for the detection and estimation of Autism Spectrum Disorder (ASD), including brain signal analysis, EEG analytics, feature selection, and analysis of blood oxygen level-dependent (BOLD) signals for ASD
- Written to help engineers, computer scientists, researchers and clinicians understand the technology and applications of Neural Engineering for the detection and diagnosis of Autism Spectrum Disorder (ASD)
Biomedical Engineers and researchers in neural engineering, medical imaging, and neural networks. Students, researchers and clinicians in autism, and a variety of other specialties
- Edition: 1
- Published: July 20, 2021
- Imprint: Academic Press
- Language: English
AS
Ayman S. El-Baz
JS
Jasjit S. Suri
Dr. Jasjit Suri, PhD, MBA, is a renowned innovator and scientist. He received the Director General’s Gold Medal in 1980 and is a Fellow of several prestigious organizations, including the American Institute of Medical and Biological Engineering and the Institute of Electrical and Electronics Engineers. Dr. Suri has been honored with lifetime achievement awards from Marcus, NJ, USA, and Graphics Era University, India. He has published nearly 300 peer-reviewed AI articles, 100 books, and holds 100 innovations/trademarks, achieving an H-index of nearly 100 with about 43,000 citations. Dr. Suri has served as chairman of AtheroPoint, IEEE Denver section, and as an advisory board member to various healthcare industries and universities globally.