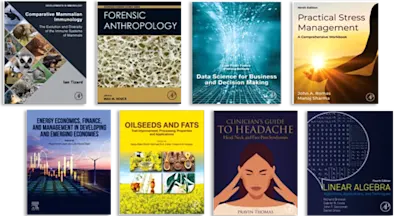
Medical Image Recognition, Segmentation and Parsing
Machine Learning and Multiple Object Approaches
- 1st Edition - December 2, 2015
- Imprint: Academic Press
- Author: S. Kevin Zhou
- Language: English
- Hardback ISBN:9 7 8 - 0 - 1 2 - 8 0 2 5 8 1 - 9
- eBook ISBN:9 7 8 - 0 - 1 2 - 8 0 2 6 7 6 - 2
This book describes the technical problems and solutions for automatically recognizing and parsing a medical image into multiple objects, structures, or anatomies. It gives all th… Read more
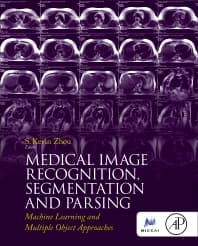
Purchase options
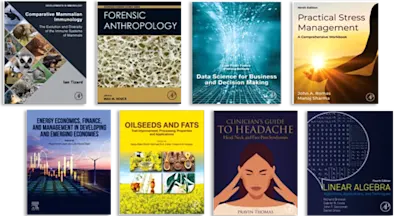
Institutional subscription on ScienceDirect
Request a sales quoteThis book describes the technical problems and solutions for automatically recognizing and parsing a medical image into multiple objects, structures, or anatomies. It gives all the key methods, including state-of- the-art approaches based on machine learning, for recognizing or detecting, parsing or segmenting, a cohort of anatomical structures from a medical image.
Written by top experts in Medical Imaging, this book is ideal for university researchers and industry practitioners in medical imaging who want a complete reference on key methods, algorithms and applications in medical image recognition, segmentation and parsing of multiple objects.
Learn:
- Research challenges and problems in medical image recognition, segmentation and parsing of multiple objects
- Methods and theories for medical image recognition, segmentation and parsing of multiple objects
- Efficient and effective machine learning solutions based on big datasets
- Selected applications of medical image parsing using proven algorithms
- Provides a comprehensive overview of state-of-the-art research on medical image recognition, segmentation, and parsing of multiple objects
- Presents efficient and effective approaches based on machine learning paradigms to leverage the anatomical context in the medical images, best exemplified by large datasets
- Includes algorithms for recognizing and parsing of known anatomies for practical applications
Industry practitioners and university researchers in medical imaging.
- Foreword
- Acknowledgments
- Chapter 1: Introduction to Medical Image Recognition, Segmentation, and Parsing
- Abstract
- 1.1 Introduction
- 1.2 Challenges and Opportunities
- 1.3 Rough-to-Exact Object Representation
- 1.4 Simple-to-Complex Probabilistic Modeling
- 1.5 Medical Image Recognition Using Machine Learning Methods
- 1.6 Medical Image Segmentation Methods
- 1.7 Conclusions
- Recommended Notations
- Part 1: Automatic Recognition and Detection Algorithms
- Chapter 2: A Survey of Anatomy Detection
- Abstract
- 2.1 Introduction
- 2.2 Methods for Detecting an Anatomy
- 2.3 Methods for Detecting Multiple Anatomies
- 2.4 Conclusions
- Chapter 3: Robust Multi-Landmark Detection Based on Information Theoretic Scheduling
- Abstract
- 3.1 Introduction
- 3.2 Literature Review
- 3.3 Methods
- 3.4 Applications
- 3.5 Conclusion
- Chapter 4: Landmark Detection Using Submodular Functions
- Abstract
- 4.1 Introduction
- 4.2 Multiple Landmark Detection
- 4.3 Finding the Anchor Landmark
- 4.4 Coarse-to-Fine Detection
- 4.5 Discussion
- 4.6 Summary
- Chapter 5: Random Forests for Localization of Spinal Anatomy
- Abstract
- 5.1 Introduction
- 5.2 Anatomy Localization Using Random Forests
- 5.3 Experimental Comparison
- 5.4 Conclusion
- Chapter 6: Integrated Detection Network for Multiple Object Recognition
- Abstract
- 6.1 Introduction
- 6.2 Independent Multiobject Recognition
- 6.3 Sequential Sampling for Multiobject Recognition
- 6.4 Applications
- 6.5 Conclusions
- Chapter 7: Organ Detection Using Deep Learning
- Abstract
- Acknowledgments
- 7.1 Introduction
- 7.2 Related Literature
- 7.3 Methods
- 7.4 Experiments
- 7.5 Conclusions
- Chapter 2: A Survey of Anatomy Detection
- Part 2: Automatic Segmentation and Parsing Algorithms
- Chapter 8: A Probabilistic Framework for Multiple Organ Segmentation Using Learning Methods and Level Sets
- Abstract
- 8.1 Introduction
- 8.2 Literature Review
- 8.3 Proposed Method
- 8.4 Experimental Results
- 8.5 Conclusions
- Chapter 9: LOGISMOS: A Family of Graph-Based Optimal Image Segmentation Methods
- Abstract
- Acknowledgments
- 9.1 Introduction
- 9.2 Layered Optimal Graph Image Segmentation of Multiple Objects and Surfaces
- 9.3 Multiobject Multisurface LOGISMOS for Knee Joint Segmentation
- 9.4 Multisurface Multiimage Co-Segmentation: Retinal OCT
- 9.5 Complex Multisurface Geometry: LOGISMOS-B for Brain Cortex
- 9.6 Future Directions
- Chapter 10: A Context Integration Framework for Rapid Multiple Organ Parsing
- Abstract
- 10.1 Introduction
- 10.2 Related Literature
- 10.3 Methods
- 10.4 Object Context
- 10.5 Automatic Mesh Vertex Selection
- 10.6 Incomplete Annotations
- 10.7 Experiments
- 10.8 Conclusions
- Chapter 11: Multiple-Atlas Segmentation in Medical Imaging
- Abstract
- 11.1 Introduction
- 11.2 Atlas Selection
- 11.3 Image Registration
- 11.4 Label Fusion
- 11.5 Conclusions
- Chapter 12: An Overview of the Multi-Object Geometric Deformable Model Approach in Biomedical Imaging
- Abstract
- Acknowledgments
- 12.1 Introduction
- 12.2 Methods
- 12.3 Segmentation of a Multi-Object Hand
- 12.4 Applications
- 12.5 Discussion and Conclusion
- Chapter 13: Robust and Scalable Shape Prior Modeling via Sparse Representation and Dictionary Learning
- Abstract
- 13.1 Introduction
- 13.2 Related Work
- 13.3 Segmentation Framework
- 13.4 Sparse Shape Composition
- 13.5 Dictionary Learning for Compact Representations
- 13.6 Mesh Partition for Local Sparse Shape Composition
- 13.7 Discussion
- Chapter 8: A Probabilistic Framework for Multiple Organ Segmentation Using Learning Methods and Level Sets
- Part 3: Recognition, Segmentation and Parsing of Specific Objects
- Chapter 14: Semantic Parsing of Brain MR Images
- Abstract
- 14.1 Introduction
- 14.2 Atlas-Based Segmentation Methods
- 14.3 Brain Atlases From MR Images
- 14.4 Conclusions
- Chapter 15: Parsing of the Lungs and Airways
- Abstract
- 15.1 Introduction
- 15.2 Overview
- 15.3 Lung and Airway Segmentation
- 15.4 Airway Tree Parsing
- 15.5 Lobar Segmentation
- 15.6 Quantification of Airway Dimensions
- 15.7 Applications
- 15.8 Conclusion
- Chapter 16: Aortic and Mitral Valve Modeling From Multi-Modal Image Data
- Abstract
- 16.1 Introduction
- 16.2 Physiological Model of the Heart Valves
- 16.3 Patient-Specific Model Parameter Estimation
- 16.4 Experimental Results
- 16.5 Conclusions
- Chapter 17: Model-Based 3D Cardiac Image Segmentation With Marginal Space Learning
- Abstract
- Acknowledgments
- 17.1 Introduction
- 17.2 Marginal Space Learning for 3D Object Segmentation
- 17.3 Cardiac Chamber Segmentation
- 17.4 Great Vessel Segmentation
- 17.5 Coronary Artery Segmentation
- 17.6 Experiments
- 17.7 Conclusions and Future Work
- Chapter 18: Spine Disk and RIB Centerline Parsing
- Abstract
- 18.1 Introduction
- 18.2 Related Work
- 18.3 Spine Disk Parsing
- 18.4 RIB Centerline Parsing
- 18.5 Conclusions
- Chapter 19: Data-Driven Detection and Segmentation of Lymph Nodes
- Abstract
- 19.1 Introduction
- 19.2 Related Work
- 19.3 LN Center Candidate Detection
- 19.4 Segmentation-Based Verification
- 19.5 Spatial Prior
- 19.6 Experiments
- 19.7 Conclusion
- Chapter 20: Polyp Segmentation on CT Colonography
- Abstract
- Acknowledgments
- 20.1 Colonic Polyp and Colon Cancer
- 20.2 CT Colonography
- 20.3 Computer-Aided Detection and Diagnosis on CTC
- 20.4 Polyp Segmentation
- 20.5 Polyp Measurement and Characterization
- 20.6 Data Acquisition and Validation Experiment
- 20.7 Results
- 20.8 Discussion
- Chapter 21: Detect Cells and Cellular Behaviors in Phase Contrast Microscopy Images
- Abstract
- 21.1 Introduction
- 21.2 Computer Vision Tasks in Analyzing Cell Populations
- 21.3 Cell Segmentation
- 21.4 Cellular Behavior Understanding
- 21.5 Systems for Analyzing Cell Populations in Time-Lapse Imaging
- 21.6 Open Source Cell Image Sequence Data
- Chapter 14: Semantic Parsing of Brain MR Images
- Index
- Edition: 1
- Published: December 2, 2015
- No. of pages (Hardback): 542
- No. of pages (eBook): 542
- Imprint: Academic Press
- Language: English
- Hardback ISBN: 9780128025819
- eBook ISBN: 9780128026762
SZ