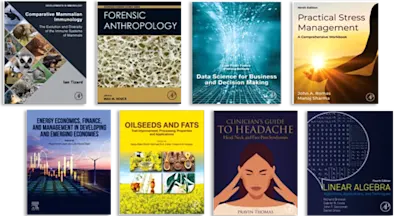
Magnetic Resonance Image Reconstruction
Theory, Methods, and Applications
- 1st Edition, Volume 7 - November 4, 2022
- Imprint: Academic Press
- Editors: Mehmet Akcakaya, Mariya Ivanova Doneva, Claudia Prieto
- Language: English
- Paperback ISBN:9 7 8 - 0 - 1 2 - 8 2 2 7 2 6 - 8
- eBook ISBN:9 7 8 - 0 - 1 2 - 8 2 2 7 4 6 - 6
Magnetic Resonance Image Reconstruction: Theory, Methods and Applications presents the fundamental concepts of MR image reconstruction, including its formulation as an invers… Read more
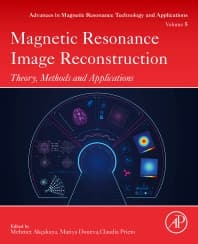
Purchase options
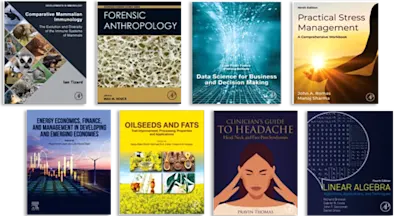
Institutional subscription on ScienceDirect
Request a sales quote- Explains the underlying principles of MRI reconstruction, along with the latest research<
- Gives example codes for some of the methods presented
- Includes updates on the latest developments, including compressed sensing, tensor-based reconstruction and machine learning based reconstruction
PART 1 Basics of MRI Reconstruction
1. Brief introduction to MRI physics
2. MRI reconstruction as an inverse problem
3. Optimization algorithms for MR reconstruction
4. Non-Cartesian MRI reconstruction
5. “Early” constrained reconstruction methods
PART 2 Reconstruction of undersampled MRI data
6. Parallel imaging
7. Simultaneous multislice reconstruction
8. Sparse reconstruction
9. Low-rank matrix and tensor–based reconstruction
10. Dictionary, structured low-rank, and manifold learning-based reconstruction
11. Machine learning for MRI reconstruction
PART 3 Reconstruction methods for nonlinear forward models in MRI
12. Imaging in the presence of magnetic field inhomogeneities
13. Motion-corrected reconstruction
14. Chemical shift encoding-based water-fat separation
15. Model-based parametric mapping reconstruction
16. Quantitative susceptibility-mapping reconstruction
APPENDIX A Linear algebra primer
- Edition: 1
- Volume: 7
- Published: November 4, 2022
- No. of pages (Paperback): 516
- Imprint: Academic Press
- Language: English
- Paperback ISBN: 9780128227268
- eBook ISBN: 9780128227466
MA
Mehmet Akcakaya
MD
Mariya Ivanova Doneva
CP