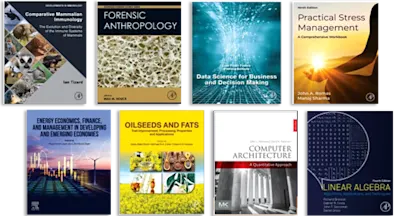
Mobile Edge Artificial Intelligence
Opportunities and Challenges
- 1st Edition - August 7, 2021
- Imprint: Academic Press
- Authors: Yuanming Shi, Kai Yang, Zhanpeng Yang, Yong Zhou
- Language: English
- Paperback ISBN:9 7 8 - 0 - 1 2 - 8 2 3 8 1 7 - 2
- eBook ISBN:9 7 8 - 0 - 1 2 - 8 2 3 8 3 5 - 6
Mobile Edge Artificial Intelligence: Opportunities and Challenges presents recent advances in wireless technologies and nonconvex optimization techniques for designing efficient… Read more
Purchase options
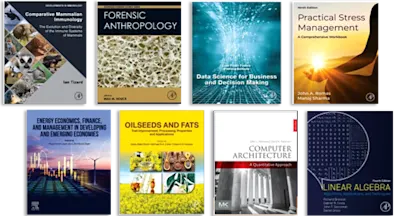
Mobile Edge Artificial Intelligence: Opportunities and Challenges presents recent advances in wireless technologies and nonconvex optimization techniques for designing efficient edge AI systems. The book includes comprehensive coverage on modeling, algorithm design and theoretical analysis. Through typical examples, the powerfulness of this set of systems and algorithms is demonstrated, along with their abilities to make low-latency, reliable and private intelligent decisions at network edge. With the availability of massive datasets, high performance computing platforms, sophisticated algorithms and software toolkits, AI has achieved remarkable success in many application domains.
As such, intelligent wireless networks will be designed to leverage advanced wireless communications and mobile computing technologies to support AI-enabled applications at various edge mobile devices with limited communication, computation, hardware and energy resources.
- Presents advanced key enabling techniques, including model compression, wireless MapReduce and wireless cooperative transmission
- Provides advanced 6G wireless techniques, including over-the-air computation and reconfigurable intelligent surface
- Includes principles for designing communication-efficient edge inference systems, communication-efficient training systems, and communication-efficient optimization algorithms for edge machine learning
I. Introduction and Overview
1. Primer on Artificial Intelligence
1.1. Basics of Machine Learning
1.1.1. Supervised Learning
1.1.2. Unsupervised Learning
1.1.3. Reinforcement Learning
1.2. Models of Deep Learning
1.2.1. Convolution Neural Network
1.2.2. Recurrent Neural Network
1.2.3. Graph Neural Network
1.2.4. Generative Adversarial Network
1.3. Model Training and Inference
2. Overview of Edge AI Systems
2.1. Motivations and Applications
2.2. Levels of Edge Intelligence
2.3. Edge Inference Process
2.3.1. Architectures
2.3.2. Performance Indicators
2.4. Edge Training Process
2.4.1. Architectures
2.4.2. Performance Indicators
II. Edge Inference
3. Model Compression for On-Device Inference
3.1. Problem Formulation
3.1.1. Layer-wise Pruning of Network
3.1.2. Nonconvex Pruning Approach
3.2. Inexact Proximal Iteratively Reweighted Algorithm
3.2.1. Construction of Convex Surrogate Functions
3.2.2. A Novel Termination Criterion
3.2.3. Implementation of iPIR Based on ADMM
3.2.4. Simulation Results
3.3. Summary
4. Wireless MapReduce for Device Distributed Inference
4.1. System Model
4.1.1. Computation Model
4.1.2. Communication Model
4.1.3. Achievable Data Rates and DoF
4.2. Interference Alignment for Data Shuffling
4.2.1. Interference Alignment Conditions
4.2.2. Low-Rank Optimization Approach
4.2.3. Problem Analysis
4.3. Difference-of-Convex Functions (DC) Programming for Low-Rank Optimization
4.3.1. Principles of DC Approach
4.3.2. A Novel DC Representation for Rank Function
4.3.3. Efficient DC Algorithm
4.3.4. Simulation Results
4.4. Summary
5. Wireless Cooperative Transmission for Edge Inference
5.1. System Model
5.1.1. Wireless Communication Model
5.1.2. Power Consumption Model
5.1.3. Channel Uncertainty Model
5.1.4. Problem Formulation
5.2. Learning based Robust Optimization Approximation for Joint Chance Constraints
5.2.1. Approximating Joint Chance Constraints via Robust Optimization
5.2.2. Learning the High Probability Region from Data Samples
5.2.3. Tractable Reformulations for Robust Optimization Problem
5.2.4. Cost-Effective Sampling Strategy
5.3. Reweighted Power Minimization for Quadratic Constrained Group Sparse Beamforming
5.3.1. Matrix Lifting for Nonconvex Quadratic Constraints
5.3.2. DC Representations for Rank-One Constraint
5.3.3. Reweighted Algorithm for Inducing Group Sparsity
5.3.4. Proposed Reweighted Power Minimization Approach
5.3.5. Simulation Results
5.4. Summary
III. Edge Training
6. Over-the-Air Computation for Federated Learning
6.1. System Model
6.1.1. On-Device Distributed Federated Learning
6.1.2. Over-the-Air Computation for Model Aggregation
6.1.3. Problem Formulation
6.2. Sparse and Low-Rank Optimization for Federated Learning
6.2.1. Sparse and Low-Rank Optimization
6.2.2. Problem Analysis
6.3. Difference-of-Convex Functions (DC) Representations
6.3.1. DC Representation for Sparse Function
6.3.2. DC Representation for Low-Rank Constraint
6.3.3. A Unified DC Representation Framework
6.3.4. DC Algorithms for Sparse and Low-Rank Optimization
6.3.5. Simulation Results
6.4. Summary
7. Blind Over-the-Air Computation for Federated Learning
7.1. Problem Formulation
7.1.1. Blind Over-the-Air Computation
7.1.2. Multi-Dimensional Nonconvex Estimation
7.2. Main Approach
7.2.1. Randomly Initialized Wirtinger Flow Algorithm
7.2.2. Theoretical Analysis
7.2.3. Simulation Results
7.3. Summary
8. Reconfigurable Intelligent Surface Aided Federated Learning System
8.1. System Model
8.1.1. Reconfigurable Intelligent Surface (RIS)-Aided Federated Learning System
8.1.2. Problem Formulation
8.2. Alternating Low-Rank Optimization for Model Aggregation
8.2.1. A Two-Stage Framework
8.2.2. Alternating Low-Rank Optimization
8.2.3. Difference-of-Convex Functions Programming Algorithm
8.2.4.Simulation Results
8.3. Summary
IV. Future Directions
9. Communication-Efficient Algorithms for Edge AI
9.1. Communication-Efficient Zeroth-Order Methods
9.2. Communication-Efficient First-Order Methods
9.3. Communication-Efficient Second-order Methods
9.4. Communication-Efficient Federated Optimization
10. Future Research Directions
10.1. Edge AI Hardware Design
10.2. Edge AI Software Platforms
10.3. Edge AI as a Service
10.4. Security and Privacy Issues
- Edition: 1
- Published: August 7, 2021
- Imprint: Academic Press
- Language: English
YS
Yuanming Shi
KY
Kai Yang
ZY
Zhanpeng Yang
YZ