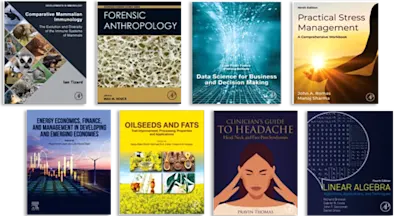
Medical Image Analysis
- 1st Edition - September 20, 2023
- Imprint: Academic Press
- Editors: Alejandro Frangi, Jerry Prince, Milan Sonka
- Language: English
- Paperback ISBN:9 7 8 - 0 - 1 2 - 8 1 3 6 5 7 - 7
- eBook ISBN:9 7 8 - 0 - 1 2 - 8 1 3 6 5 8 - 4
Medical imaging is increasingly at the base of many breakthroughs in biomedical sciences, becoming a fundamental enabling technology of biomedical scientific progress. Medical Im… Read more
Purchase options
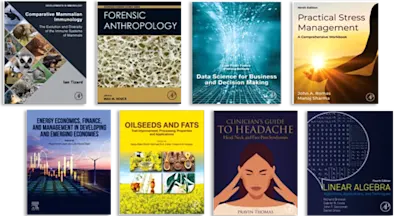
Institutional subscription on ScienceDirect
Request a sales quote- An authoritative presentation of key concepts and methods from experts in the field
- Sections clearly explaining key methodological principles within relevant medical applications
- Self-contained chapters enable the text to be used on courses with differing structures
- A representative selection of modern topics and techniques in medical image computing
- Focus on medical image computing as an enabling technology to tackle unmet clinical needs
- Presentation of traditional and machine learning approaches to medical image computing
PART I Introductory topics
1. Medical imaging modalities
Mathias Unberath and Andreas Maier
1.1 Introduction
1.2 Image quality
1.3 Modalities and contrast mechanisms
1.4 Clinical scenarios
1.5 Exercises
References
2. Mathematical preliminaries
Carlos Alberola-López and Alejandro F. Frangi
2.1 Introduction
2.2 Imaging: definitions, quality and similarity measures
2.3 Vector and matrix theory results
2.4 Linear processing and transformed domains
2.5 Calculus
2.6 Notions on shapes
2.7 Exercises
References
3. Regression and classification
Thomas Moreau and Demian Wassermann
3.1 Introduction
Nomenclature
3.2 Multidimensional linear regression
3.3 Treating non-linear problems with linear models
3.4 Exercises
References
4. Estimation and inference
Gonzalo Vegas Sánchez-Ferrero and Carlos Alberola-López
4.1 Introduction: what is estimation?
4.2 Sampling distributions
4.3 Estimation. Data-based methods
4.4 A working example
4.5 Estimation. Bayesian methods
4.6 Monte Carlo methods
4.7 Exercises
References
PART II Image representation and processing
5. Image representation and 2D signal processing
Santiago Aja-Fernández, Gabriel Ramos-Llordén, and Paul A. Yushkevich
5.1 Image representation
5.2 Images as 2D signals
5.3 Frequency representation of 2D signals
5.4 Image sampling
5.5 Image interpolation
5.6 Image quantization
5.7 Further reading
5.8 Exercises
References
6. Image filtering: enhancement and restoration
Santiago Aja-Fernández, Ariel H. Curiale, and Jerry L. Prince
6.1 Medical imaging filtering
6.2 Point-to-point operations
6.3 Spatial operations
6.4 Operations in the transformdomain
6.5 Model-based filtering: image restoration
6.6 Further reading
6.7 Exercises
References
7. Multiscale and multiresolution analysis
Jon Sporring
7.1 Introduction
7.2 The image pyramid
7.3 The Gaussian scale-space
7.4 Properties of the Gaussian scale-space
7.5 Scale selection
7.6 The scale-space histogram
7.7 Exercises
References
PART III Medical image segmentation
8. Statistical shape models
Tim Cootes
8.1 Introduction
8.2 Representing structures with points
8.3 Comparing shapes
8.4 Aligning two shapes
8.5 Aligning a set of shapes
8.6 Building shape models
8.7 Statistical models of texture
8.8 Combined models of appearance (shape and texture)
8.9 Image search
8.10 Exhaustive search
8.11 Alternating approaches
8.12 Constrained local models
8.13 3D models
8.14 Recapitulation
8.15 Exercises
References
9. Segmentation by deformable models
Jerry L. Prince
9.1 Introduction
9.2 Boundary evolution
9.3 Forces and speed functions
9.4 Numerical implementation
9.5 Other considerations
9.6 Recapitulation
9.7 Exercises
References
10. Graph cut-based segmentation
Jens Petersen, Ipek Oguz, and Marleen de Bruijne
10.1 Introduction
10.2 Graph theory
10.3 Modeling image segmentation using Markov random fields
10.4 Energy function, image term, and regularization term
10.5 Graph optimization and necessary conditions
10.6 Interactive segmentation
10.7 More than two labels
10.8 Recapitulation
10.9 Exercises
References
PART IV Medical image registration
11. Points and surface registration
Shan Cong and Li Shen
11.1 Introduction
11.2 Points registration
11.3 Surface registration
11.4 Summary
11.5 Exercises
References
12. Graph matching and registration
Aristeidis Sotiras, Mattias Heinrich, Julia Schnabel, and Nikos Paragios
12.1 Introduction
12.2 Graph-based image registration
12.3 Exercises
References
13. Parametric volumetric registration
Paul A. Yushkevich, Miaomiao Zhang, and Jon Sporring
13.1 Introduction to volumetric registration
13.2 Mathematical concepts
13.3 Parametric volumetric registration
13.4 Exercises
References
14. Non-parametric volumetric registration
Paul A. Yushkevich and Miaomiao Zhang
14.1 Introduction
14.2 Mathematical concepts
14.3 Optical flow and related non-parametric methods
14.4 Large deformation diffeomorphic metric mapping
14.5 Exercises
References
15. Image mosaicking
Sophia Bano and Danail Stoyanov
15.1 Introduction
15.2 Motion models
15.3 Matching
15.4 Clinical applications
15.5 Recapitulation
15.6 Exercises
References
PART V Machine learning in medical image analysis
16. Deep learning fundamentals
Nishant Ravikumar, Arezoo Zakeri, Yan Xia, and Alejandro F. Frangi
16.1 Introduction
16.2 Learning as optimization
16.3 Inductive bias, invariance, and equivariance
16.4 Recapitulation
16.5 Further reading
16.6 Exercises
References
17. Deep learning for vision and representation learning
Arezoo Zakeri, Yan Xia, Nishant Ravikumar, and Alejandro F. Frangi
17.1 Introduction
17.2 Convolutional neural networks
17.3 Deep representation learning
17.4 Recapitulation
17.5 Further reading
17.6 Exercises
References
18. Deep learning medical image segmentation
Sean Mullan, Lichun Zhang, Honghai Zhang, and Milan Sonka
18.1 Introduction
18.2 Convolution-based deep learning segmentation
18.3 Transformer-based deep learning segmentation
18.4 Hybrid deep learning segmentation
18.5 Training efficiency
18.6 Explainability
18.7 Case study
18.8 Recapitulation
18.9 Further reading
18.10 Exercises
References
19. Machine learning in image registration
Bob D. de Vos, Hessam Sokooti, Marius Staring, and Ivana Išgum
19.1 Introduction
19.2 Image registration with deep learning
19.3 Deep neural network architecture
19.4 Supervised image registration
19.5 Unsupervised image registration
19.6 Recapitulation
19.7 Exercises
References
PART VI Advanced topics in medical image analysis
20. Motion and deformation recovery and analysis
James S. Duncan and Lawrence H. Staib
20.1 Introduction
20.2 The unmet clinical need
20.3 Image-centric flow fields: Eulerian analysis
20.4 Object-centric, locally derived flow fields: Lagrangian Analysis
20.5 Multiframe analysis: Kalman filters, particle tracking
20.6 Advanced strategies: model-based analysis and data-driven deep learning
20.7 Evaluation
20.8 Recapitulation
20.9 Exercises
References
21. Imaging Genetics
Marco Lorenzi and Andre Altmann
21.1 Introduction
21.2 Genome-wide association studies
21.3 Multivariate approaches to imaging genetics
21.4 Exercises
References
PART VII Large-scale databases
22. Detection and quantitative enumeration of objects from large images
Cheng Lu, Simon Graham, Nasir Rajpoot, and Anant Madabhushi
22.1 Introduction
22.2 Classical image analysis methods
22.3 Learning from data
22.4 Detection and counting of mitotic cells using Bayesian modeling and classical image processing
22.5 Detection and counting of nuclei using deep learning
22.6 Recapitulation
22.7 Exercises
References
23. Image retrieval in big image data
Sailesh Conjeti, Stefanie Demirci, and Vincent Christlein
23.1 Introduction
23.2 Global image descriptors for image retrieval
23.3 Deep learning-based image retrieval
23.4 Efficient indexing strategies
23.5 Exercises
References
PART VIII Evaluation in medical image analysis
24. Assessment of image computing methods
Ipek Oguz, Melissa Martin, and Russell T. Shinohara
24.1 The fundamental methodological concept
24.2 Introduction
24.3 Evaluation for classification tasks
24.4 Learning and validation
24.5 Evaluation for segmentation tasks
24.6 Evaluation of registration tasks
24.7 Intra-rater and inter-rater comparisons
24.8 Recapitulation
24.9 Exercises
References
- Edition: 1
- Published: September 20, 2023
- No. of pages (Paperback): 698
- Imprint: Academic Press
- Language: English
- Paperback ISBN: 9780128136577
- eBook ISBN: 9780128136584
AF
Alejandro Frangi
JP
Jerry Prince
MS