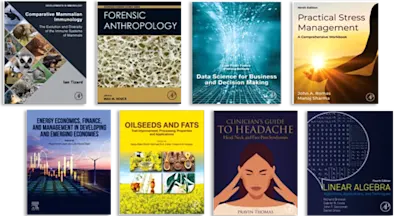
Machine Learning Proceedings 1992
Proceedings of the Ninth International Workshop (ML92)
- 1st Edition - June 1, 1992
- Imprint: Morgan Kaufmann
- Editors: Peter Edwards, Derek Sleeman
- Language: English
- Paperback ISBN:9 7 8 - 1 - 5 5 8 6 0 - 2 4 7 - 2
- eBook ISBN:9 7 8 - 1 - 4 8 3 2 - 9 8 5 3 - 5
Machine Learning: Proceedings of the Ninth International Workshop (ML92) covers the papers and posters presented at ML92, the Ninth International Machine Learning Conference, held… Read more
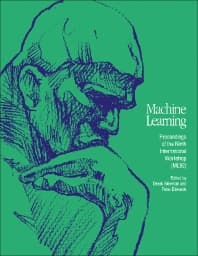
Purchase options
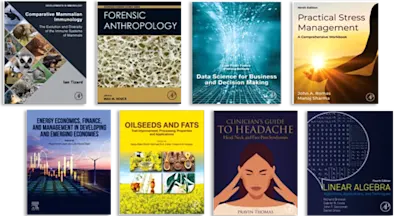
Institutional subscription on ScienceDirect
Request a sales quoteMachine Learning: Proceedings of the Ninth International Workshop (ML92) covers the papers and posters presented at ML92, the Ninth International Machine Learning Conference, held at Aberdeen, Scotland on July 1-3, 1992. The book focuses on the advancements of practices, methodologies, approaches, and techniques in machine learning. The selection first offers information on the principal axes method for constructive induction; learning by incomplete explanations of failures in recursive domains; and eliminating redundancy in explanation-based learning. Topics include means-ends analysis search in recursive domains, description space transformation, distance metric, generating similarity matrix, and learning principal axes. The text then examines trading off consistency and efficiency in version-space induction; improving path planning with learning; finding the conservation of momentum; and learning to predict in uncertain continuous tasks. The manuscript elaborates on a teaching method for reinforcement learning, compiling prior knowledge into an explicit bias, spatial analogy and subsumption, and multistrategy learning with introspective meta-explanations. The publication also ponders on selecting typical instances in instance-based learning and temporal difference learning of backgammon strategy. The selection is a valuable source of information for researchers interested in machine learning.
Generalizing from Case Studies: A Case Study
On Learning More Concepts
The Principal Axes Method for Constructive Induction
Learning by Incomplete Explanations of Failures in Recursive Domains
Eliminating Redundancy in Explanation-Based Learning
Trading Off Consistency and Efficiency in Version-Space Induction
Peepholing: Choosing Attributes Efficiently for Megainduction
Improving Path Planning with Learning
The Right Representation for Discovery: Finding the Conservation of Momentum
Learning to Predict in Uncertain Continuous Tasks
Lazy Partial Evaluation: An Integration of Explanation-Based Generalisation and Partial Evaluation
A Teaching Method for Reinforcement Learning
Compiling Prior Knowledge into an Explicit Bias
Spatial Analogy and Subsumption
Learning to Satisfy Conjunctive Goals
Multistrategy Learning with Introspective Meta-Explanations
An Asymptotic Analysis of Speedup Learning
Why EBL Produces Overly-Specific Knowledge: A Critique of the PRODIGY Approaches
Automatic Feature Generation for Problem Solving Systems
Towards Inductive Generalisation in Higher Order Logic
Ordering Effects in Clustering
Learning Structured Concepts Using Genetic Algorithms
An Analysis of Learning to Plan as a Search Problem
An Approach to Anytime Learning
Artificial Universes—Towards a Systematic Approach to Evaluating Algorithms Which Learn from Examples
Average Case Analysis of Learning k-CNF Concepts
The MENTLE Approach to Learning Heuristics for the Control of Logic Programs
Fuzzy Substructure Discovery
Efficient Classification of Massive, Unsegmented Datastreams
Induction of One-Level Decision Trees
Combining Competition and Cooperation in Supervised Inductive Learning
A Practical Approach to Feature Selection
Learning as Optimization: Stochastic Generation of Multiple Knowledge
Dynamic Optimization
Sub-Unification: A Tool for Efficient Induction of Recursive Programs
Augmenting and Efficiently Utilizing Domain Theory in Explanation-Based Natural Language Acquisition
Enhancing Transfer in Reinforcement Learning by Building Stochastic Models of Robot Actions
THOUGHT: An Integrated Learning System for Acquiring Knowledge Structure
An Approach to Concept Learning Based on Term Generalization
Using Transitional Proximity for Faster Reinforcement Learning
NFDT: A System that Learns Flexible Concepts Based on Decision Trees for Numerical Attributes
A Symbolic Algorithm for Computing Coefficients' Accuracy in Regression
Compression, Significance, and Accuracy
Guiding Example Acquisition by Generating Scenarios
Constructive Induction Using a Non-Greedy Strategy for Feature Selection
Training Second-Order Recurrent Neural Networks Using Hints
DYNAMIC: A New Role for Training Problems in EBL
A Framework for Discovering Discrete Event Models
Learning Episodes for Optimization
Learning to Fly
Deconstructing the Digit Recognition Problem
On Combining Multiple Speedup Techniques
Scaling Reinforcement Learning Algorithms by Learning Variable Temporal Resolution Models
Detecting Novel Classes with Applications to Fault Diagnosis
Measuring Utility and the Design of Provably Good EBL Algorithms
Refining a Relational Theory with Multiple Faults in the Concept and Subconcepts
Cooperation in Knowledge Base Refinement
Temporal Difference Learning of Backgammon Strategy
AGIL: Solving the Exploration Versus Exploitation Dilemma in a Simple Classifier System Applied to Simulated Robotics
Conceptual Clustering with Systematic Missing Values
Selecting Typical Instances in Instance-Based Learning
The First Phase of Real-World Discovery: Determining Repeatability and Error of Experiments
Author Index
Subject Index
- Edition: 1
- Published: June 1, 1992
- No. of pages (eBook): 448
- Imprint: Morgan Kaufmann
- Language: English
- Paperback ISBN: 9781558602472
- eBook ISBN: 9781483298535
PE
Peter Edwards
Affiliations and expertise
University of GlamorganRead Machine Learning Proceedings 1992 on ScienceDirect