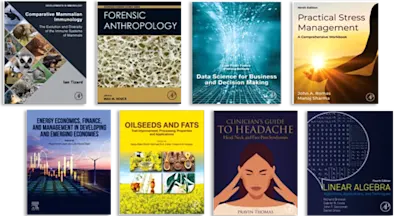
Machine Learning Solutions for Inverse Problems: Part A
- 1st Edition, Volume 26 - October 1, 2025
- Imprint: Academic Press
- Editors: Andreas Hauptmann, Michael Hintermüller, Bangti Jin, Carola-Bibiane Schönlieb
- Language: English
- Hardback ISBN:9 7 8 - 0 - 4 4 3 - 4 1 7 8 9 - 4
- eBook ISBN:9 7 8 - 0 - 4 4 3 - 4 1 7 9 0 - 0
Machine Learning Solutions for Inverse Problems: Part A, Volume 26 in the Handbook of Numerical Analysis, highlights new advances in the field, with this new volume presenting in… Read more
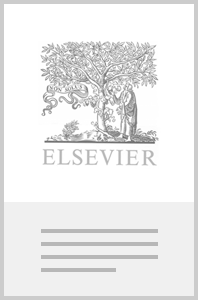
Purchase options
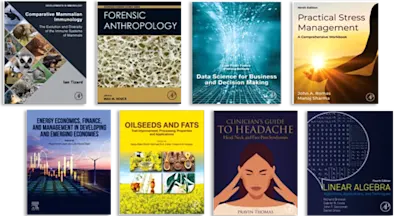
Institutional subscription on ScienceDirect
Request a sales quoteMachine Learning Solutions for Inverse Problems: Part A, Volume 26 in the Handbook of Numerical Analysis, highlights new advances in the field, with this new volume presenting interesting chapters on a variety of timely topics, including Data-Driven Approaches for Generalized Lasso Problems, Implicit Regularization of the Deep Inverse Prior via (Inertial) Gradient Flow, Generalized Hardness of Approximation, Hallucinations, and Trustworthiness in Machine Learning for Inverse Problems, Energy-Based Models for Inverse Imaging Problems, Regularization Theory of Stochastic Iterative Methods for Solving Inverse Problems, and more.
Other sections cover Advances in Identifying Differential Equations from Noisy Data Observations, The Complete Electrode Model for Electrical Impedance Tomography: A Comparative Study of Deep Learning and Analytical Methods, Learned Iterative Schemes: Neural Network Architectures for Operator Learning, Jacobian-Free Backpropagation for Unfolded Schemes with Convergence Guarantees, and Operator Learning Meets Inverse Problems: A Probabilistic Perspective
Other sections cover Advances in Identifying Differential Equations from Noisy Data Observations, The Complete Electrode Model for Electrical Impedance Tomography: A Comparative Study of Deep Learning and Analytical Methods, Learned Iterative Schemes: Neural Network Architectures for Operator Learning, Jacobian-Free Backpropagation for Unfolded Schemes with Convergence Guarantees, and Operator Learning Meets Inverse Problems: A Probabilistic Perspective
- Provides the authority and expertise of leading contributors from an international board of authors
- Presents the latest release in the Handbook of Numerical Analysis series
- Updated release includes the latest information on the Machine Learning Solutions for Inverse Problems
Mathematicians, Computational Scientists, Engineers, Computer Scientists, Academic Instructors and Educators, Machine Learning Researchers, Data Analysts, Scientific Programmers. Quantitative Analysts
1. Data-Driven Approaches for Generalized Lasso Problems
2. Implicit Regularization of the Deep Inverse Prior via (Inertial) Gradient Flow
3. Generalized Hardness of Approximation, Hallucinations, and Trustworthiness in Machine Learning for Inverse Problems
4. Energy-Based Models for Inverse Imaging Problems
5. Regularization Theory of Stochastic Iterative Methods for Solving Inverse Problems
6. Advances in Identifying Differential Equations from Noisy Data Observations
7. The Complete Electrode Model for Electrical Impedance Tomography: A Comparative Study of Deep Learning and Analytical Methods
8. Learned Iterative Schemes: Neural Network Architectures for Operator Learning
9. Jacobian-Free Backpropagation for Unfolded Schemes with Convergence Guarantees
10. Operator Learning Meets Inverse Problems: A Probabilistic Perspective
2. Implicit Regularization of the Deep Inverse Prior via (Inertial) Gradient Flow
3. Generalized Hardness of Approximation, Hallucinations, and Trustworthiness in Machine Learning for Inverse Problems
4. Energy-Based Models for Inverse Imaging Problems
5. Regularization Theory of Stochastic Iterative Methods for Solving Inverse Problems
6. Advances in Identifying Differential Equations from Noisy Data Observations
7. The Complete Electrode Model for Electrical Impedance Tomography: A Comparative Study of Deep Learning and Analytical Methods
8. Learned Iterative Schemes: Neural Network Architectures for Operator Learning
9. Jacobian-Free Backpropagation for Unfolded Schemes with Convergence Guarantees
10. Operator Learning Meets Inverse Problems: A Probabilistic Perspective
- Edition: 1
- Volume: 26
- Published: October 1, 2025
- Imprint: Academic Press
- Language: English
- Hardback ISBN: 9780443417894
- eBook ISBN: 9780443417900
AH
Andreas Hauptmann
Andreas Hauptmann received his PhD in 2017 from the University of Helsinki in Applied Mathematics. He currently holds a position as Academy Research Fellow and Associate Professor (tenure track) of Computational Mathematics at the Research Unit of Mathematical Sciences, University of Oulu, and as Honorary Associate Professor at the Department of Computer Science, University College London. His research interest is in inverse problems and tomographic imaging, with a focus on combining model-based inversion techniques with data-driven methods and the study of their theoretical properties.
Affiliations and expertise
Research Unit of Mathematical Sciences, University of Oulu, Oulu, Finland. Department of Computer Science, University College London, London, UKMH
Michael Hintermüller
Affiliations and expertise
Institut für Mathematik, Humboldt-Universität zu Berlin, Berlin, GermanyBJ
Bangti Jin
Bangti Jin received a PhD in Mathematics from The Chinese University
of Hong Kong, Hong Kong in 2008. Previously, he was Lecturer and Reader, and Professor at Department of Computer Science, University
College London (2014-2022), an assistant professor of Mathematics at the University of California, Riverside (2013–2014), a visiting assistant professor at Texas A&M University (2010–2013), an Alexandre von Humboldt Postdoctoral Researcher at University of Bremen (2009–2010). Currently he is Professor of Mathematics, Global STEM Scholar, at The Chinese University of Hong Kong. His research interests include inverse problems, numerical analysis and machine learning.
Affiliations and expertise
Department of Mathematics, The Chinese University of Hong Kong, Shatin, N.T., Hong KongCS
Carola-Bibiane Schönlieb
Carola-Bibiane Schönlieb graduated from the Institute for Mathematics, University of Salzburg (Austria) in 2004. From 2004 to 2005 she held a teaching position in Salzburg. She received her PhD degree from the University of Cambridge (UK) in 2009. After one year of postdoctoral activity at the University of Göttingen (Germany), she became a Lecturer at Cambridge in 2010, promoted to Reader in 2015 and promoted to Professor in 2018. Since 2011 she is a fellow of Jesus College Cambridge. She currently is Professor of Applied Mathematics (2006) at the University of Cambridge where she is head of the Cambridge Image Analysis group. Her current research interests focus on variational methods, partial differential equations and machine learning for image analysis, image processing and inverse imaging problems.
Affiliations and expertise
Department of Applied Mathematics and Theoretical Physics, University of Cambridge, UK