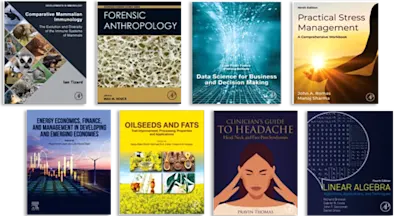
Modeling and Control of Dynamic Spatially Distributed Systems
Pharmaceutical Processes
- 1st Edition - November 8, 2024
- Imprint: Academic Press
- Authors: Yizhi Wang, Zhong Yang
- Language: English
- Paperback ISBN:9 7 8 - 0 - 3 2 3 - 9 5 3 9 2 - 4
- eBook ISBN:9 7 8 - 0 - 3 2 3 - 9 5 3 9 1 - 7
Modeling and Control of Dynamic Spatially Distributed Systems: Pharmaceutical Processes provides a balanced approach to help readers to get started quickly in the field of bioche… Read more
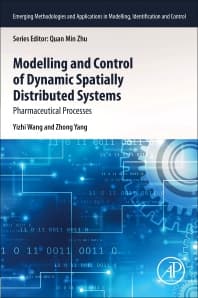
Purchase options
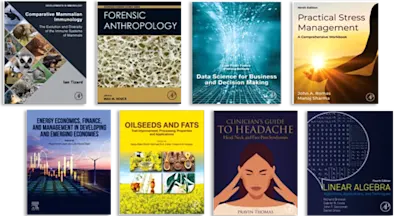
Institutional subscription on ScienceDirect
Request a sales quoteModeling and Control of Dynamic Spatially Distributed Systems: Pharmaceutical Processes provides a balanced approach to help readers to get started quickly in the field of biochemical pharmaceuticals. From a theoretical perspective, dynamic spatially distributed systems are introduced to address their industrial applications. After identifying problems, the book provides readers with modeling and control system design techniques via a novel fuzzy set (class of objects with a continuum of grades of membership, to describe the grade of the object belonging to this fuzzy set) and intelligent computation methods.
From an application perspective, the book provides a thorough understanding of Good Manufacture Practices (GMP) and the importance of identification, modelling, and intelligent control of such systems, reducing the test-and-error cost, and the R&D design time cycle of original drug development.
- Provides an updated, supplemental knowledge to the body of distributed parameter systems
- Covers control and analysis framework based on a state-space approach for a non-standard model from industrial complex systems
- Presents a novel proposed fuzzy set and applies it to case studies to illustrate its feasibility
- Includes a control system design solution from perspective of medicine production
PART 1 Background
1. Dynamic Spatially Distributed Systems: Pharmaceutical Processes
2. Fundamentals of Fuzzy Control
3. Pharmaceutical Engineering And Processes
PART 2 Modeling of Dynamic spatially Distributed systems
4. Introduction to Depyrogenation Tunnel
5. Conventional Methods in Modelling and Simulation of Depyrogenation Tunnel
6. Quasi-Guassian Fuzzy Sets and Approximation
7. Quasi-Guassian Fuzzy Systems and Applications
PART 3 Intelligent Control Methods Of Dynamic Spatially Distributed Systems
8. General Control methods of Depyrogenation Tunnel
9. Hierarchical Fuzzy Control Of Depyrogenation Tunnel
10. Conclusions and Future Prospects
- Edition: 1
- Published: November 8, 2024
- No. of pages (Paperback): 316
- Imprint: Academic Press
- Language: English
- Paperback ISBN: 9780323953924
- eBook ISBN: 9780323953917
YW
Yizhi Wang
Yizhi Wang received her Ph.D. degree in Control Engineering from the University of the West of England, received her M.Sc. degree in System Engineering from the University of South Australia and a B.Sc. degree in Chemical Engineering from Nanjing Forestry University. Yizhi served as an Associated Professor since 2024.8 and lecturer since 2018.7 in Discipline of Automation, College of Intelligent Science and Control Engineering, Jinling Institute of Technology and also a Post Doc. Fellow at Nanjing Agricultural University. She has served as Technical Vice President of Nanjing Leechdom Biopharm Co. Ltd in pharmaceutical process control and optimization since 2019.2. Her current research interest includes advanced fuzzy theory in evaluation, modeling, identification and control of intelligent manufacturing systems and unmanned agricultural equipment development.
ZY
Zhong Yang
Zhong Yang obtained his PhD in engineering from Nanjing University of Aeronautics and Astronautics in 1996 and did a 2-year research postdoc at Southeast University in Automatic Control until 1997. He is the chief investigator of several academic and teaching reform research projects funded by the National Natural Science Foundation of China (NSFC) and the Natural Science Foundation of Jiangsu Province. He has also received several highly recognized prizes, including the Second Prize of Science and Technology in China Machinery Industry. Currently, Prof. Yang serves as the dean of the College of Intelligent Science and Control Engineering, Jinling Institute of Technology, as well as the research degree supervisor. His research interests now focus on intelligence science, control engineering, solar energy photovoltaic generation, and optical scattering.